Physics-Informed Approximation of Internal Thermal History for Surface Deformation Predictions in Wire Arc Directed Energy Deposition
Journal of Manufacturing Science and Engineering(2024)
Abstract
Abstract This work presents a physics-informed fusion methodology for deformation detection using multi-sensor thermal data. A challenge with additive manufacturing (AM) is that abnormalities commonly occur due to rapid changes in the thermal gradient. Different non-destructive in-situ thermal sensors capture parts of the thermal history but are limited by the visible temperature spectrum and sensor field of view of the fabrication process. Various sensors mitigate problems with the loss of thermal history information; however, it brings forth challenges with integrating different data streams and the need to interpolate the internal thermal history. This study develops a thermal data-informed heat flux methodology that fills the gap in fusing numerical temperature approximation with data-driven knowledge of the surface of additive manufactured (AM) components. First, this study fuses infrared (IR) thermal data complexities during the AM process with the Goldak double ellipsoidal heat flux to model the energy input into the component. Second, a thermal physics-informed model input (PIMI) is created with thermal data-informed heat flux to capture internal thermal history. Lastly, regression convolutional neural network (CNN) captures the relationship between the three-dimensional thermal gradient and surface deformation. The rapid thermal gradient formation and identification of deformation is a key step toward using thermal history data and machine learning to improve quality control in AM. The proposed surface deformation detection model achieved an MSE of 1.14 mm and an R2 of 0.89.
MoreTranslated text
AI Read Science
Must-Reading Tree
Example
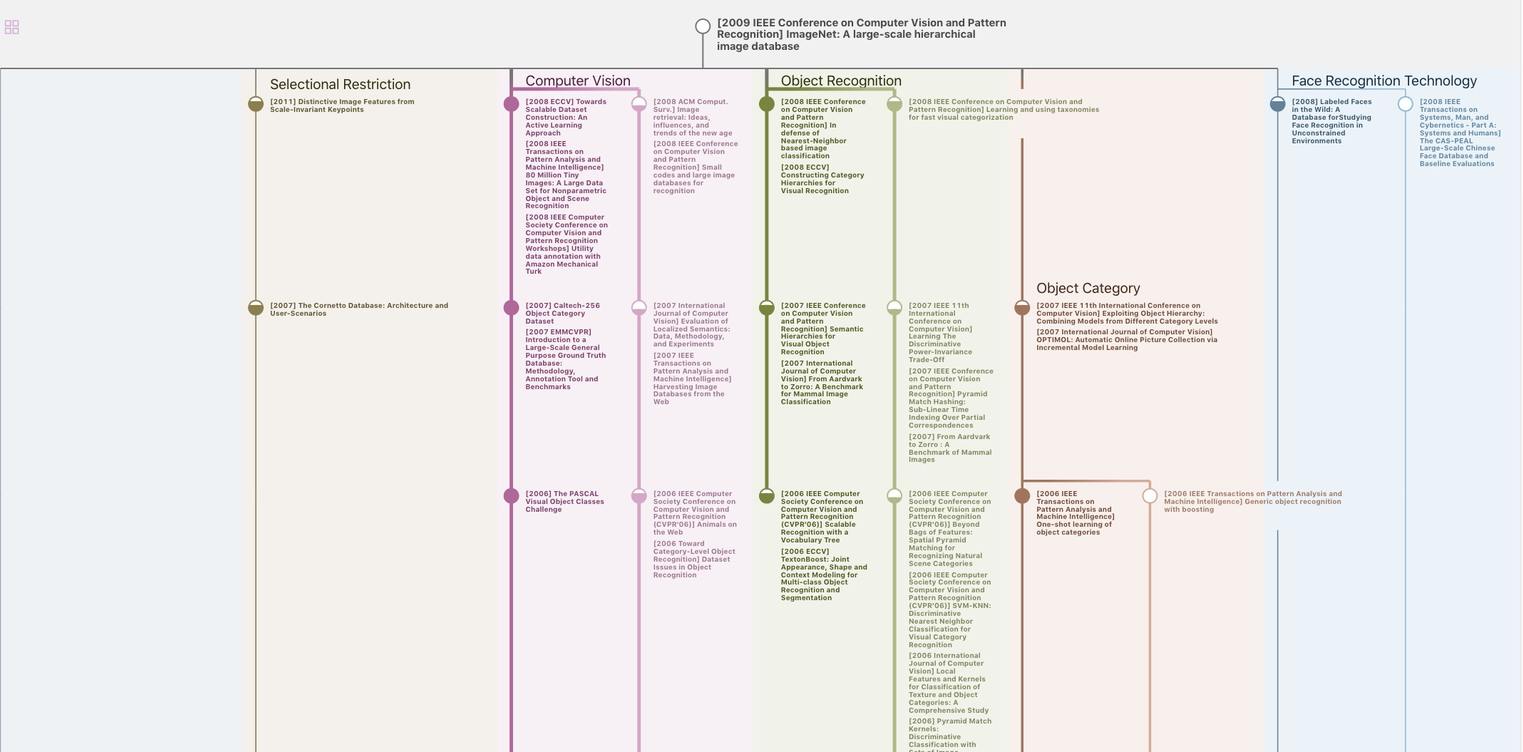
Generate MRT to find the research sequence of this paper
Chat Paper
Summary is being generated by the instructions you defined