A Differentially Privacy Assisted Federated Learning Scheme to Preserve Data Privacy for IoMT Applications
IEEE Transactions on Network and Service Management(2024)
摘要
The rapid development of Artificial Intelligence (AI) has had a significant impact on various industries, including healthcare. The Internet of Medical Things (IoMT) has played a vital role in this evolution. However, while AI has contributed to many benefits in healthcare, concerns about data privacy and security persist. To address these concerns, we propose a framework that combines Federated Learning (FL) and Differential Privacy (DP) to enhance data protection within IoMT. By integrating FL’s decentralized approach with DP’s mechanism to prevent data reconstruction from model outputs, we can improve data confidentiality. This integrated approach is used to develop and analyze high-performing Convolutional Neural Networks (CNNs) for detecting Tuberculosis using chest X-ray datasets. The framework undergo thorough performance evaluation, utilizing various metrics to establish its superiority over baseline models. The results demonstrate the effectiveness of our framework as a robust solution for secure and private AI applications in healthcare.
更多查看译文
关键词
Computer Vision,Differential Privacy,Ensemble Learning,Federated Learning,Internet of Medical Things
AI 理解论文
溯源树
样例
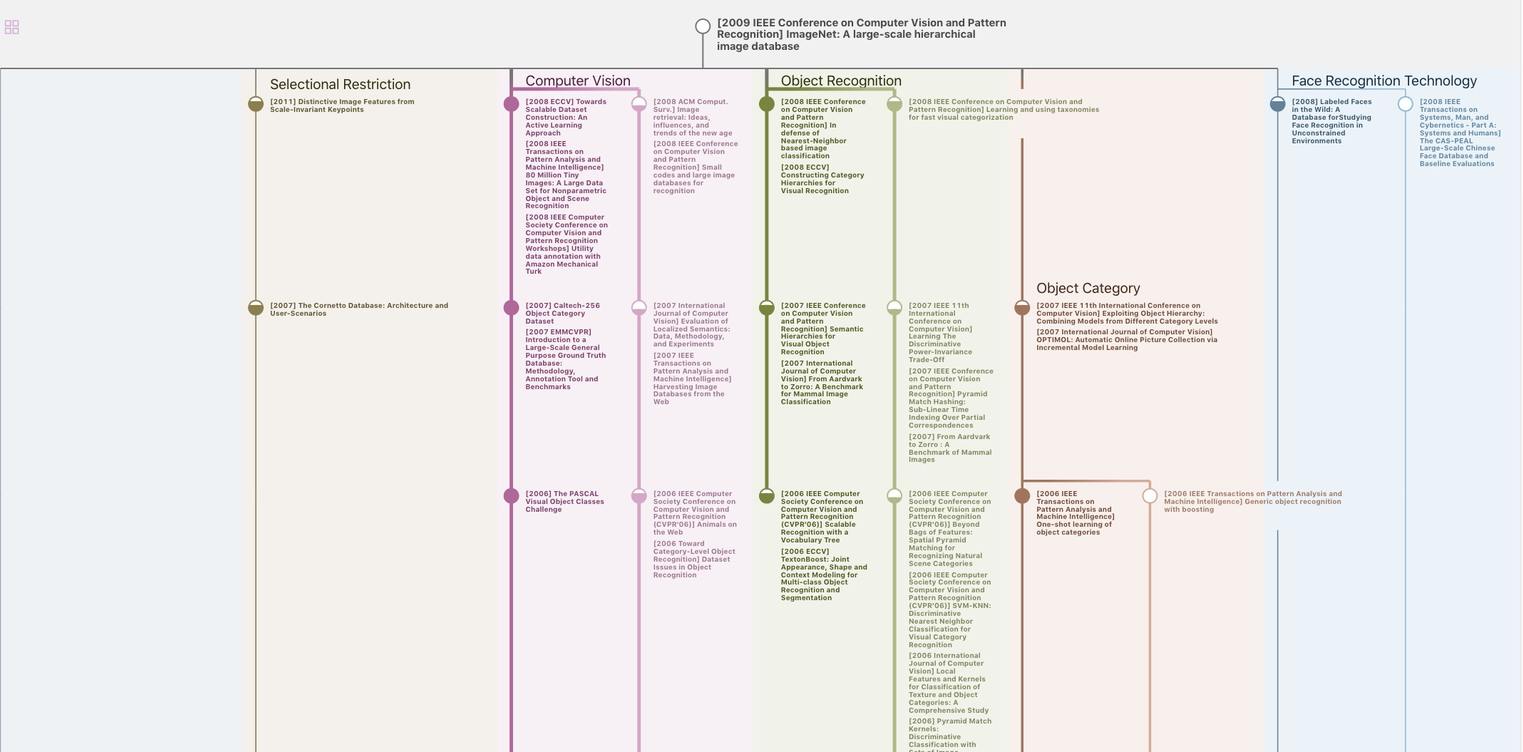
生成溯源树,研究论文发展脉络
Chat Paper
正在生成论文摘要