Optimal filtering strategies for task-specific functional PET imaging
crossref(2024)
摘要
Functional Positron Emission Tomography (fPET) has advanced as an effective tool for investigating dynamic processes in glucose metabolism and neurotransmitter action, offering potential insights into brain function, disease progression, and treatment development. Despite significant methodological advances, extracting stimulation-specific information presents additional challenges in optimizing signal processing across both spatial and temporal domains, which are essential for obtaining clinically relevant insights. This study aims to provide a systematic evaluation of state-of-the-art filtering techniques for fPET imaging. Forty healthy participants underwent a single [18F]FDG PET/MR scan, engaging in the cognitive task Tetris®. Twenty thereof also underwent a second PET/MR session. Eight filtering techniques, including 3D and 4D Gaussian smoothing, highly constrained backprojection (hypr), iterative hypr (Ihypr4D), two MRI-Markov Random Field (MRI-MRF) filters (L=10 and 14 mm neighborhood) as well as static and dynamic Non-Local Means (sNLM and dNLM respectively) approaches, were applied to fPET data. Test-retest reliability (intraclass correlation coefficient), the identifiability of the task signal (temporal signal-to-noise ratio (tSNR)), spatial task-based activation (group level t-values), and sample size calculations were assessed. Results indicate distinct performance between filtering techniques. Compared to standard 3D Gaussian smoothing, dNLM, sNLM, MRI-MRF L=10 and Ihypr4D filters exhibited superior tSNR, while only dNLM and hypr showed improved test-retest reliability. Spatial task-based activation was enhanced by both NLM filters and MRI-MRF approaches. The dNLM enabled a minimum reduction of 15.4% in required sample size. The study systematically evaluated filtering techniques in fPET data processing, highlighting their strengths and limitations. The dNLM filter emerges as a promising choice, with improved performance across all metrics. However, filter selection should align with specific study objectives, considering factors like processing time and resource constraints.
更多查看译文
AI 理解论文
溯源树
样例
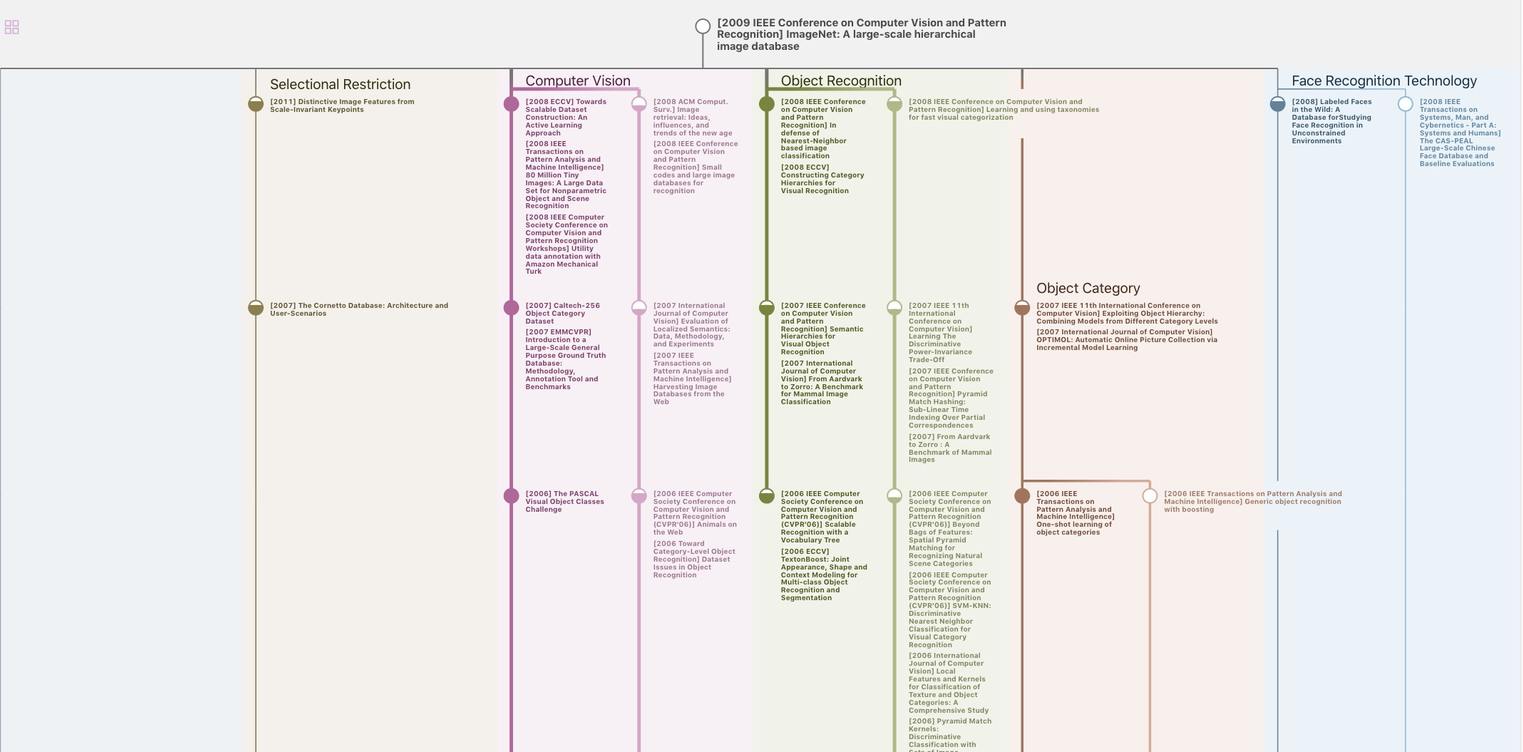
生成溯源树,研究论文发展脉络
Chat Paper
正在生成论文摘要