MIB-Net: Balance the mutual information flow in deep learning network for multi-dimensional segmentation of COVID-19 CT images
Biomedical Signal Processing and Control(2024)
Abstract
Computed tomography (CT) based lung screening followed by computer-vision-based automated segmentation has great potential in early diagnosis of COVID-19, but low sample size and high patient-to-patient variability of infection characteristics are two of the major challenges. We propose a novel Mutual Information Balanced Net (MIB-Net) with three distinctive features. First, it captures mutual information across feature maps, CT scans, and predicted segmentation labels to optimize the contribution of different feature maps for better segmentation accuracy. Second, it incorporates a three-dimensional structural prior of CT scans based on predicted segmentation of neighboring CT slices to improve accuracy. Third, a novel Weight Optimization Filter (WOF) is used to adjust the proportion of 3D structural prior to be incorporated, which further improves the segmentation accuracy. Experimental results show that the proposed approach outperforms state-of-the-art biomedical image segmentation models by 7.87%, 3.85%, and 5.01% in Dice Score, respectively for three popular COVID-19 CT datasets. The better performance of the proposed approach suggests that it may serve as an attractive alternative to Lung CT-based screening for COVID-19.
MoreTranslated text
Key words
Computed tomography,Biomedical image segmentation,Mutual information,Information theory,COVID-19
AI Read Science
Must-Reading Tree
Example
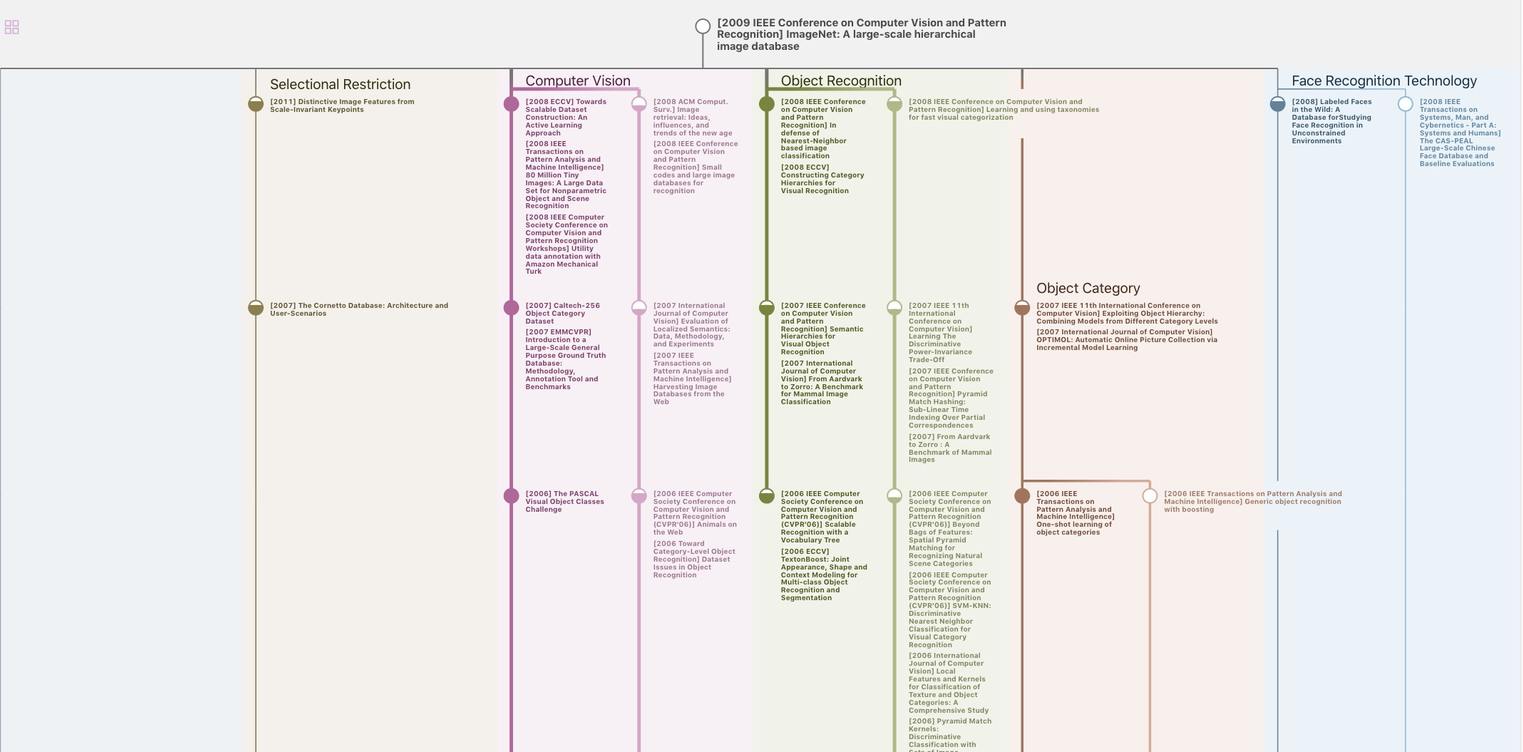
Generate MRT to find the research sequence of this paper
Chat Paper
Summary is being generated by the instructions you defined