Risk Assessment of Distribution Network Nodes based on Data-driven Awareness Model by Adaptive Online Deep Learning Algorithm
2023 5th International Academic Exchange Conference on Science and Technology Innovation (IAECST)(2023)
Abstract
The structure of the distribution network has become increasingly complex due to the continuous penetration of distributed generations. Additionally, the overgeneration of distributed generations can lead to fault conditions, such as overvoltage at nodes. Thus, it is crucial to conduct risk assessments of nodes to plan effective preventive measures. To address the challenges of limited node information and frequent topology changes, this paper proposes a risk assessment method for distribution network nodes based on a data-driven awareness model. First, implicit information is extracted from power flow variables, followed by construction of a data-driven perceptual model using an adaptive online deep learning algorithm. Subsequently, a node risk assessment indicator set is constructed by incorporating three indicators: the ratio of injected power at the node, overall voltage margin, and risk indicator for node voltage overlimit. The entropy weight method is then utilized to assign weights and aggregate these indicators, forming a comprehensive node risk assessment indicator. Finally, the feasibility and effectiveness of the proposed method are demonstrated through its application to the IEEE 33-node system arithmetic example.
MoreTranslated text
Key words
component,notes risk assessment,data-driven awareness,power flow mapping model
AI Read Science
Must-Reading Tree
Example
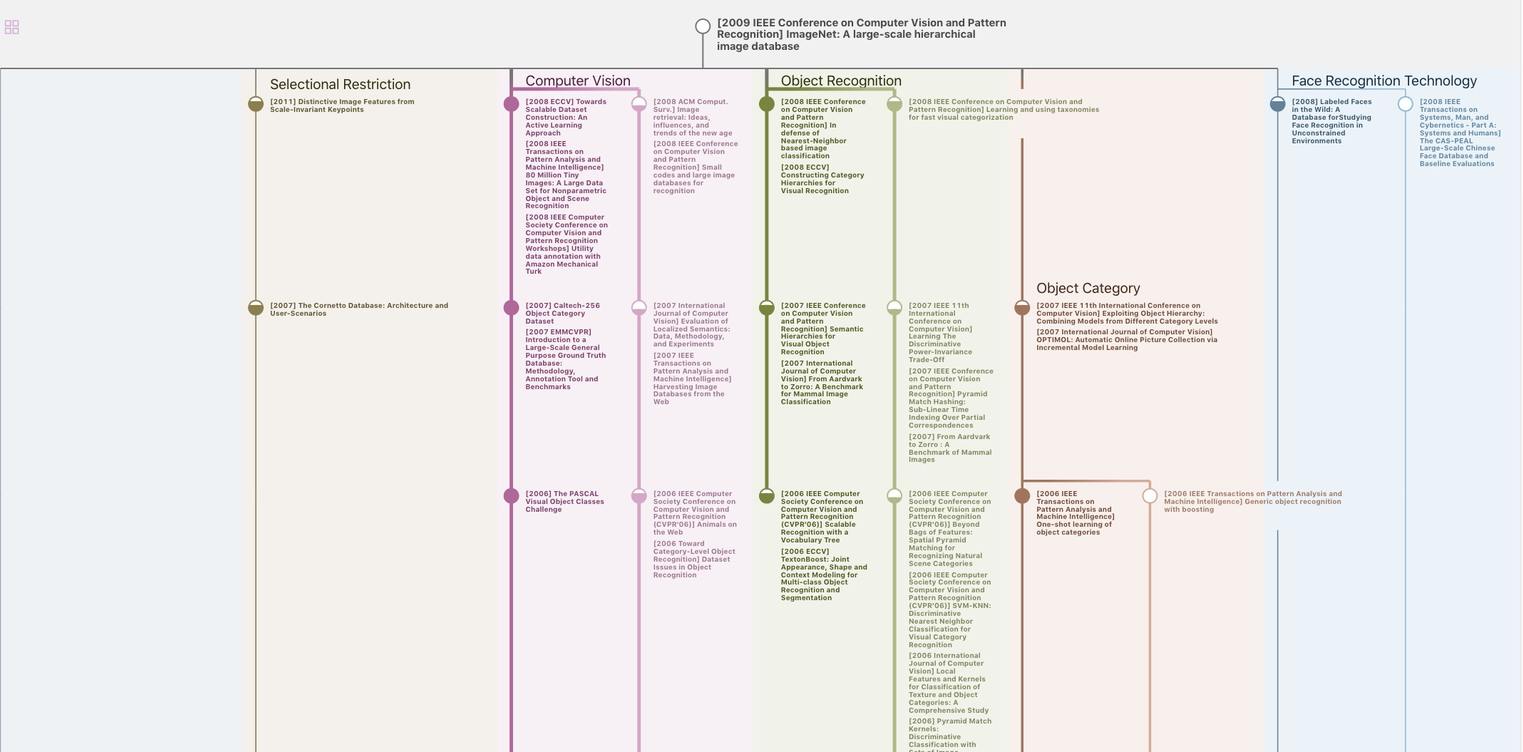
Generate MRT to find the research sequence of this paper
Chat Paper
Summary is being generated by the instructions you defined