Adversarial Consistency and the Uniqueness of the Adversarial Bayes Classifier
CoRR(2024)
Abstract
Adversarial training is a common technique for learning robust classifiers.
Prior work showed that convex surrogate losses are not statistically consistent
in the adversarial context – or in other words, a minimizing sequence of the
adversarial surrogate risk will not necessarily minimize the adversarial
classification error. We connect the consistency of adversarial surrogate
losses to properties of minimizers to the adversarial classification risk,
known as adversarial Bayes classifiers. Specifically, under reasonable
distributional assumptions, a convex loss is statistically consistent for
adversarial learning iff the adversarial Bayes classifier satisfies a certain
notion of uniqueness.
MoreTranslated text
AI Read Science
Must-Reading Tree
Example
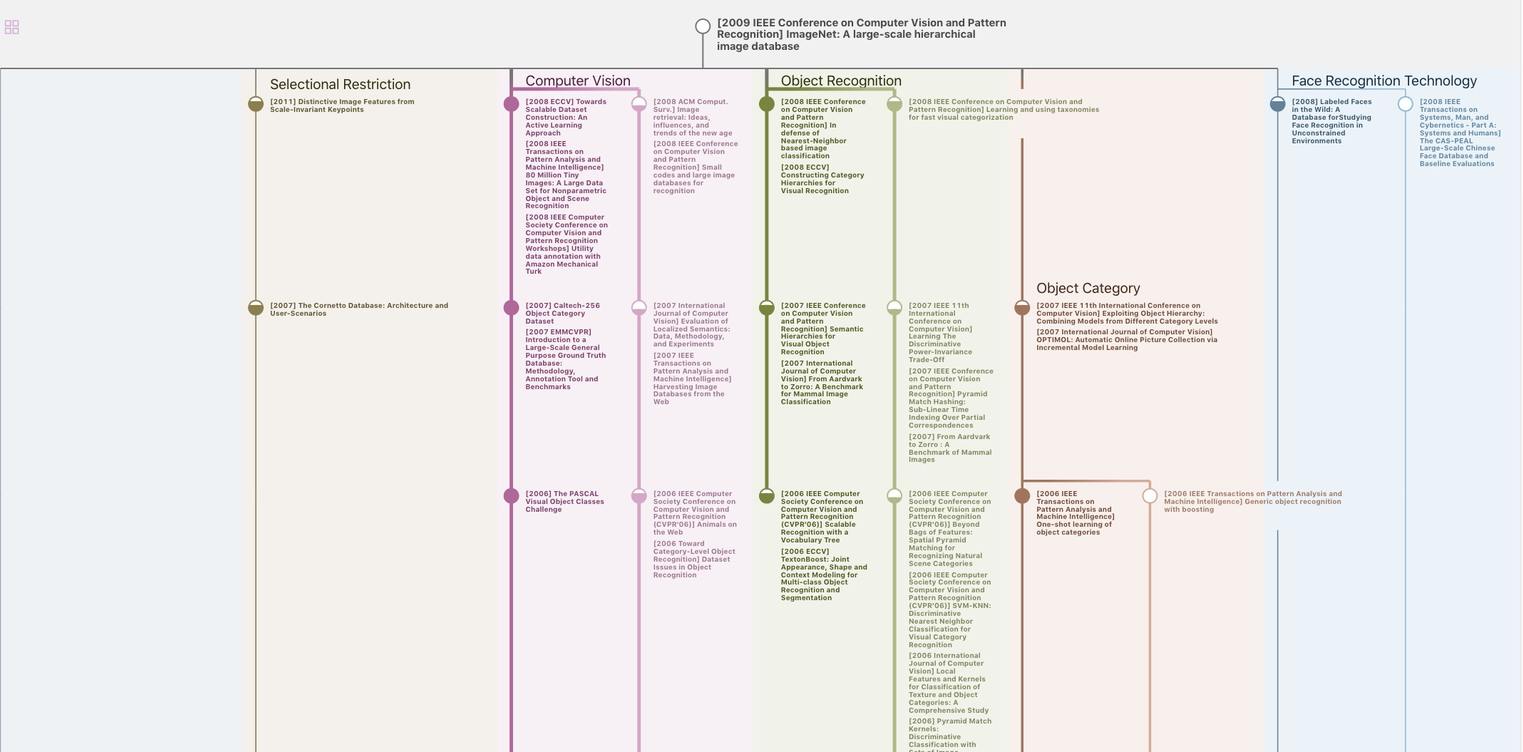
Generate MRT to find the research sequence of this paper
Chat Paper
Summary is being generated by the instructions you defined