Convolutional Neural Network for Emotional EEG Decoding and Visualization.
International Conferences on Computing and Pattern Recognition(2023)
Abstract
In recent years, deep learning has been increasingly utilized in affective Brain-Computer Interface (aBCI) research. The application of Convolutional Neural Networks (ConvNets) for end-to-end analysis of electroencephalographic (EEG) signals has become a common approach in deep learning-based aBCI. However, limited research has been conducted on a better understanding of how to design and train ConvNets for end-to-end emotional EEG decoding. This study explores three kinds of ConvNets architectures, including shallow, middle, and deep configuration, to evaluate their design and training schemes. The findings of this paper demonstrate that, for aBCI, it is crucial to ensure an adequate sample size for model training while maintaining the stability of EEG signals. Additionally, achieving a balance between sample length and size is crucial for effective model training. Notably, EEGNet outperforms the other two models in terms of classification accuracy, indicating that an excessively shallow number of convolutional layers leads to insufficient feature extraction, while an excessively deep number of convolutional layers increases the risk of overfitting.
MoreTranslated text
AI Read Science
Must-Reading Tree
Example
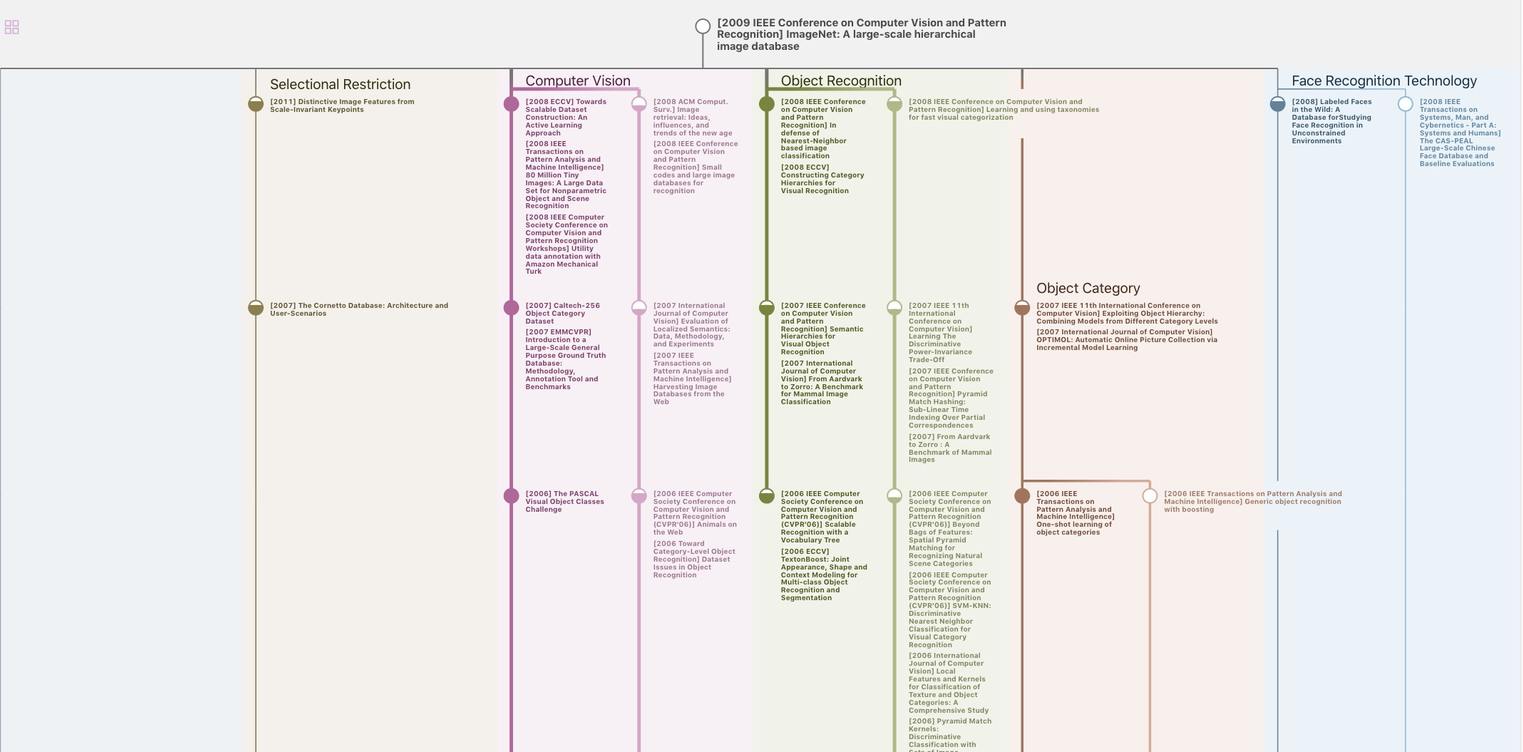
Generate MRT to find the research sequence of this paper
Chat Paper
Summary is being generated by the instructions you defined