Successive Convexification for Trajectory Optimization with Continuous-Time Constraint Satisfaction
arxiv(2024)
摘要
We present successive convexification, a real-time-capable solution method
for nonconvex trajectory optimization, with continuous-time constraint
satisfaction and guaranteed convergence, that only requires first-order
information. The proposed framework combines several key methods to solve a
large class of nonlinear optimal control problems: (i) exterior penalty-based
reformulation of the path constraints; (ii) generalized time-dilation; (iii)
multiple-shooting discretization; (iv) ℓ_1 exact penalization of the
nonconvex constraints; and (v) the prox-linear method, a sequential convex
programming (SCP) algorithm for convex-composite minimization. The
reformulation of the path constraints enables continuous-time constraint
satisfaction even on sparse discretization grids and obviates the need for mesh
refinement heuristics. Through the prox-linear method, we guarantee convergence
of the solution method to stationary points of the penalized problem and
guarantee that the converged solutions that are feasible with respect to the
discretized and control-parameterized optimal control problem are also
Karush-Kuhn-Tucker (KKT) points. Furthermore, we highlight the specialization
of this property to global minimizers of convex optimal control problems,
wherein the reformulated path constraints cannot be represented by canonical
cones, i.e., in the form required by existing convex optimization solvers. In
addition to theoretical analysis, we demonstrate the effectiveness and
real-time capability of the proposed framework with numerical examples based on
popular optimal control applications: dynamic obstacle avoidance and rocket
landing.
更多查看译文
AI 理解论文
溯源树
样例
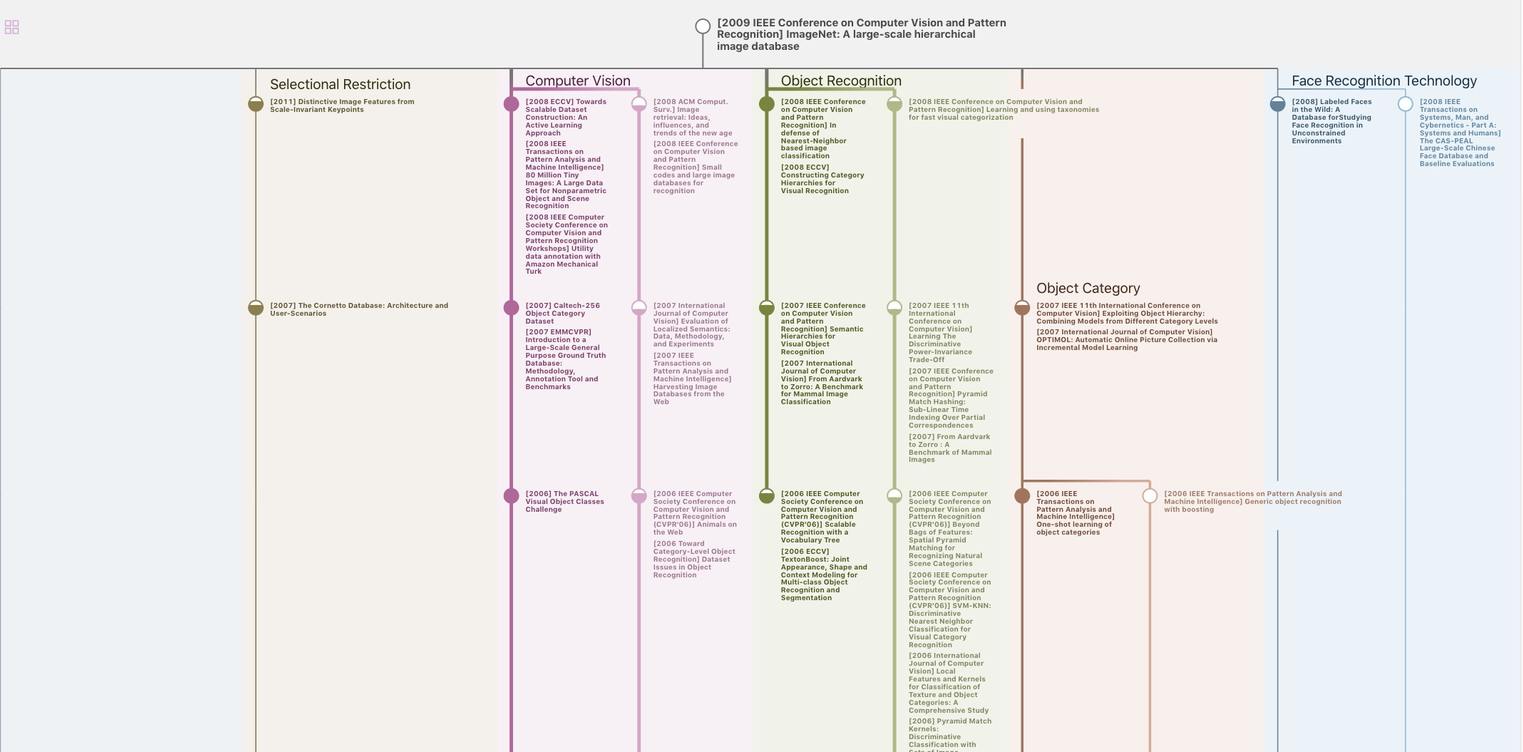
生成溯源树,研究论文发展脉络
Chat Paper
正在生成论文摘要