A CNN-BiLSTM-Attention approach for EHA degradation prediction based on time-series generative adversarial network
Mechanical Systems and Signal Processing(2024)
摘要
As a representative integrated system for power-by-wire (PBW) systems, Electro-hydrostatic actuator (EHA) has series of advantages such as high power density, compactness, and high efficiency, which is one of the important development directions of future hydraulic system field. However, due to its high integration and high reliability requirements, it is challenging to conduct degradation studies in short period of time with limited data samples. For this type of high integrated mechatronics system, Prognostics and Health Management (PHM) is one of the key works to ensure its safety and reliability, especially the performance degradation prediction presented in this paper. To deal with the small size of EHA data, a time-based data enhancement method for expanding the performance data set is proposed based on Time Generative Adversarial Network (TimeGAN). Considering the complex of working state and system performance, the relationship between the EHA operation data and its health indicator is then analyzed using the CNN-BiLSTM-Attention model, so as to generate the health indicator combine with TimeGAN synthesis data. Finally, CNN-BiLSTM-Attention model with multi-input channels is developed, and EHA data as well as TimeGAN synthesized EHA data are incorporated into the model. The results show that this method can greatly improve the prediction accuracy of EHA performance, and provide a novel method for performance degradation prediction of integrated mechatronic system.
更多查看译文
关键词
TimeGAN,Electro-hydrostatic actuator,Data enhancement,CNN-BiLSTM-Attention,Prognostics health management (PHM)
AI 理解论文
溯源树
样例
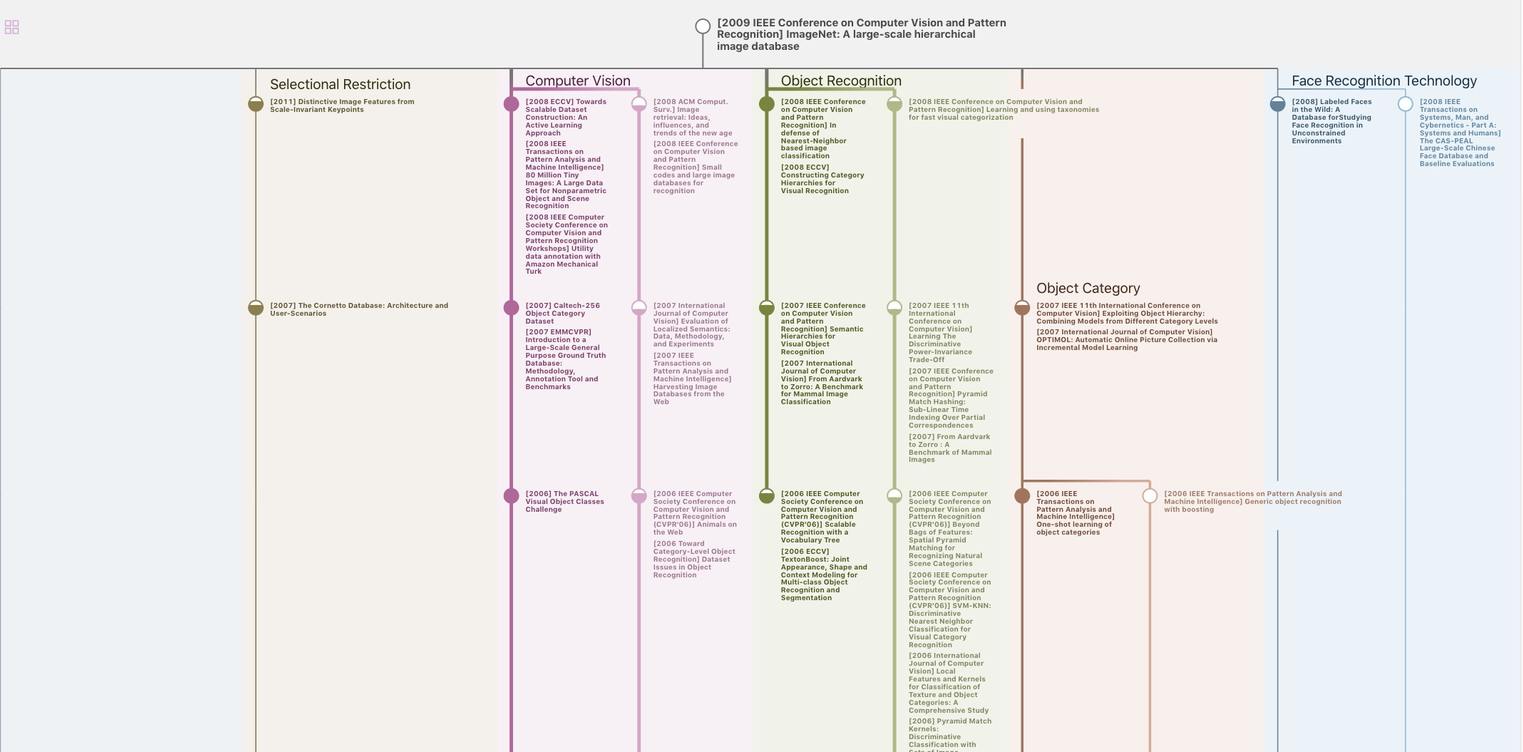
生成溯源树,研究论文发展脉络
Chat Paper
正在生成论文摘要