Simplifying Bayesian analysis of graphical models for the social sciences with easybgm: A user-friendly R-package
crossref(2024)
Abstract
Network psychometrics models psychological constructs as interconnected variables. Rather than treating variables as independent entities, network analysis views them as nodes in a system that interact with each other; their interactions yield partial associations. Recently, researchers have emphasized the use of Bayesian methods in graphical modeling to accurately quantify uncertainty in the model and its parameters. Several R packages have been developed that implement different Bayesian estimation approaches for graphical modeling in R. However, they all require different inputs and produce different outputs, making them difficult to use for applied researchers. In this paper, we present a user-friendly R package called easybgm that combines the powerful analysis tools into a cohesive package for applied re-searchers. The package allows researchers to fit any type of cross-sectional data, extract results, and visualize findings with network, edge evidence, and structure uncertainty plots. We introduce the package and demonstrate its use with two examples.
MoreTranslated text
AI Read Science
Must-Reading Tree
Example
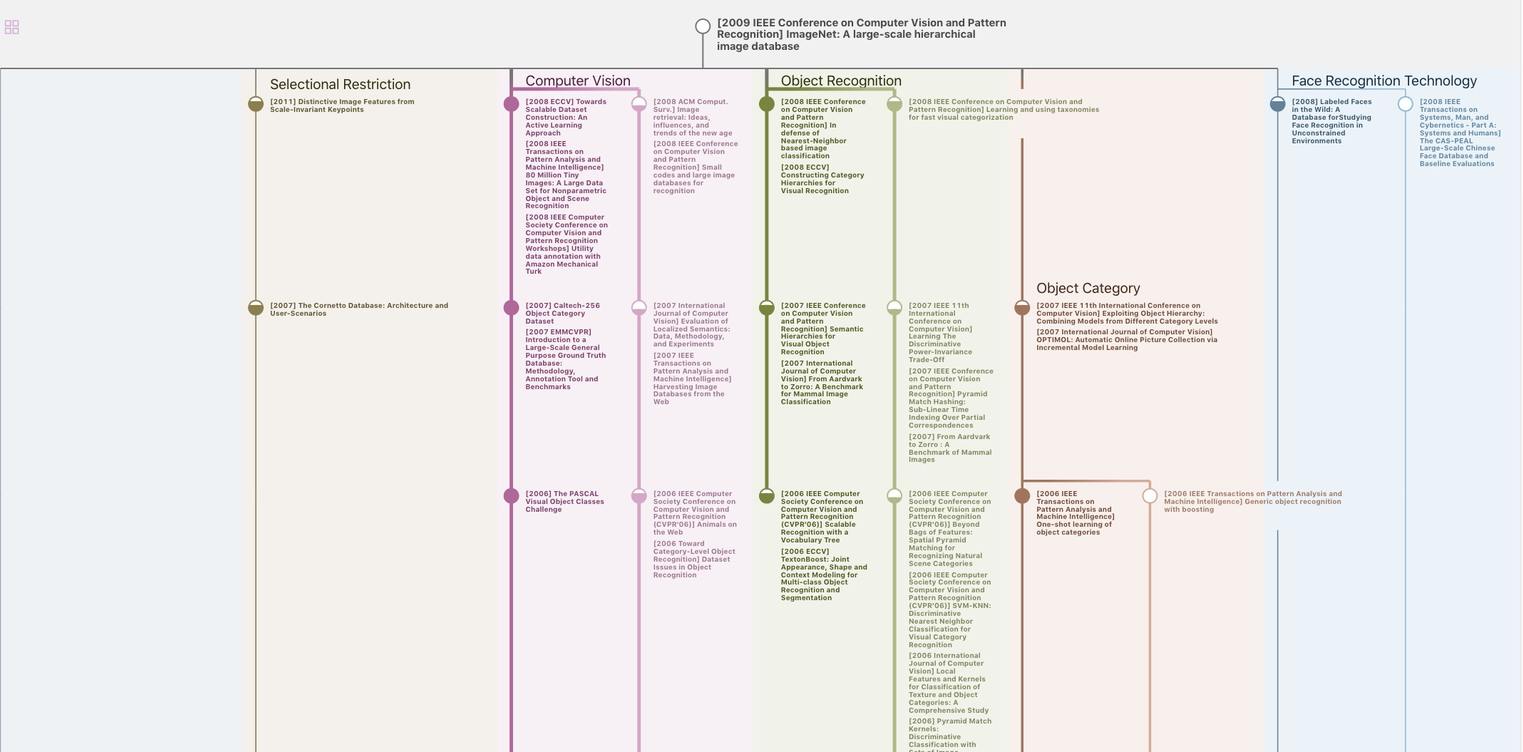
Generate MRT to find the research sequence of this paper
Chat Paper
Summary is being generated by the instructions you defined