Decomposed intrinsic mode functions and deep learning algorithms for water quality index forecasting
Neural Computing and Applications(2024)
Abstract
The water quality index (WQI) serves as a global representation of river water quality (WQ). Existing studies related to the WQI have mainly focused on two aspects: (i) a WQI point estimation using multiple WQ inputs; and (ii) a one-step-ahead WQI forecasting with datasets of lower temporal resolution. These approaches, however, are limited in their ability to forecast future trends of the WQI for timely and prompt responses to pollution events. In this study, the deep learning algorithms, namely the long short-term memory (LSTM) and the gated recurrent unit (GRU), were selected for direct multi-step-ahead WQI forecasting. To enhance the capability of the models in capturing their temporal patterns, the input signal was pre-decomposed using the empirical mode decomposition (EMD) and variational mode decomposition (VMD) into several intrinsic mode functions (IMFs). The characteristics of these IMFs were then analyzed and used to ease model learning on capturing their temporal patterns. Our study shows that the selection of signal decomposition strategies significantly impact the model performance. Both deep learning algorithms offered comparable performances, with the VMD-LSTM exhibiting the lowest prediction errors (MAPE = 1.9237
MoreTranslated text
Key words
Long short-term memory,Gated recurrent unit,Signal decomposition,Multi-step forecasting
AI Read Science
Must-Reading Tree
Example
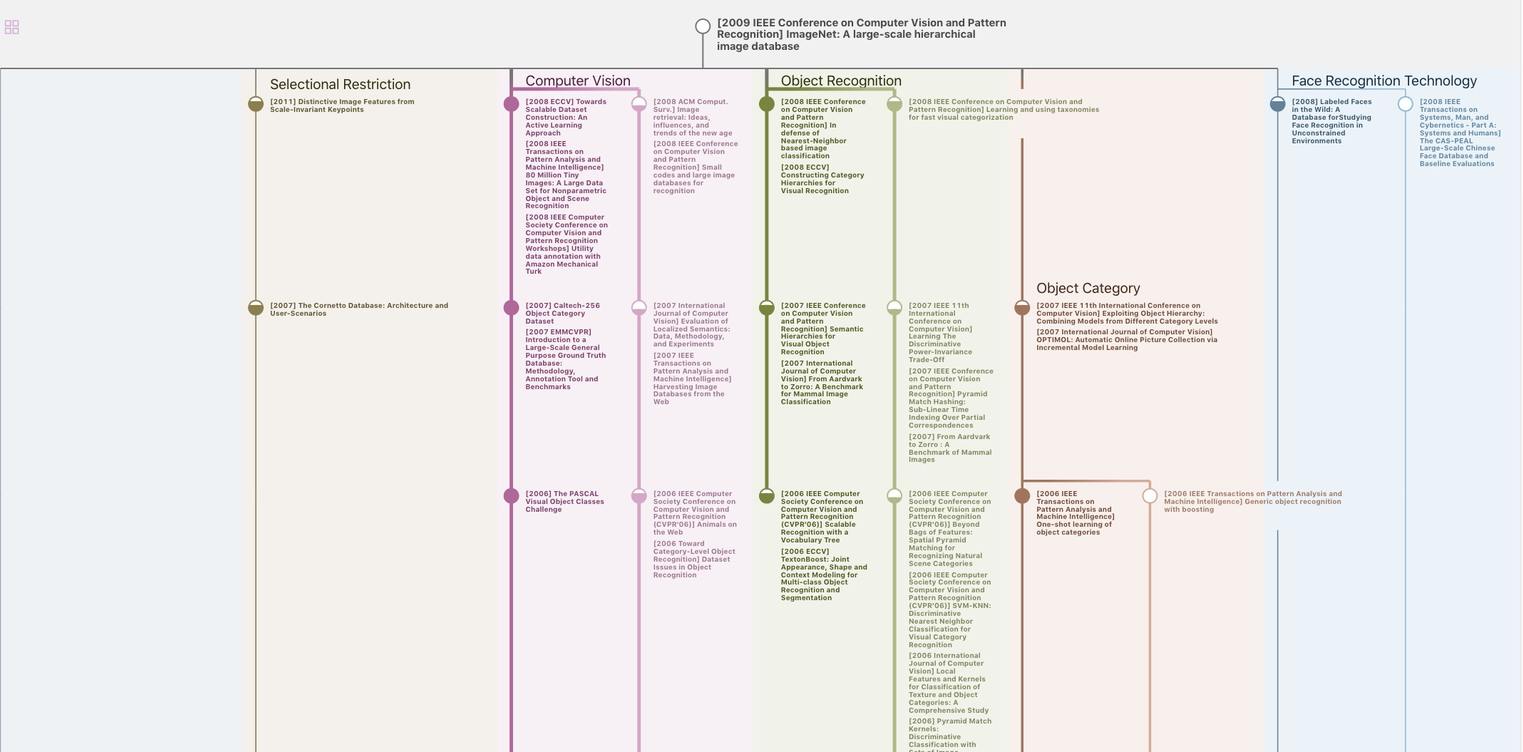
Generate MRT to find the research sequence of this paper
Chat Paper
Summary is being generated by the instructions you defined