FATN: Flow-Guided Alignment-Based Transformer Network for High Dynamic Range Imaging
2023 5th International Academic Exchange Conference on Science and Technology Innovation (IAECST)(2023)
Abstract
High dynamic range (HDR) imaging presents a formidable challenge, aiming to produce ghost-free HDR images featuring lifelike details. The alignment-merger pipeline is a popular choice for this task. However, training deformable alignment is difficult, and existing methods struggle with ghost removal and detail recovery. In this work, a new flow-guided alignment-based transformer network (FATN) is proposed to cope with HDR imaging in dynamic scenes. To improve the training stability of deformable alignment, we propose a flow-guided deformable convolution module (FDCM). To jointly extract both global and local dependencies, we propose a dual-branch transformer merger layer (DTML) as the basic component of the feature merger network. DTML combines a global branch to model remote motions and a local branch for neighborhood content similarity. Extensive experiments showcase the superior performance of the proposed approach over other competing methods both quantitatively and qualitatively.
MoreTranslated text
Key words
high dynamic range imaging,alignment-merger pipeline,flow-guided deformable convolution,dual-branch transformer merger
AI Read Science
Must-Reading Tree
Example
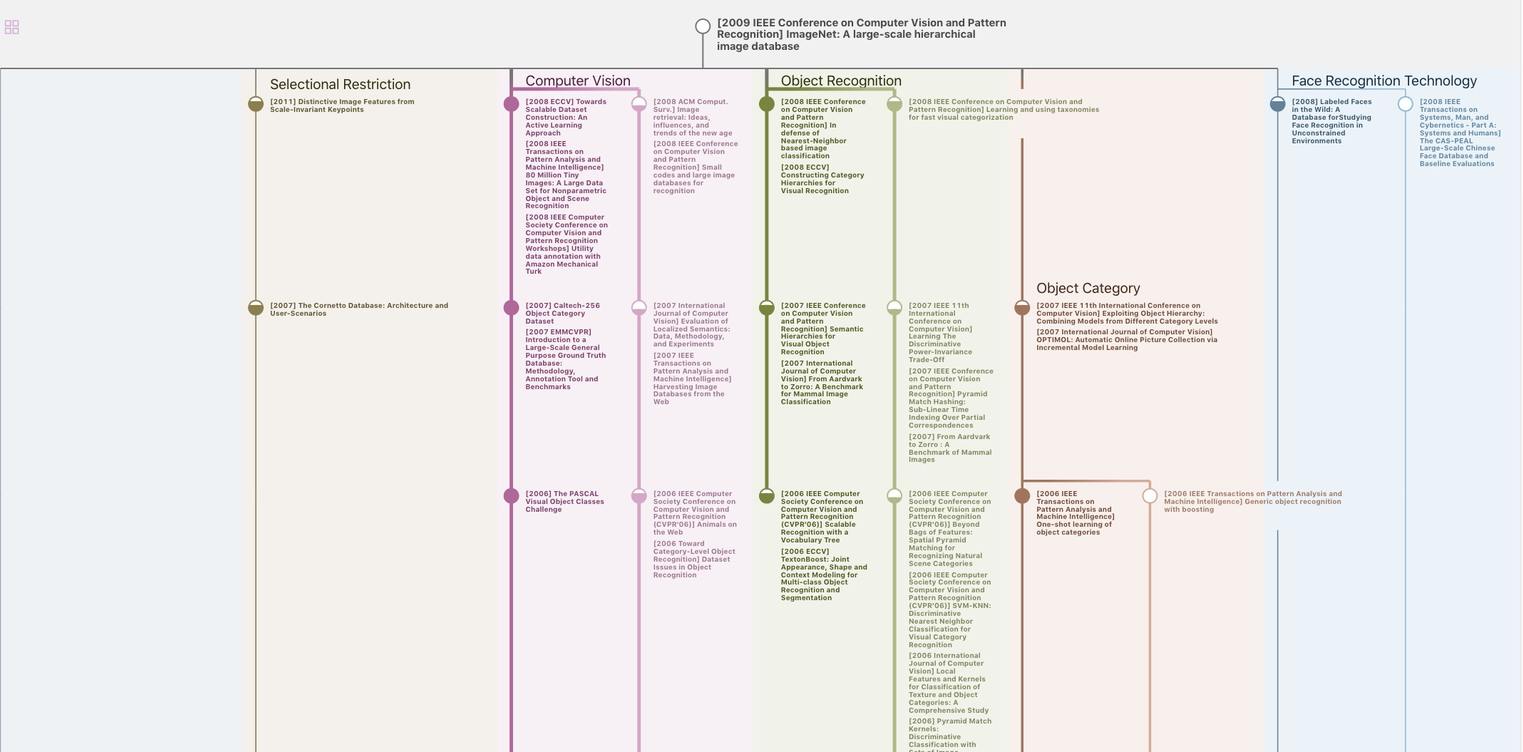
Generate MRT to find the research sequence of this paper
Chat Paper
Summary is being generated by the instructions you defined