Development and evaluation of two open-source nnU-Net models for automatic segmentation of lung tumors on PET and CT images with and without respiratory motion compensation
European Radiology(2024)
摘要
In lung cancer, one of the main limitations for the optimal integration of the biological and anatomical information derived from Positron Emission Tomography (PET) and Computed Tomography (CT) is the time and expertise required for the evaluation of the different respiratory phases. In this study, we present two open-source models able to automatically segment lung tumors on PET and CT, with and without motion compensation. This study involved time-bin gated (4D) and non-gated (3D) PET/CT images from two prospective lung cancer cohorts (Trials 108237 and 108472) and one retrospective. For model construction, the ground truth (GT) was defined by consensus of two experts, and the nnU-Net with 5-fold cross-validation was applied to 560 4D-images for PET and 100 3D-images for CT. The test sets included 270 4D- images and 19 3D-images for PET and 80 4D-images and 27 3D-images for CT, recruited at 10 different centres. In the performance evaluation with the multicentre test sets, the Dice Similarity Coefficients (DSC) obtained for our PET model were DSC(4D-PET) = 0.74 ± 0.06, improving 19
更多查看译文
关键词
Lung cancer,Positron emission tomography,Computed tomography,Deep learning,Respiratory motion
AI 理解论文
溯源树
样例
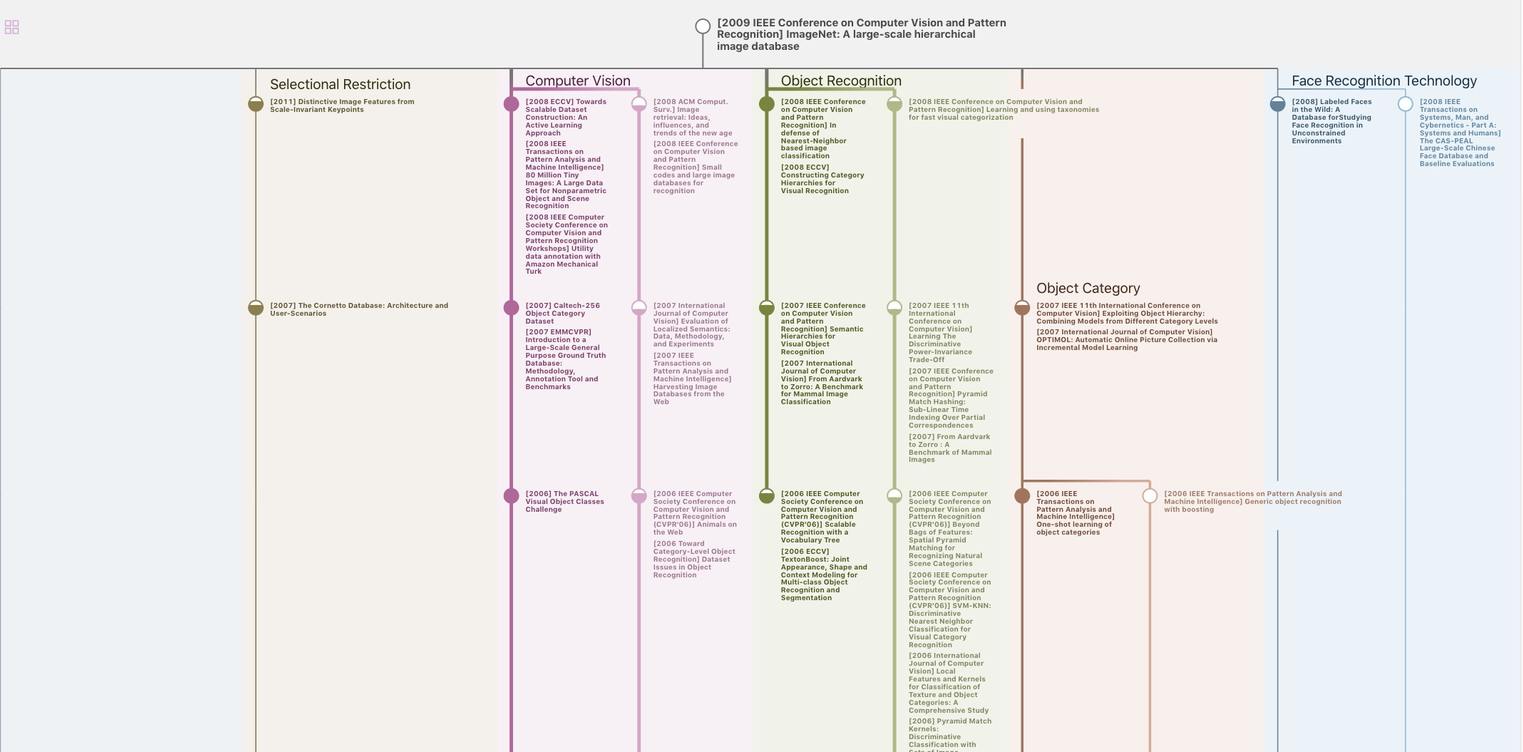
生成溯源树,研究论文发展脉络
Chat Paper
正在生成论文摘要