Estimation of wheat biophysical variables through UAV hyperspectral remote sensing using machine learning and radiative transfer models
Computers and Electronics in Agriculture(2024)
摘要
Accurate and timely estimation of crop biophysical variables is necessary for monitoring crop growth and implementing effective nutrient management practices. Incorporating machine learning multivariate models with UAV-based hyperspectral imaging provides a fast non-destructive and near real-time prediction of these variables. In the present study, the hyperspectral data in the spectral range of 400–1000 nm from an imaging spectrometer integrated into an unmanned aerial vehicle (UAV) was used for mapping experimental fields of wheat crop in ICAR-Indian Agricultural Research Institute (ICAR-IARI), New Delhi. The imaging spectroradiometer has a spectral resolution of 2.2 nm with 269 distinct bands and imaging with an ultrahigh spatial resolution of 4 cm was employed for the experiment. Five competitive machine learning algorithms, i.e., artificial neural network (ANN), extreme learning machine (ELM), multivariate adaptive regression spline (MARS), random forest (RF), and support vector machine (SVM) were evaluated for predicting biophysical variables of the wheat crop, namely leaf area index (LAI), leaf chlorophyll content (LCC), and canopy chlorophyll content (CCC). The physical radiative transfer model (RTM) PROSAIL was also applied in combination with a machine learning regression algorithms toolbox as available in the automated radiative transfer model’s operator (ARTMO) software, leading to hybrid models. In the empirical analysis, ELM outperformed the other algorithms with maximum validation R2 of 0.948, 0.990, and 0.963 for LAI, LCC, and CCC, respectively. However, in the case of hybrid modelling, on validated against simulated data ANN outperforms the other models with maximum validation R2 of 0.983, 0.969, and 0.998 for LAI, LCC, and CCC, respectively. Validated against real data, the NRMSE values obtained for LAI, LCC, and CCC retrieved maps are 24.51 %, 38.74 %, and 36.16 %, respectively. The accurate retrieval of LAI and CCC with the highest prediction accuracy was obtained using a hybrid approach, while empirical multivariate regression applied to image spectra showed the best performance for LCC mapping. The study provides feasible solutions to infer the state of croplands in support of better farm management practices.
更多查看译文
关键词
UAV,Hyperspectral remote sensing,Leaf area index,Chlorophyll content,Machine learning,Radiative transfer model
AI 理解论文
溯源树
样例
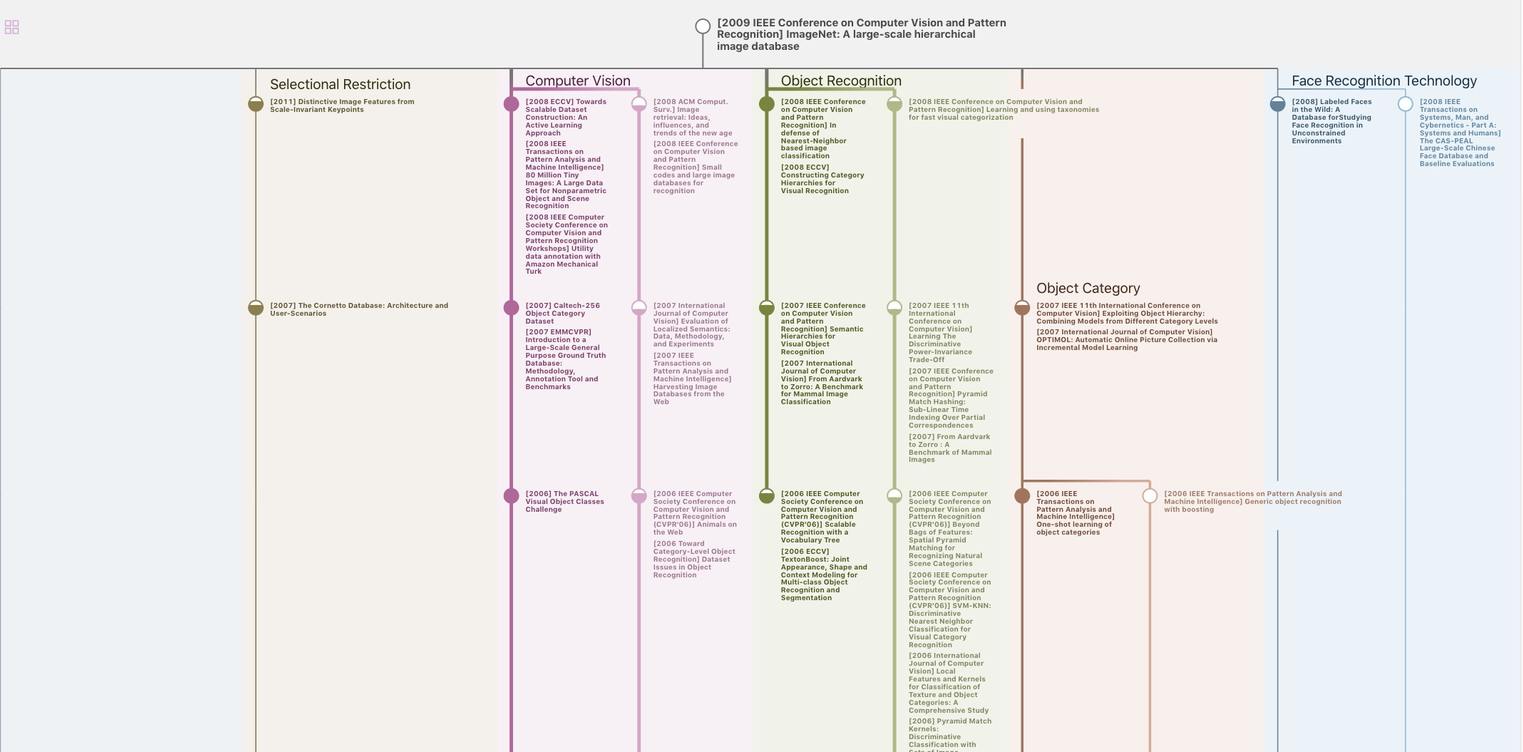
生成溯源树,研究论文发展脉络
Chat Paper
正在生成论文摘要