3D Mineral Prospectivity Modeling Using Multi-Scale 3D Convolution Neural Network and Spatial Attention Approaches
GEOCHEMISTRY(2024)
关键词
3D,Mineral prospectivity modeling,Convolutional neural network, spatial attention mechanism,Multi-scale features
AI 理解论文
溯源树
样例
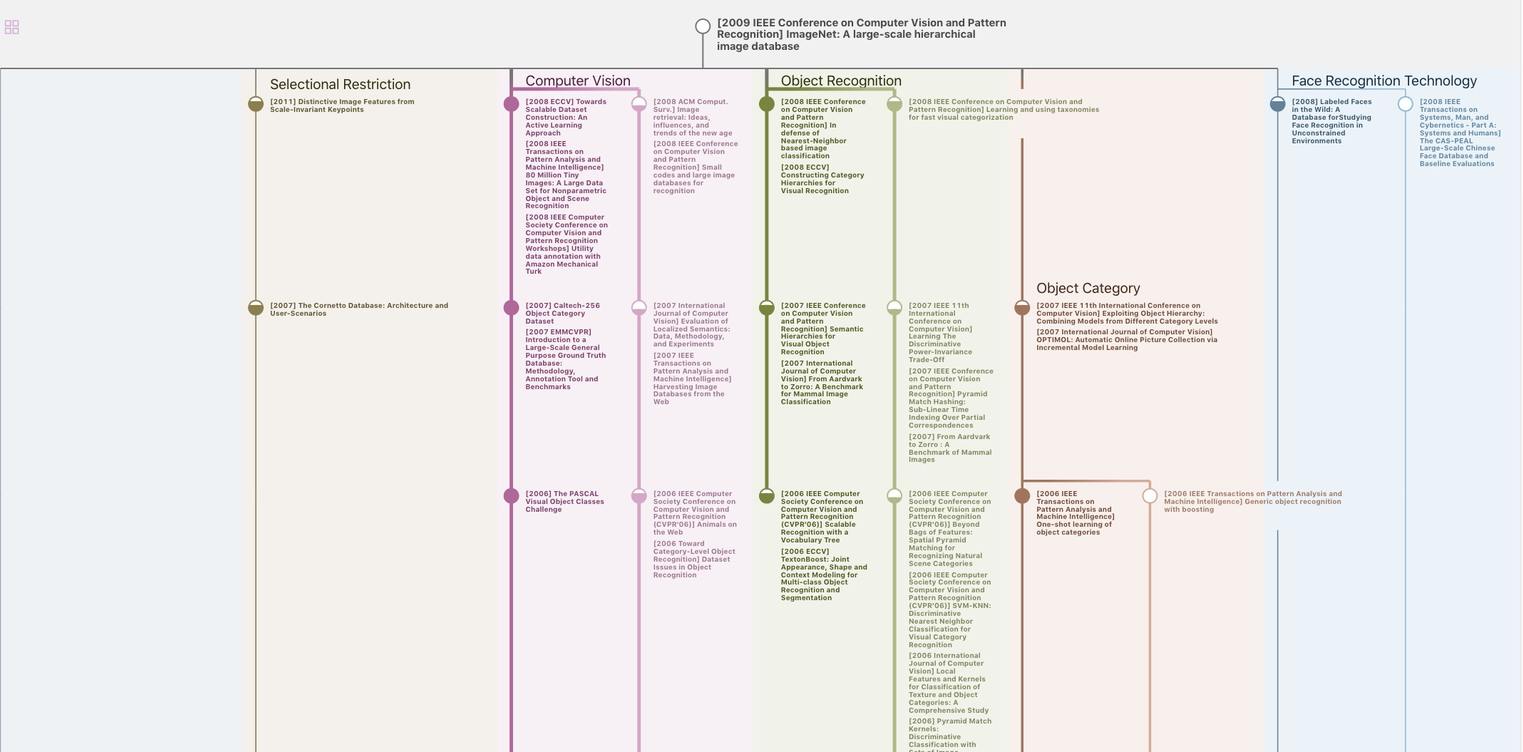
生成溯源树,研究论文发展脉络
Chat Paper
正在生成论文摘要