Multi-bolt looseness positioning using multivariate recurrence analytic active sensing method and MHAMCNN model
STRUCTURAL HEALTH MONITORING-AN INTERNATIONAL JOURNAL(2024)
摘要
The piezoelectric active sensing method has been proven to be an effective, nondestructive approach for bolt looseness monitoring. The conventional damage index relies on the energy index of the wavelet packet. However, the method of wavelet packet could not identify the looseness location of the multi-bolt. Therefore, an innovative multi-bolt intelligent method combined the multivariate recurrence analytic active sensing method with an improved convolutional neural network (CNN) model was proposed for multi-bolt looseness positioning. In this method, the multivariate recurrence plots (RPs) are firstly constructed based on the received stress waves and then it is utilized to extract the recursive attributes presented within the stress wave signals. Secondly, a novel model, the CNN improved by the multi-head attention mechanism (MHAMCNN), is constructed to extract nonlinear features hidden in multivariate RPs. Repeated experiments demonstrate that the proposed method, which combines MHAMCNN and un-thresholded multivariate RPs (i.e., mean detection accuracy: 98.62%), outperformed other methods, including multivariate RPs (i.e., mean detection accuracy: 97.06%) and common RPs. Compared with traditional pattern recognition algorithms, MHAMCNN has higher classification accuracy. In summary, the suggested approach presents a promising opportunity for the real-time monitoring of multi-bolt looseness.
更多查看译文
关键词
Multi-bolt looseness,piezoelectric active sensing method,multivariate recurrence analysis method,MHAMCNN model,structural health monitoring
AI 理解论文
溯源树
样例
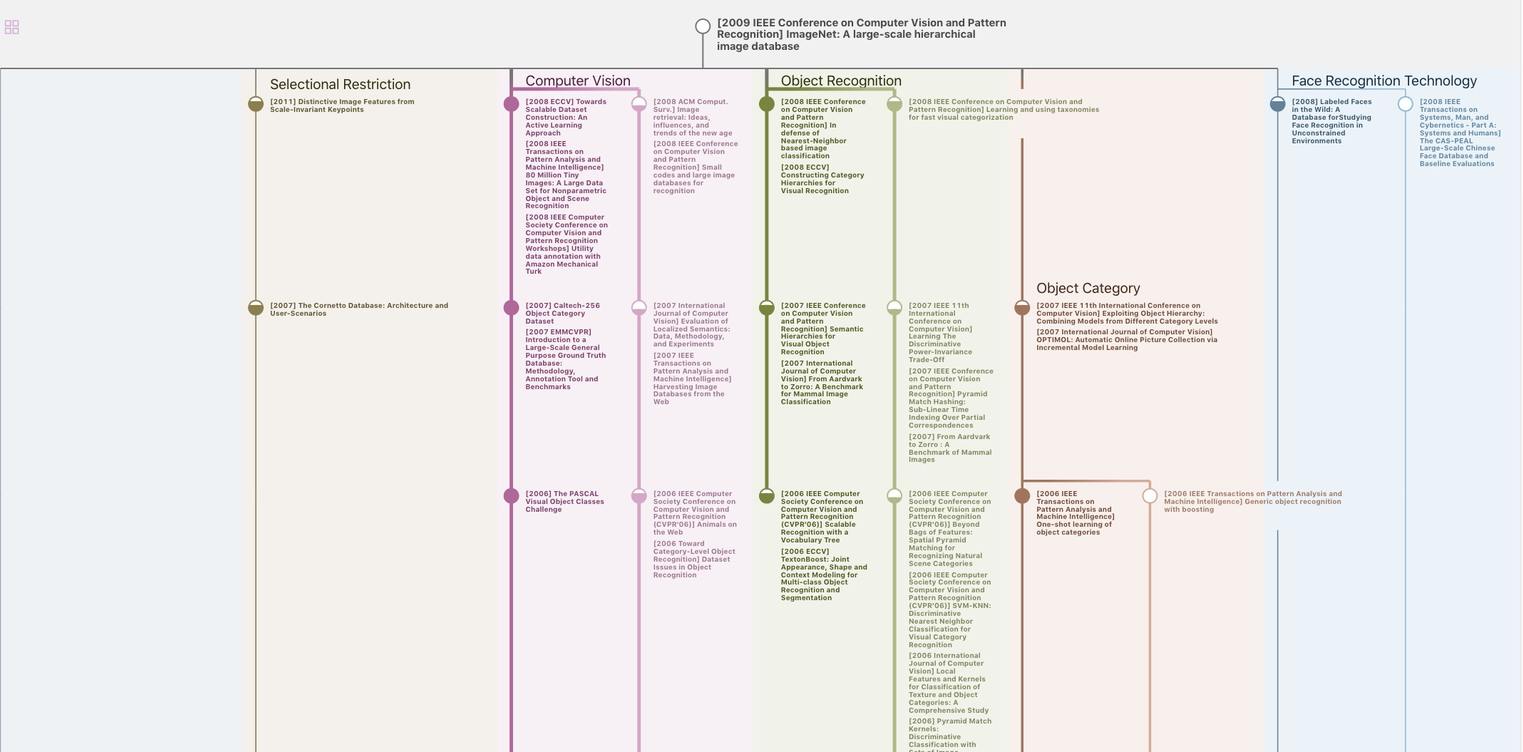
生成溯源树,研究论文发展脉络
Chat Paper
正在生成论文摘要