Meta-learning with hypernetworks: Cost-effective fault detection in Parabolic Trough plants
Engineering Applications of Artificial Intelligence(2024)
摘要
Detecting receivers with broken glass envelopes in Parabolic Trough plants presents a crucial challenge in Concentrated Solar Power systems. While existing research has addressed this problem, some limitations persist, particularly in the context of data collection and the accuracy of the proposed methods. To overcome these challenges, we propose a fully automated and cost-efficient solution. Leveraging thermal camera data, we develop a novel cropping algorithm that achieves a 91% success rate in detecting the location of the receiver tubes without the need for labeled samples. Additionally, we introduce a luminescence-based filter for color images in Red–Green–Blue format to assess biases in the training and test sets. To solve our target problem we rely on Convolutional Neural Network architectures augmented with Hypernetworks as meta-learners. Our proposal is inspired by traditional voting methods but it is trainable end-to-end. In our test set, the image-based meta-learners obtain the best performance over all evaluation metrics. Furthermore, we provide experimentally driven insights to the weaknesses and strengths of our approach. Combining the experimental results with the luminescence-based filter, we achieve a robust method with a 95% F1-Score for detecting broken glass samples, including edge cases. Our approach surpasses the best individual learner by 2.1%–5.8%, depending on brightness conditions.
更多查看译文
关键词
Fault detection,Parabolic Trough,Meta-learning,Hypernetworks,Data collection
AI 理解论文
溯源树
样例
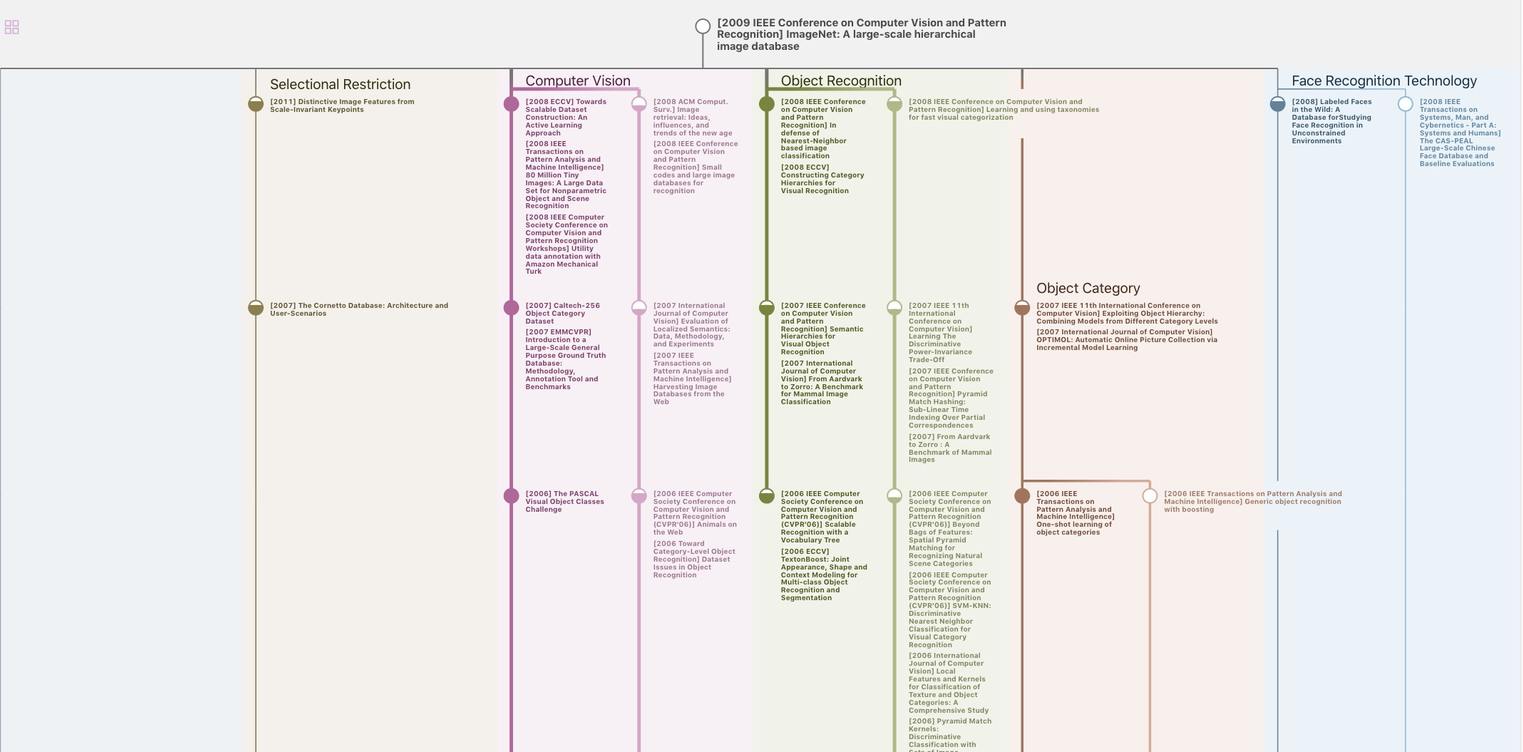
生成溯源树,研究论文发展脉络
Chat Paper
正在生成论文摘要