Bayesian data-driven framework for structural health monitoring of composite structures under limited experimental data
STRUCTURAL HEALTH MONITORING-AN INTERNATIONAL JOURNAL(2024)
摘要
Ultrasonic-guided waves can be used to monitor the health of thin-walled structures. However, the run of experimental damage tests on materials like carbon fiber-reinforced plastics can be impractical and costly. Instead, numerical models can be used to create hybrid datasets to train machine learning algorithms, integrating data from numerical and experimental tests. This paper presents a Bayesian-driven framework to compensate for limited experimental data regarding Lamb wave propagation in composite plates. Using Bayesian inference, the framework updates a numerical finite element model, considering observed uncertainties by sampling posterior probability density functions for input parameters using Markov-Chain Monte Carlo simulations with the Metropolis-Hastings algorithm. A neural network surrogate model speeds-up these simulations, leading to a model that replicates the uncertain experimental setup. This model then generates data to augment true experimental data. Finally, a one-dimensional convolutional neural network is trained on a three different datasets to analyze Lamb wave signals and assess damage. Comparing training strategies shows the hybrid dataset augmented by samples generated by the updated FE model gives the most accurate damage size predictions.
更多查看译文
关键词
Structural health monitoring,composite materials,Bayesian calibration,machine learning,convolutional neural networks
AI 理解论文
溯源树
样例
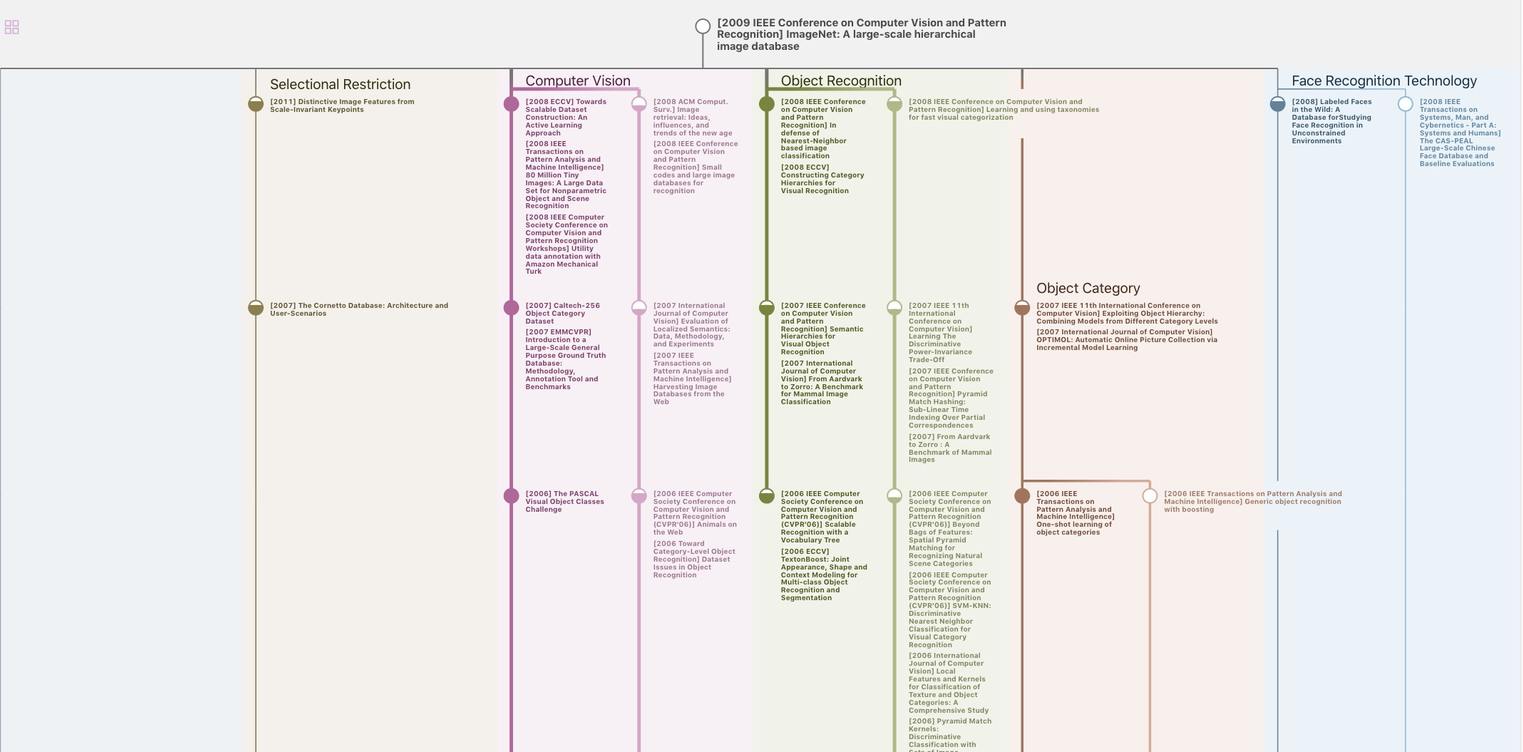
生成溯源树,研究论文发展脉络
Chat Paper
正在生成论文摘要