Bayesian Federated Inference for Survival Models
arxiv(2024)
摘要
In cancer research, overall survival and progression free survival are often
analyzed with the Cox model. To estimate accurately the parameters in the
model, sufficient data and, more importantly, sufficient events need to be
observed. In practice, this is often a problem. Merging data sets from
different medical centers may help, but this is not always possible due to
strict privacy legislation and logistic difficulties. Recently, the Bayesian
Federated Inference (BFI) strategy for generalized linear models was proposed.
With this strategy the statistical analyses are performed in the local centers
where the data were collected (or stored) and only the inference results are
combined to a single estimated model; merging data is not necessary. The BFI
methodology aims to compute from the separate inference results in the local
centers what would have been obtained if the analysis had been based on the
merged data sets. In this paper we generalize the BFI methodology as initially
developed for generalized linear models to survival models. Simulation studies
and real data analyses show excellent performance; i.e., the results obtained
with the BFI methodology are very similar to the results obtained by analyzing
the merged data. An R package for doing the analyses is available.
更多查看译文
AI 理解论文
溯源树
样例
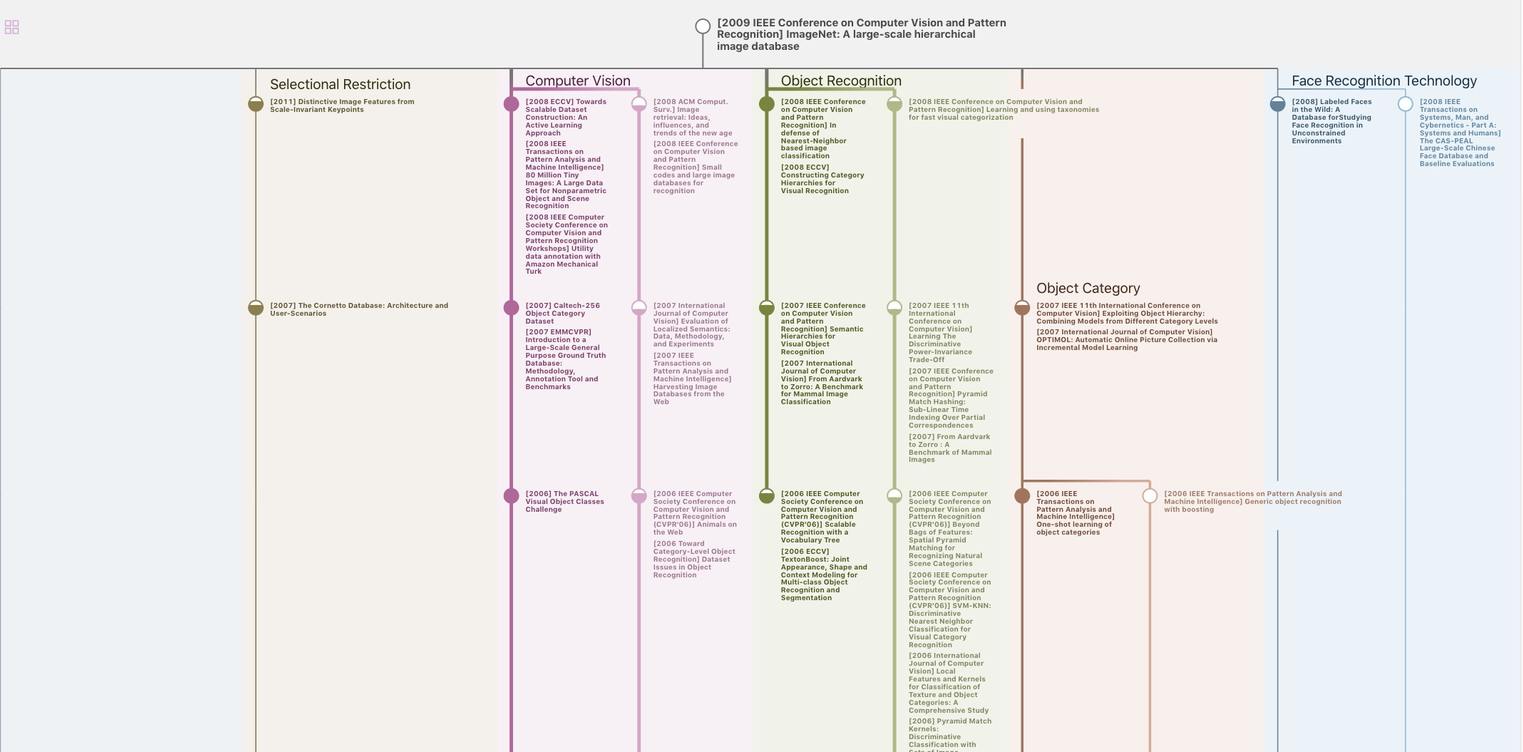
生成溯源树,研究论文发展脉络
Chat Paper
正在生成论文摘要