A novel two-stage wrapper feature selection approach based on greedy search for text sentiment classification
Neurocomputing(2024)
摘要
Sentiment analysis is a crucial step in obtaining subjective data from online text sources. Nevertheless, the substantial challenge of high dimensionality prevails within text classification. Addressing this, dimension reduction emerges as a valuable approach to enhance the efficacy of classification in the domain of machine learning. The discerning removal of redundant features not only expedites training processes but also bolsters the achievement of accurate classifications. It is worth noting that the effectiveness of distinct feature selection methodologies can be contingent upon the unique attributes inherent in diverse datasets. Within the purview of this investigation, a novel two-stage approach is introduced, characterized by a greedy search-based wrapper feature selection algorithm. The underpinning of this algorithm involves leveraging the outcomes yielded by filter-based feature selection techniques to establish a prioritized sequence for the scrutiny of features within the proposed framework. This strategic sequencing harnesses the cumulative insights from a series of filter-based methodologies, thereby facilitating the curation of feature subsets that underscore pivotal attributes. Nonetheless, it is acknowledged that the greedy selection approach primarily favors features with high-ranking scores, and thus, it may not adequately evaluate the potential of feature combinations that involve low-scoring elements. An extensive experimental inquiry was conducted across widely recognized sentiment analysis datasets to assess the performance of the introduced methodology. The computational findings eloquently demonstrate that the proposed algorithm attains an average accuracy of 96.88% for nine public sentiment datasets and 94.43% for the Humir datasets when coupled with the multinomial Naive Bayes classifier. Furthermore, the experimental outcomes conspicuously establish the superiority of the proposed technique in state-of-the-art studies across the same set of nine sentiment datasets and the Humir datasets.
更多查看译文
关键词
Sentiment classification,Multinomial Naive Bayes,Greedy search,Machine learning,Feature selection
AI 理解论文
溯源树
样例
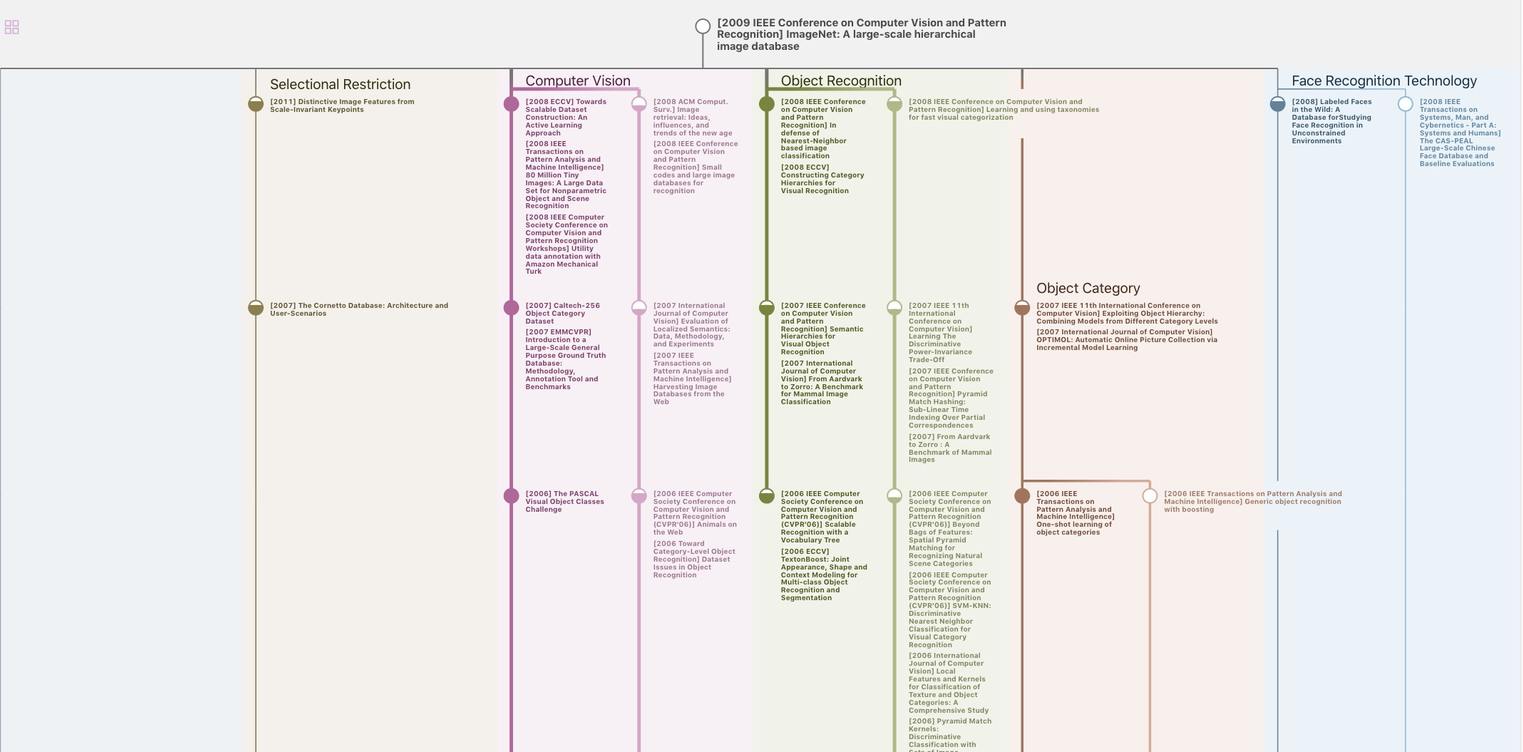
生成溯源树,研究论文发展脉络
Chat Paper
正在生成论文摘要