Pearls from Pebbles: Improved Confidence Functions for Auto-labeling
arxiv(2024)
摘要
Auto-labeling is an important family of techniques that produce labeled
training sets with minimum manual labeling. A prominent variant,
threshold-based auto-labeling (TBAL), works by finding a threshold on a model's
confidence scores above which it can accurately label unlabeled data points.
However, many models are known to produce overconfident scores, leading to poor
TBAL performance. While a natural idea is to apply off-the-shelf calibration
methods to alleviate the overconfidence issue, such methods still fall short.
Rather than experimenting with ad-hoc choices of confidence functions, we
propose a framework for studying the optimal TBAL confidence function.
We develop a tractable version of the framework to obtain
(Confidence functions for Efficient and Reliable Auto-labeling), a new post-hoc
method specifically designed to maximize performance in TBAL systems. We
perform an extensive empirical evaluation of our method and
compare it against methods designed for calibration. achieves
up to 60% improvements on coverage over the baselines while maintaining
auto-labeling error below 5% and using the same amount of labeled data as
the baselines.
更多查看译文
AI 理解论文
溯源树
样例
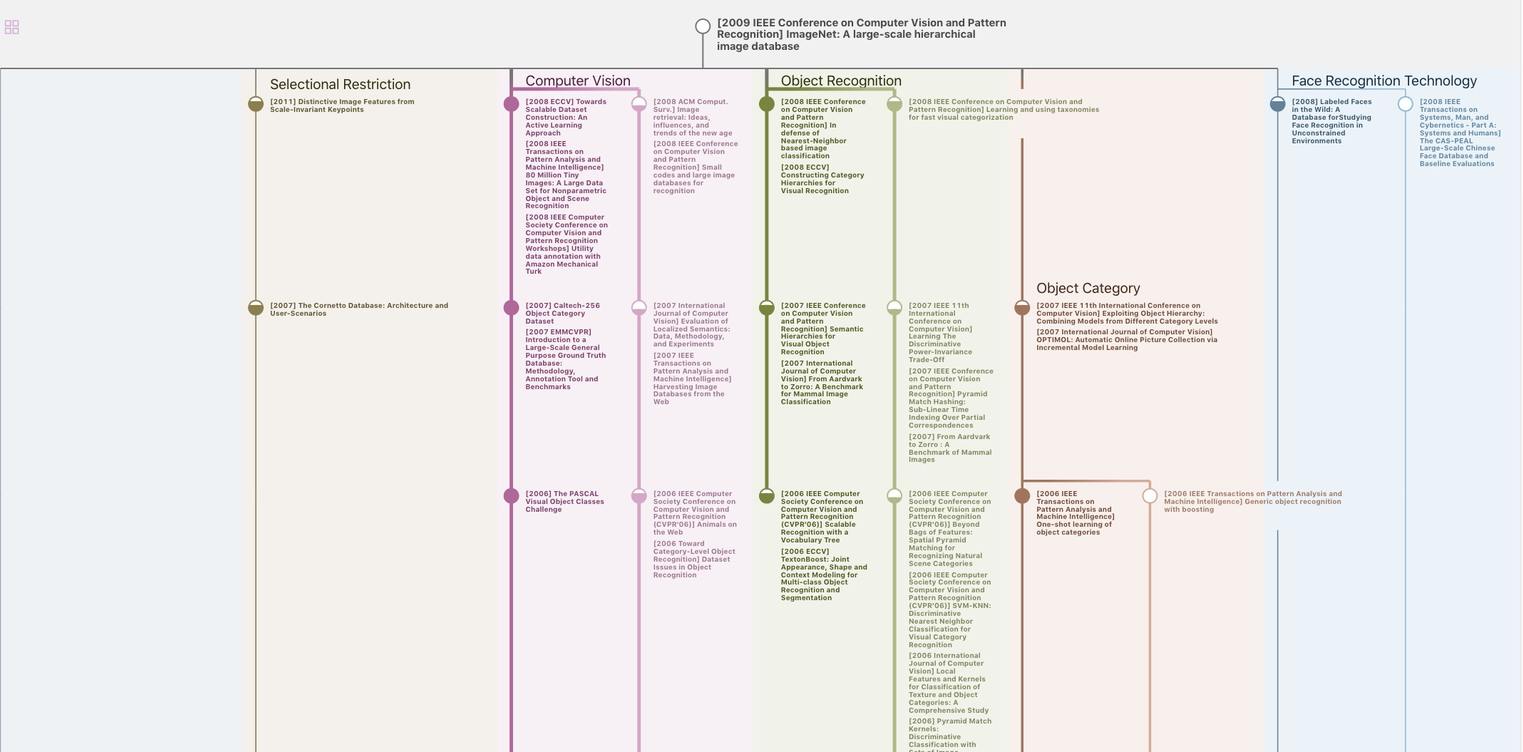
生成溯源树,研究论文发展脉络
Chat Paper
正在生成论文摘要