PROMISE: A pre-trained knowledge-infused multimodal representation learning framework for medication recommendation
Information Processing & Management(2024)
摘要
Electronic Health Records (EHRs) significantly enhance clinical decision-making, particularly in safe and effective medication recommendation based on complex patient data. Current methods, while encoding each medical event individually with domain-specific knowledge, inadequately harness multi-source domain knowledge and neglect the interrelations among various medical codes, the influence of historical patient visits, and the relevance of similar patient trajectories. To address these limitations, we present PROMISE, a multimodal medication recommendation framework that progressively learns patient representations from specific health states to a comprehensive view. PROMISE integrates domain knowledge into modality-specific encoders to improve local and global patient representations, facilitating enhanced medication recommendations through the interaction of patient representations from various modalities. Specifically, within the code modality, PROMISE utilizes encoding of EHR hypergraphs to learn patient representations featuring structured information. Simultaneously, in the text modality, it acquires patient representations with semantic information by encoding clinical texts obtained from tables. Our framework surpasses state-of-the-art baselines with up to 2.06% and 1.97% improvements on key metrics within the MIMIC-III and IV datasets, respectively, confirming its effectiveness and superiority.
更多查看译文
关键词
Multimodal learning,Healthcare,Data mining,Medication recommendation
AI 理解论文
溯源树
样例
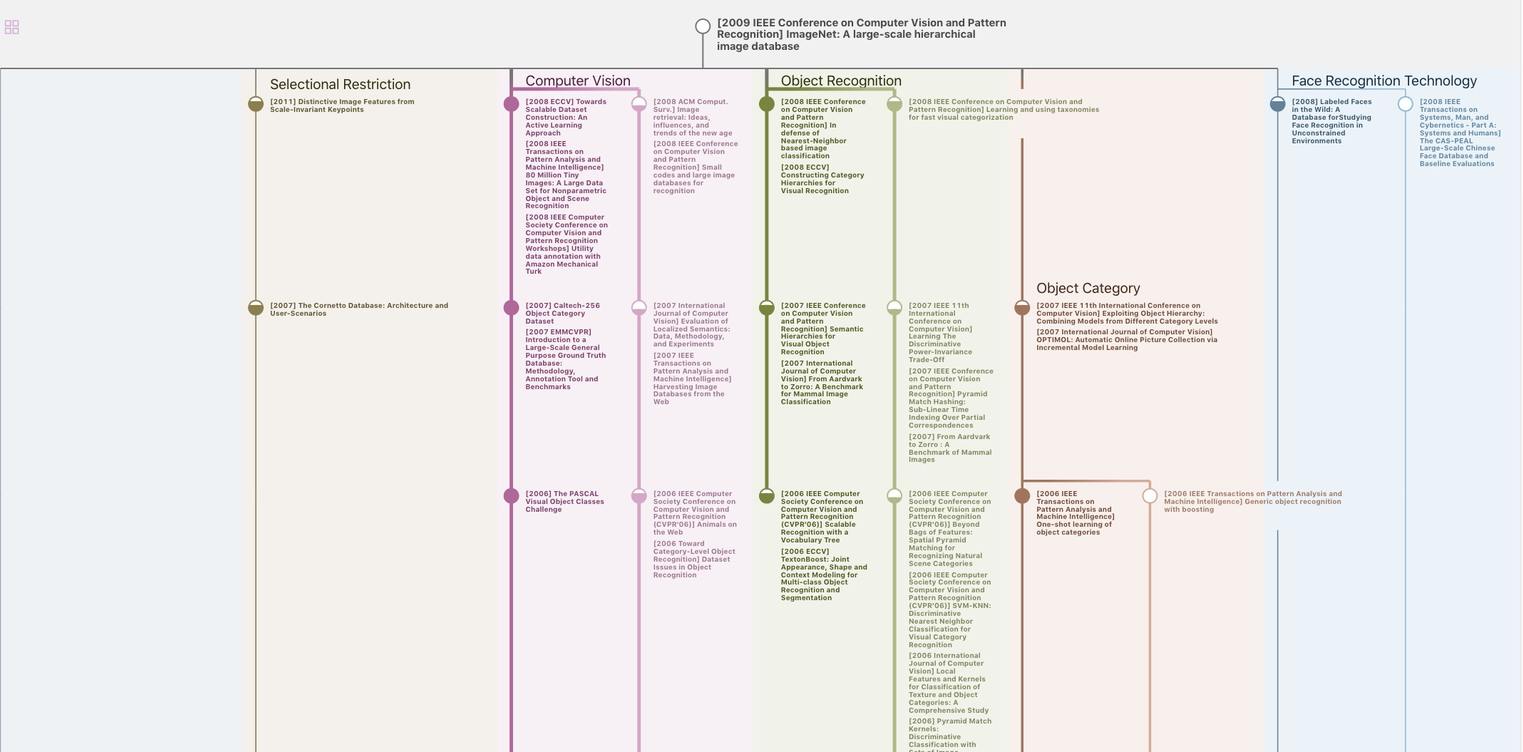
生成溯源树,研究论文发展脉络
Chat Paper
正在生成论文摘要