Assessment of artificial intelligence for predicting porosity of dehydrated food products
Computers and Electronics in Agriculture(2024)
Abstract
Porosity is a relevant characteristic of dried products, and it depends on several parameters such as food material properties, drying technologies, and process conditions. The multifaceted interactions between these parameters make the mathematical modeling of porosity a difficult task. In recent decades, Artificial Intelligence (AI) tools were found to be efficient in describing complex and non-linear problems in many fields. The aim of this contribution was assessing AI techniques to predict porosity of dried foods using a database comprising nine experimental input variables. In total, 511 combinations of these input variables were modeled with the extreme learning machine (ELM) technique. The input variables with the most impact on porosity prediction were highlighted after a systematic investigation, and the relevant combinations of input variables were selected. The product, technology, pretreatment, temperature, and pressure input variables were found to have the most influence on porosity predictions (ELM 5-inputs model: R=0.887, RMSE=0.102, AIC=-5.852, NSE=0.786). In addition to ELM technique, the support vector machine (SVM) and evolutionary polynomial regression (EPR) were also used for modeling the best combinations of input variables obtained with the ELM. The comparison between these three modeling techniques revealed that EPR provided practical models in terms of simplicity and accuracy (EPR 5-inputs model: correlation coefficient (R)=0.874, root mean square error (RMSE)=0.107, Akaike’s information criterion (AIC)=-206.555, Nash-Sutcliffe efficiency (NSE)=0.763), but SVM provided the most accurate model (SVM 9-inputs model: R=0.895, RMSE=0.099, AIC=2.927, NSE=0.797). The ELM model with 5 inputs, ranked as the second most accurate among all models was deemed practically favorable compared to others due to its minor statistical differences from the SVM model with 9 inputs, coupled with the advantage of featuring only 5 inputs instead of 9. External data was used to validate the models, and the predictions of porosity were in agreement with the experimental data. Creating a model that can effectively predict the porosity of dried foods across various drying scenarios is a significant challenge in porosity modeling. This study tackles this challenge by evaluating different AI techniques to develop the most versatile predictive model for porosity in dried foods. The developed models can be used as rapid and straightforward tools for predicting porosity of dehydrated products and therefore assist in designing and optimizing drying processes.
MoreTranslated text
Key words
Extreme learning machine,Evolutionary polynomial regression,Support vector regression,Modelling,Food drying,Food porous structure
AI Read Science
Must-Reading Tree
Example
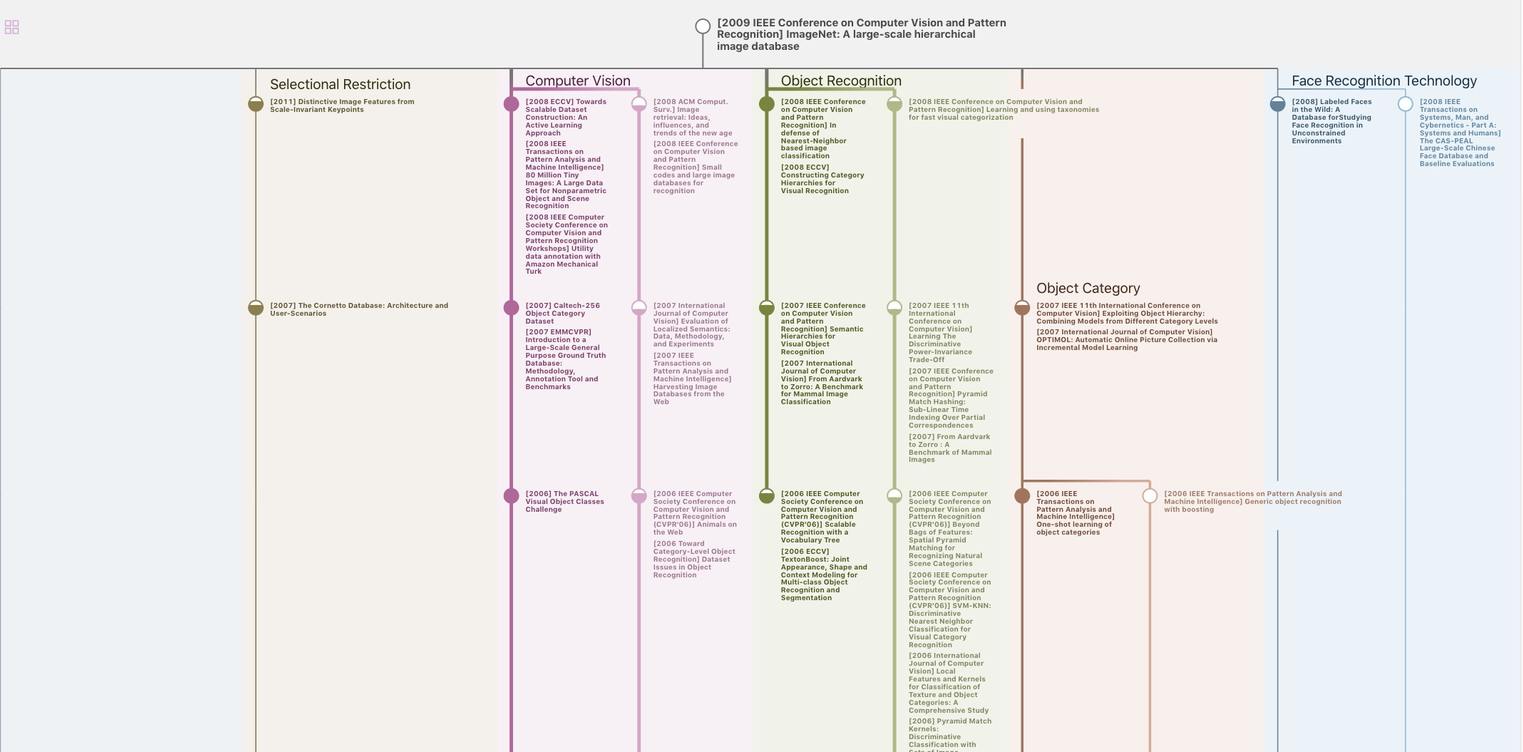
Generate MRT to find the research sequence of this paper
Chat Paper
Summary is being generated by the instructions you defined