Supercompiler Code Optimization with Zero-Shot Reinforcement Learning
CoRR(2024)
摘要
Effective code optimization in compilers plays a central role in computer and
software engineering. While compilers can be made to automatically search the
optimization space without the need for user interventions, this is not a
standard practice since the search is slow and cumbersome. Here we present
CodeZero, an artificial intelligence agent trained extensively on large data to
produce effective optimization strategies instantly for each program in a
single trial of the agent. To overcome the huge range of possible test
programs, we prepare a large dataset of training programs that emphasize
quality, naturalness, and diversity. To tackle the vast space of possible
optimizations, we adapt deep reinforcement learning to train the agent in a
sample-efficient manner through interacting with a world model of the compiler
environment. Evaluation on both benchmark suites and production-level code
optimization problems demonstrates our agent's supercompiler performances and
zero-shot generalization abilities, outperforming built-in optimization options
designed by compiler experts. Our methodology kindles the great potential of
artificial intelligence for engineering and paves the way for scaling machine
learning techniques in the realm of code optimization.
更多查看译文
AI 理解论文
溯源树
样例
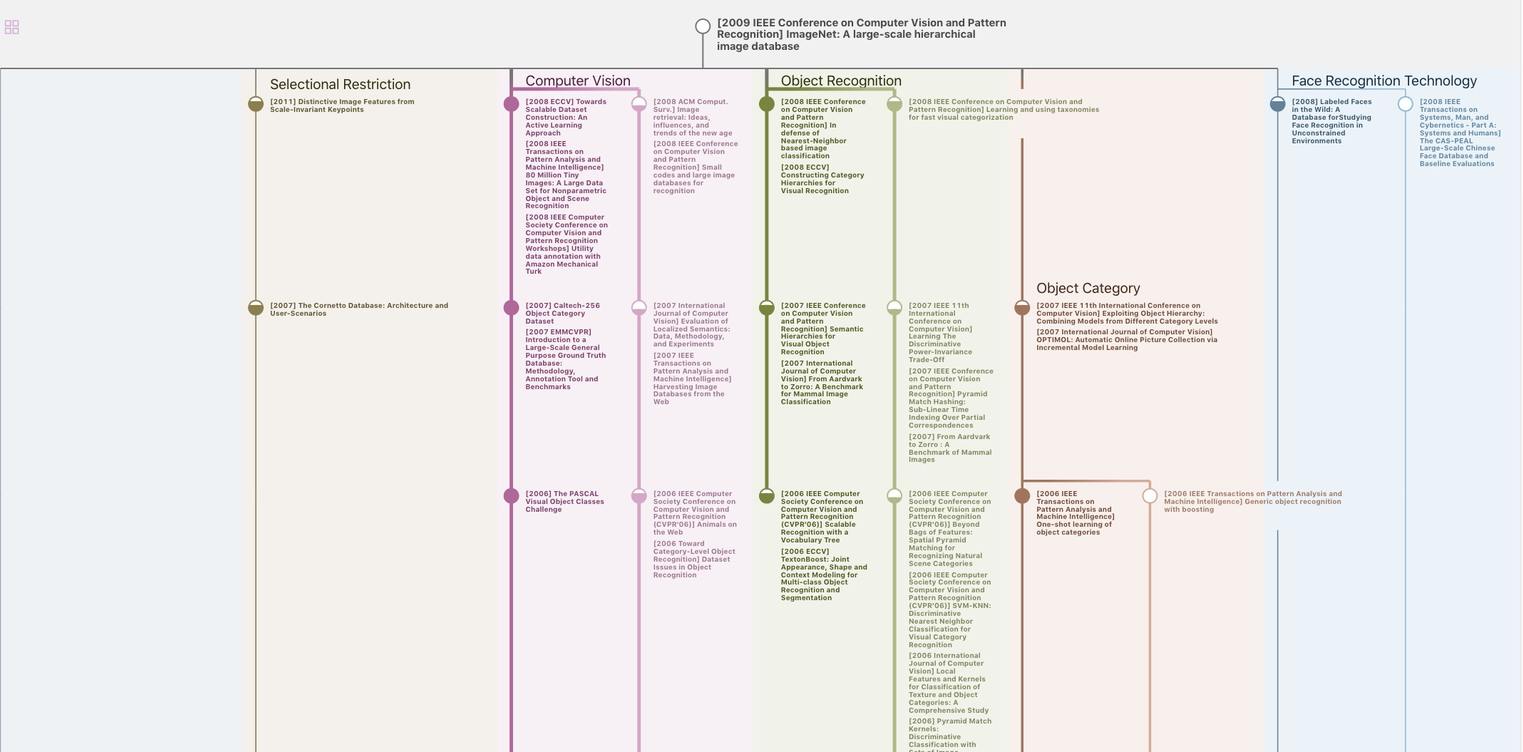
生成溯源树,研究论文发展脉络
Chat Paper
正在生成论文摘要