Prediction of storm surge in the Pearl River Estuary based on data-driven model
Frontiers in Marine Science(2024)
摘要
Storm surges, a significant coastal hazard, cause substantial damage to both property and lives. Precise and efficient storm surge models are crucial for long-term risk assessment and guiding emergency management decisions. While high-fidelity dynamic models offer accurate predictions, their computational costs are substantial. Hence, recent efforts focus on developing data-driven storm surge surrogate models. This study focuses on the Pearl River Estuary in Guangdong Province. Initially, the dynamic ADvanced CIRCulation (ADCIRC) model was utilized to construct storm surge data for 16 historical typhoons, serving as training, validation, and testing data for data-driven models. Subsequently, Long Short-Term Memory (LSTM), Convolutional Neural Networks (CNN), and Informer deep learning (DL) models were employed for forecasting of storm surge over the next 1h, 3h, 6h, 12h, and 18h. Finally, Shapley Additive exPlanations (SHAP) values were used for interpretability analysis of the input factors across different models. Results indicated that the proposed DL storm surge prediction model can effectively replicate the dynamic model’s simulation results in short-term forecasts, significantly reducing computational costs. This model offers valuable scientific assistance for future coastal storm surge forecasts in the Greater Bay Area.
更多查看译文
关键词
storm surge,ADCIRC,DL,SHAP,intelligent forecasting
AI 理解论文
溯源树
样例
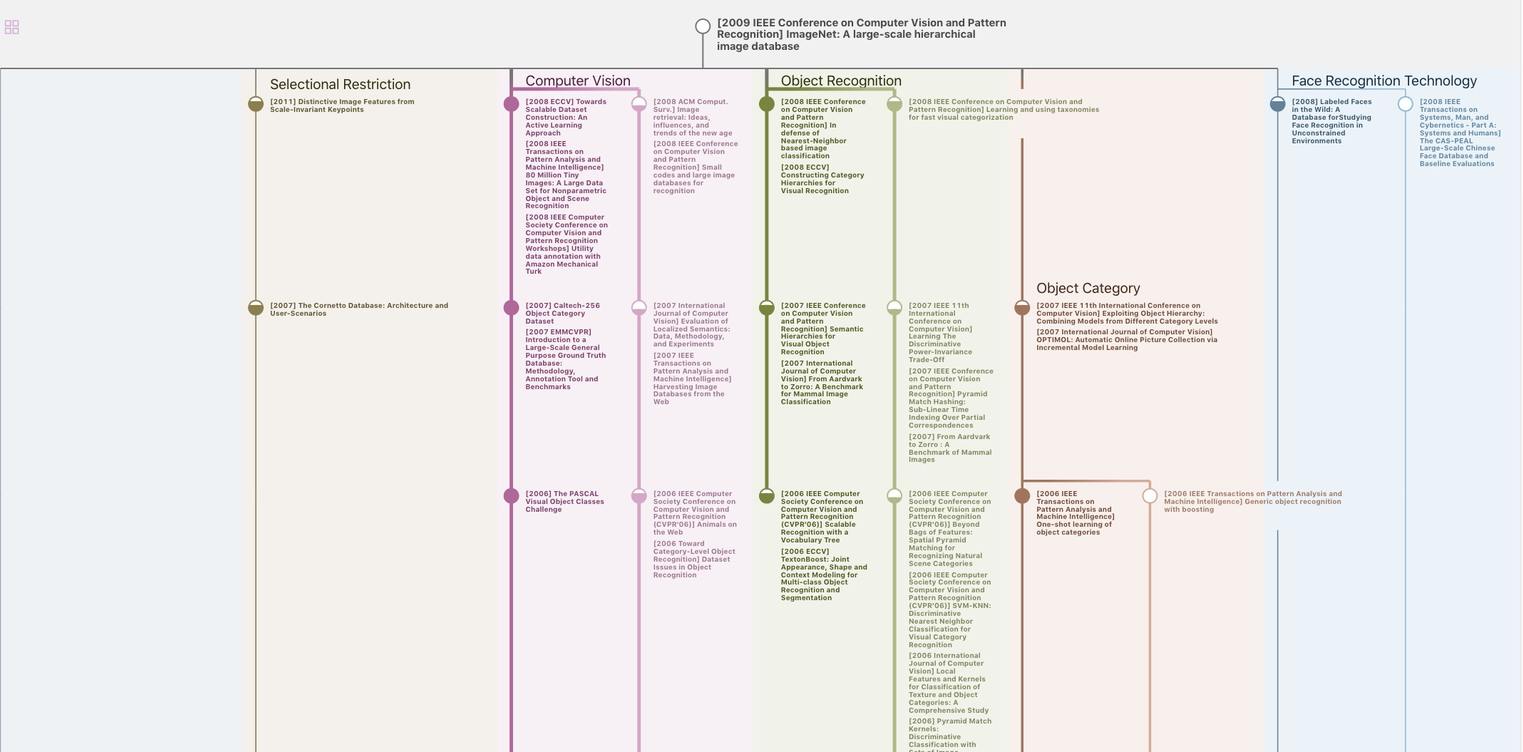
生成溯源树,研究论文发展脉络
Chat Paper
正在生成论文摘要