Blockchain transaction deanonymization using ensemble learning
Multimedia Tools and Applications(2024)
Abstract
Bitcoin is a digital currency that provides a way to transact without any trusted intermediary; however, privacy is an issue. Numerous deanonymization endeavors have been proposed, in spite of the fact that Bitcoin addresses aren’t linked with a specific identity. In this work, blockchain transactions are deanonymized using ensemble learning. An excess of four million labeled dataset samples comprising user activities such as pools, services, gambling, and exchanges have been gathered from various repositories and prepared for training and validation to perform the classification. The main aim is to deanonymize blockchain transactions via classification and separate legitimate ones from illegitimate ones. On the class imbalanced dataset, remarkable cross-validation accuracy was attained using the EXtreme Gradient Boosting with default parameters and hyperparameters. Using EXtreme Gradient Boosting, Random Forest, and Bagging on the class-balanced dataset produced the best cross-validation accuracy when using the default parameters and hyperparameters. The empirical findings indicate that the effectiveness of the proposed deanonymization using the proposed ensemble learning model has achieved up to 98.45
MoreTranslated text
Key words
Blockchain,Supervised machine learning,Ensemble learning,Hyperparameter tuning,Randomized search,Grid search
AI Read Science
Must-Reading Tree
Example
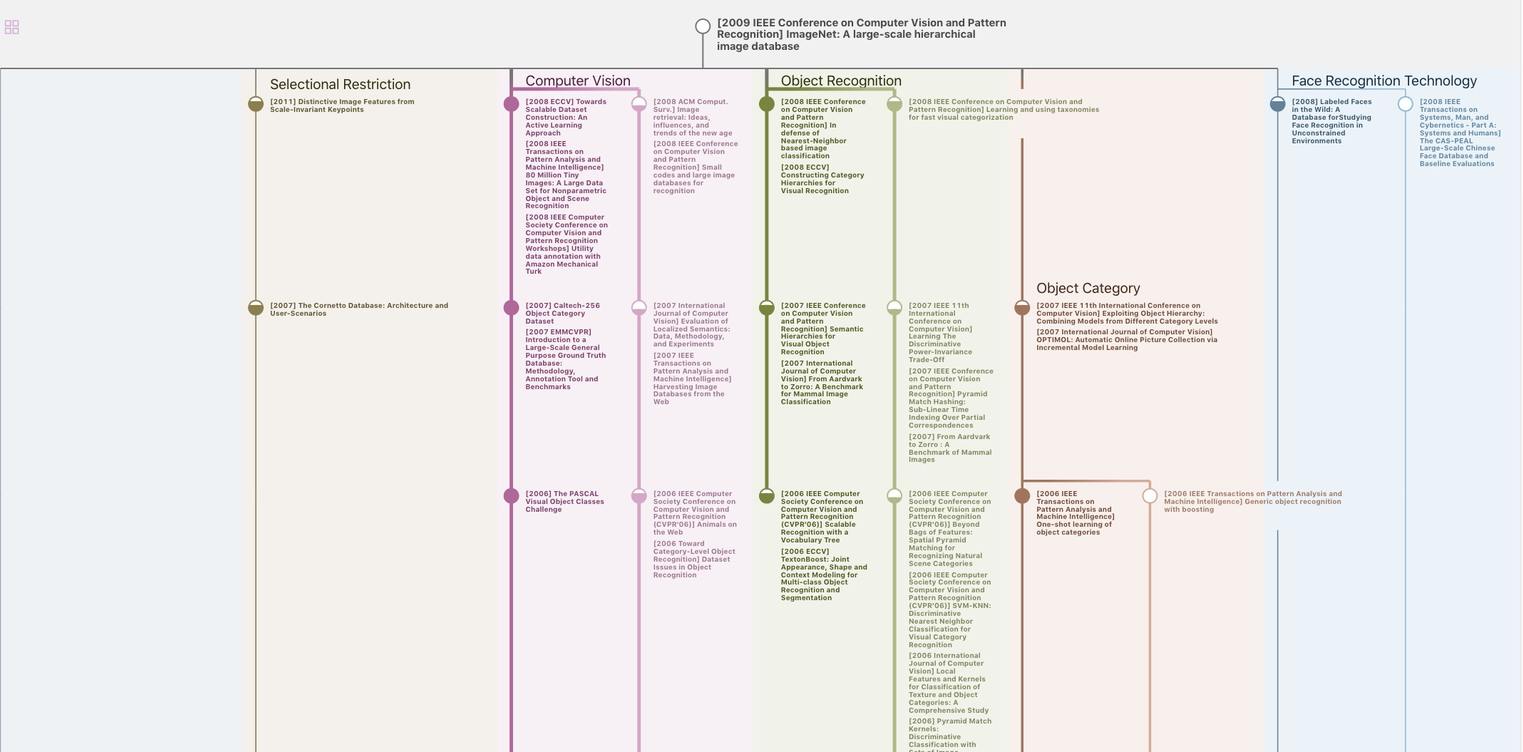
Generate MRT to find the research sequence of this paper
Chat Paper
Summary is being generated by the instructions you defined