On the factor ambiguity of MCR problems for blockwise incomplete data sets
Chemometrics and Intelligent Laboratory Systems(2024)
Abstract
Multivariate curve resolution (MCR) methods are sometimes faced with missing or erroneous data, e.g., due to sensor saturation. In some cases, an estimation of the missing data is possible, but often MCR works with the largest submatrix without missing entries. This ignores all rows and columns of the data matrix that contain missing values. A successful approach to deal with incomplete data multisets has been proposed by Alier and Tauler (2013), but it does not include a factor ambiguity analysis. Here, the missing data problem is addressed in combination with a factor ambiguity analysis. An approach is presented that minimizes the factor ambiguity by extracting a maximum of spectral information even from incomplete rows and columns of the spectral data matrix. The method requires a high signal-to-noise ratio. Applications are presented for UV/Vis and HSI data.
MoreTranslated text
Key words
Multivariate curve resolution,Area of feasible solutions,Incomplete data,Missing data
AI Read Science
Must-Reading Tree
Example
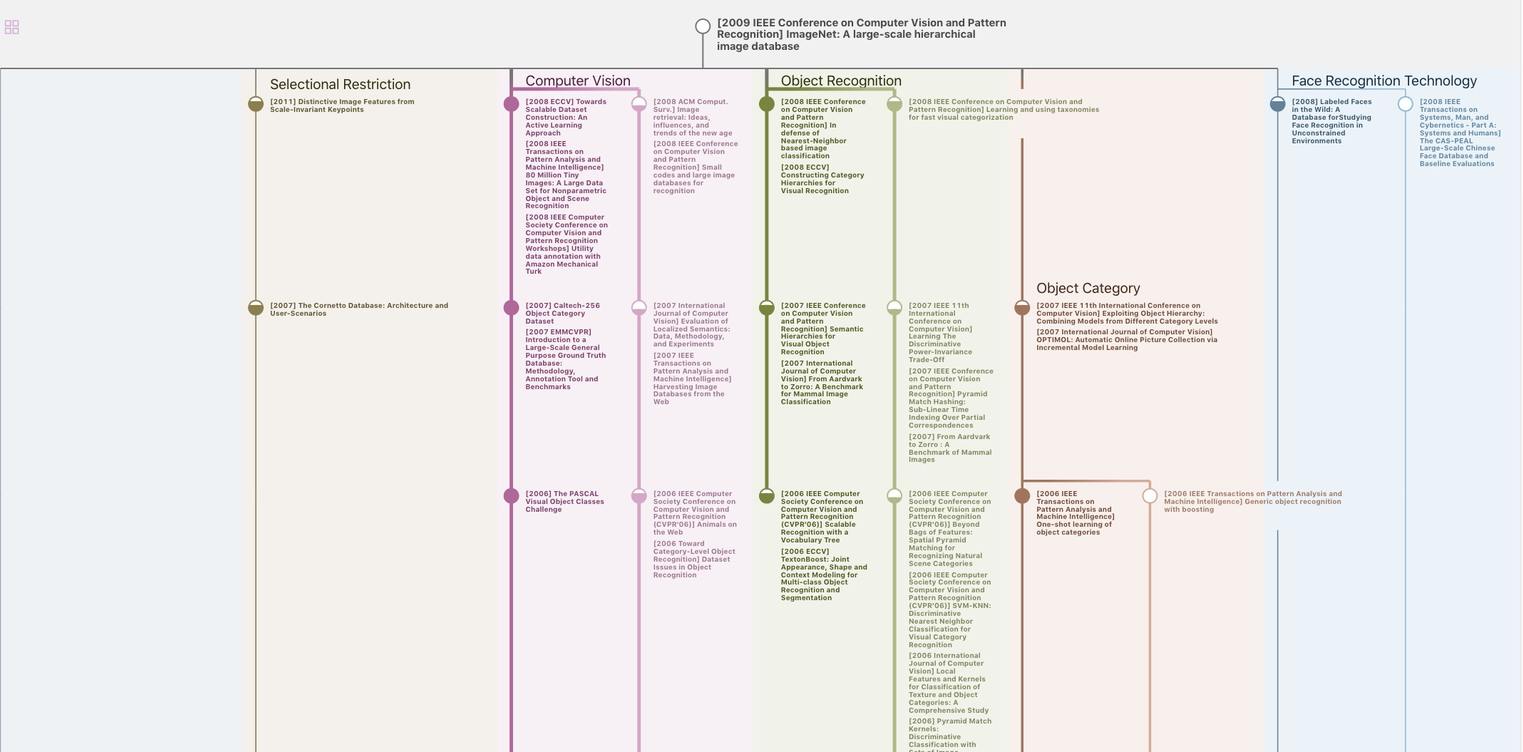
Generate MRT to find the research sequence of this paper
Chat Paper
Summary is being generated by the instructions you defined