Significant wave height prediction in nested domains using radial basis function neural networks
Ocean Engineering(2024)
Abstract
This study presents a novel machine learning-statistics framework combining radial basis function neural networks (RBFNNs) with 2D geospatial interpolation for predicting the significant wave height (SWH) in nested fine resolution domains, while avoiding intensive computations. First, the wave model (WAM) is employed for generating data for ocean wave conditions in a dense and a coarse grid domain using high and low-resolution simulations. Then an RBFNN is trained using the fuzzy means algorithm to achieve highly accurate approximations of the SWH calculated by the high-resolution simulation at the common grid points of the two domains; the RBFNN uses as inputs information derived only from the low-resolution simulation, together with wind and bathymetry data. Having obtained accurate approximations at the common points, interpolation is employed for estimating SWH at the rest of the high-resolution grid. The proposed method performance is evaluated in two diverse case–study sites located in the Mediterranean Sea and a comparative analysis with alternative approaches is conducted. Results indicate that the proposed framework accurately reproduces WAM SWHs in dense nested regions, achieving coefficients of determination (R2) equal to 0.96 and 0.97 for each respective case study, while the computational time per forecast is on average equal to 50 μs.
MoreTranslated text
Key words
Neural networks,Numerical modelling,Radial basis function,WAM wave modelling,Wave height forecasting
AI Read Science
Must-Reading Tree
Example
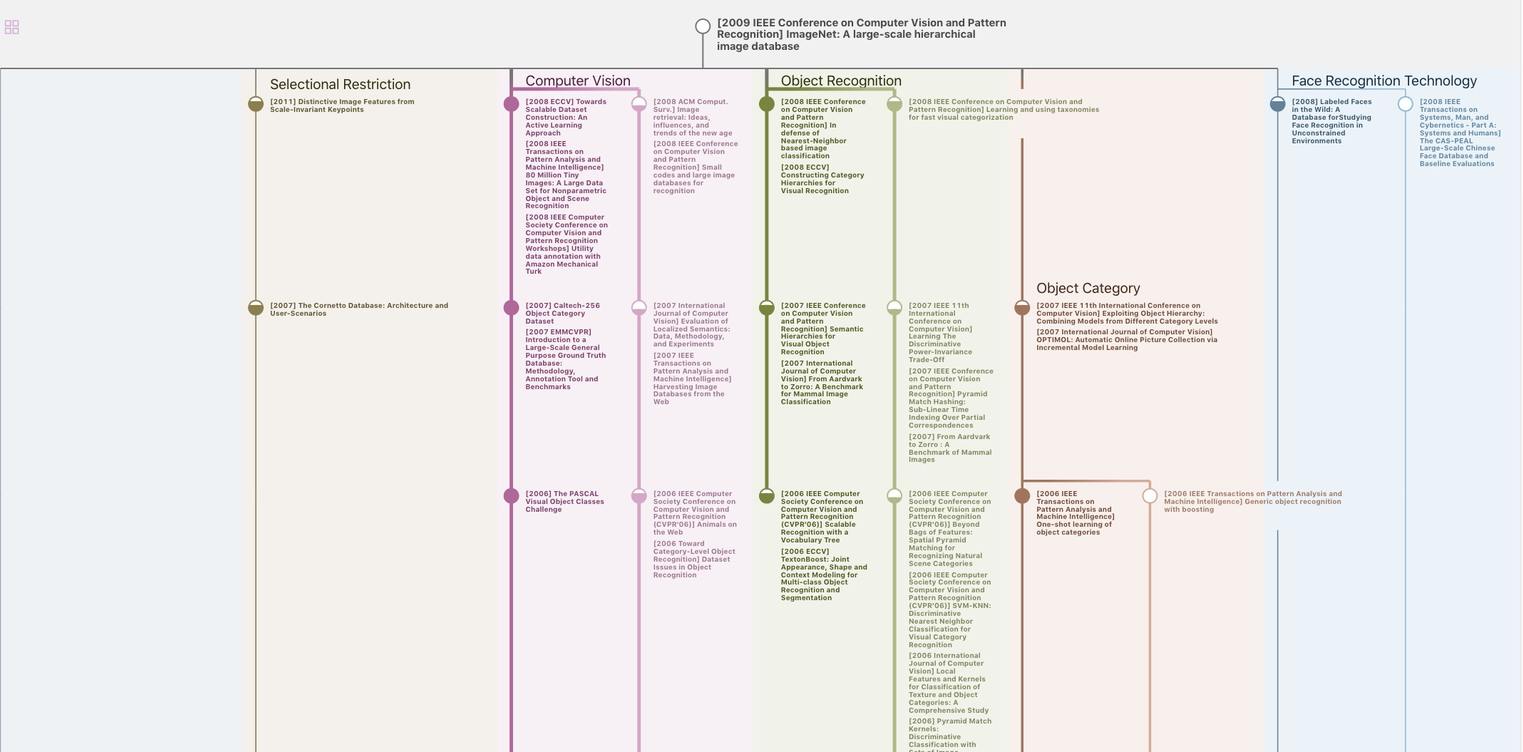
Generate MRT to find the research sequence of this paper
Chat Paper
Summary is being generated by the instructions you defined