Application of machine learning for high-throughput tumor marker screening
Life Sciences(2024)
Abstract
High-throughput sequencing and multiomics technologies have allowed increasing numbers of biomarkers to be mined and used for disease diagnosis, risk stratification, efficacy assessment, and prognosis prediction. However, the large number and complexity of tumor markers make screening them a substantial challenge. Machine learning (ML) offers new and effective ways to solve the screening problem. ML goes beyond mere data processing and is instrumental in recognizing intricate patterns within data. ML also has a crucial role in modeling dynamic changes associated with diseases. Used together, ML techniques have been included in automatic pipelines for tumor marker screening, thereby enhancing the efficiency and accuracy of the screening process. In this review, we discuss the general processes and common ML algorithms, and highlight recent applications of ML in tumor marker screening of genomic, transcriptomic, proteomic, and metabolomic data of patients with various types of cancers. Finally, the challenges and future prospects of the application of ML in tumor therapy are discussed.
MoreTranslated text
Key words
Tumor markers,Machine learning,Screening
AI Read Science
Must-Reading Tree
Example
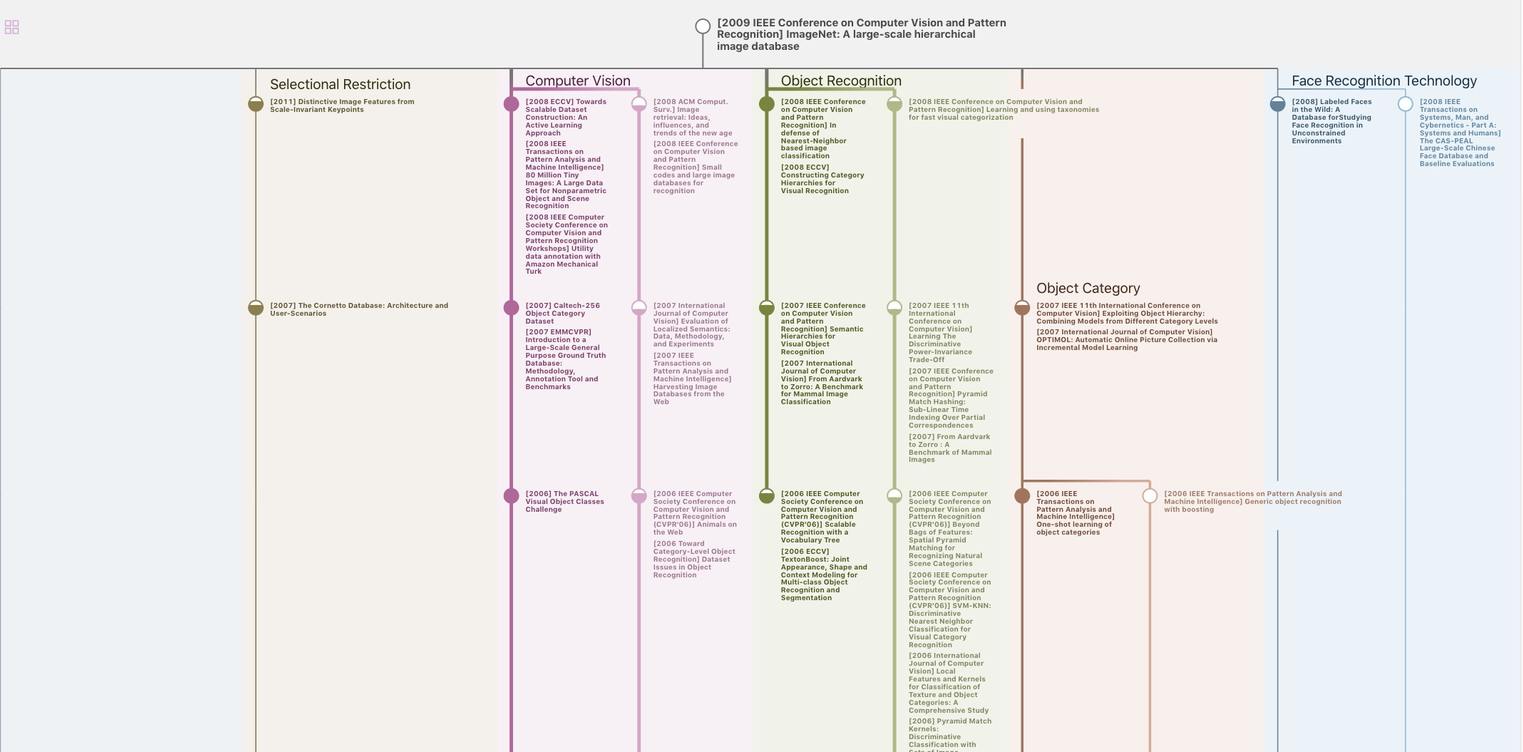
Generate MRT to find the research sequence of this paper
Chat Paper
Summary is being generated by the instructions you defined