A large-scale graph clustering method for cell conditions spatio-temporal localization in aluminum electrolysis
Information Sciences(2024)
Abstract
Sensor nodes (industrial nodes) clustering is an efficacious method for gaining local working conditions real-timely in the production process. The existing deep graph neural networks-based industrial nodes clustering studies construct static graphs to describe the potential spatial correlation among industrial nodes, but the temporal correlation among industrial nodes is ignored, resulting in inferior real-time performance. Meanwhile, these deep learning models fail to obtain good clustering results due to the large-scale and noise-containing characteristics of industrial nodes. In this paper, a large-scale graph clustering method (LsGCM) is proposed for cell conditions spatio-temporal localization in aluminum electrolysis to address the above issues. Specifically, a spatio-temporal graph construction method based on industrial process mechanism knowledge and local working condition boundary types statistical feature fusion is proposed for capturing effective spatio-temporal correlations between industrial nodes. Then, hysteresis knowledge of industrial production system is used to guide the nodes merging, thereby avoiding the incorrect merging of time-asynchronous nodes when performing the spatio-temporal region detection of local working conditions. Finally, combined with the actual spatio-temporal location information of industrial nodes, a novel spatio-temporal information expansion method (StIEM) of the sub-spatio-temporal graph describing local working condition is proposed for obtaining spatio-temporal feature information of the neighborhood, which is beneficial to improve the performance of industrial node clustering. Validation studies on a numerical simulation example and multiple real aluminum electrolysis industrial datasets certificate the effectiveness and superiority of the proposed method. In particular, compared with existing graph clustering methods, our method improves the clustering accuracy by 7.79%.
MoreTranslated text
Key words
Large-scale graph clustering,Spatio-temporal graph,Aluminum electrolysis industrial process,Working conditions spatio-temporal localization,Spatio-temporal location information
AI Read Science
Must-Reading Tree
Example
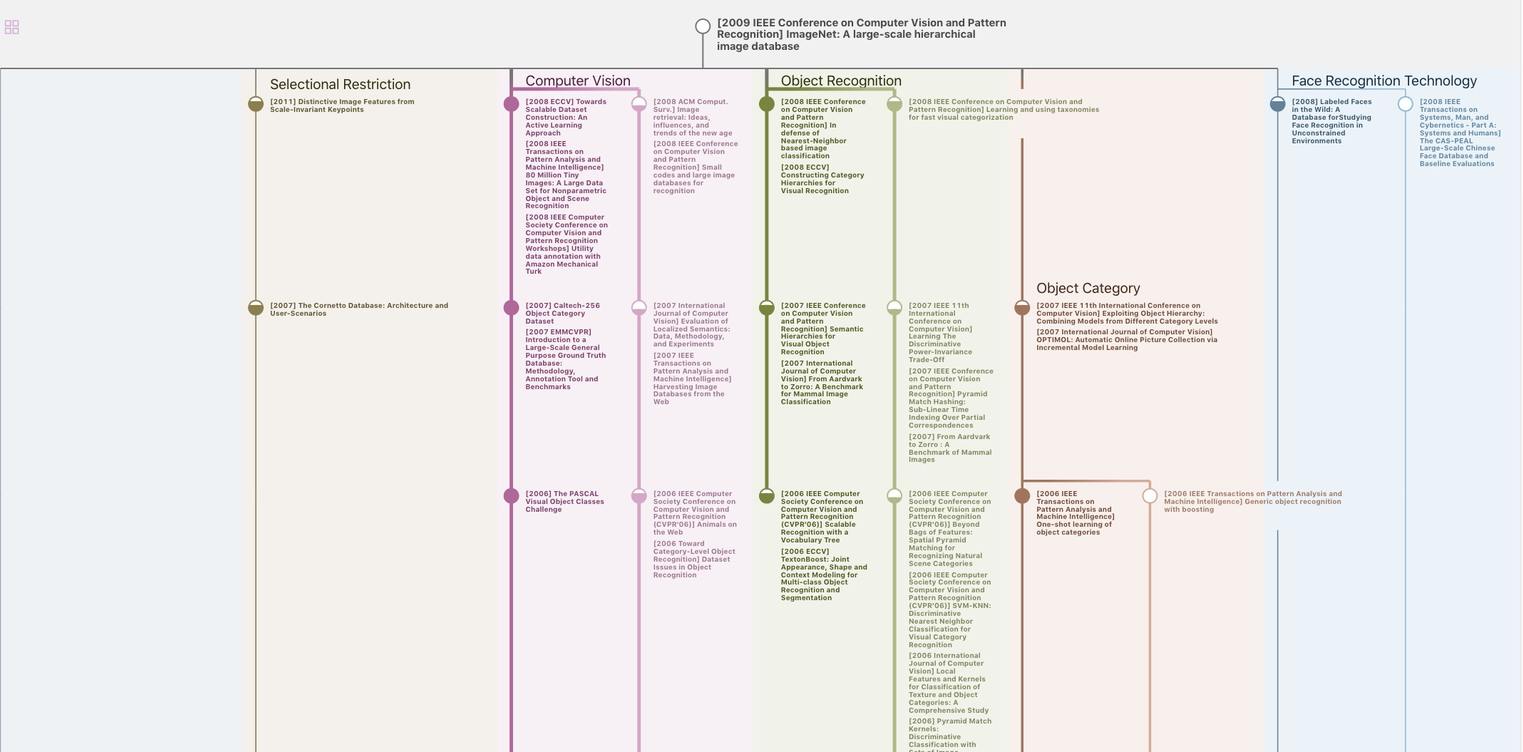
Generate MRT to find the research sequence of this paper
Chat Paper
Summary is being generated by the instructions you defined