Robustness verification of k-nearest neighbors by abstract interpretation
Knowledge and Information Systems(2024)
Abstract
We study the certification of stability properties, such as robustness and individual fairness, of the k-nearest neighbor algorithm (kNN). Our approach leverages abstract interpretation, a well-established program analysis technique that has been proven successful in verifying several machine learning algorithms, notably, neural networks, decision trees, and support vector machines. In this work, we put forward an abstract interpretation-based framework for designing a sound approximate version of the kNN algorithm, which is instantiated to the interval and zonotope abstractions for approximating the range of numerical features. We show how this abstraction-based method can be used for stability, robustness, and individual fairness certification of kNN. Our certification technique has been implemented and experimentally evaluated on several benchmark datasets. These experimental results show that our tool can formally prove the stability of kNN classifiers in a precise and efficient way, thus expanding the range of machine learning models amenable to robustness certification.
MoreTranslated text
Key words
k-nearest neighbors,Robustness,Individual fairness,Data poisoning,Formal certification
AI Read Science
Must-Reading Tree
Example
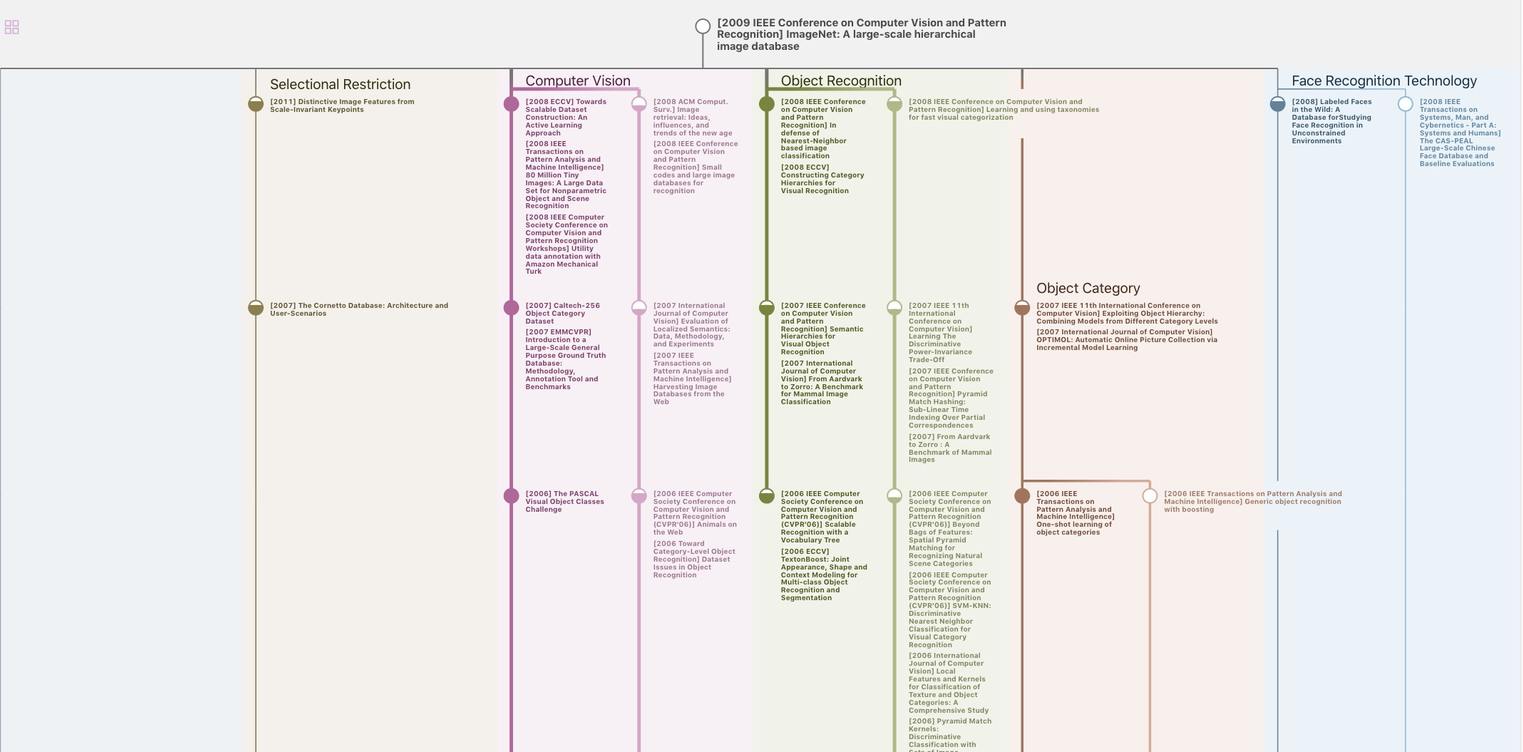
Generate MRT to find the research sequence of this paper
Chat Paper
Summary is being generated by the instructions you defined