Dynamic fault detection and diagnosis for alkaline water electrolyzer with variational Bayesian Sparse principal component analysis
arxiv(2024)
摘要
Electrolytic hydrogen production serves as not only a vital source of green
hydrogen but also a key strategy for addressing renewable energy consumption
challenges. For the safe production of hydrogen through alkaline water
electrolyzer (AWE), dependable process monitoring technology is essential.
However, random noise can easily contaminate the AWE process data collected in
industrial settings, presenting new challenges for monitoring methods. In this
study, we develop the variational Bayesian sparse principal component analysis
(VBSPCA) method for process monitoring. VBSPCA methods based on Gaussian prior
and Laplace prior are derived to obtain the sparsity of the projection matrix,
which corresponds to ℓ_2 regularization and ℓ_1 regularization,
respectively. The correlation of dynamic latent variables is then analyzed by
sparse autoregression and fault variables are diagnosed by fault
reconstruction. The effectiveness of the method is verified by an industrial
hydrogen production process, and the test results demonstrated that both
Gaussian prior and Laplace prior based VBSPCA can effectively detect and
diagnose critical faults in AWEs.
更多查看译文
AI 理解论文
溯源树
样例
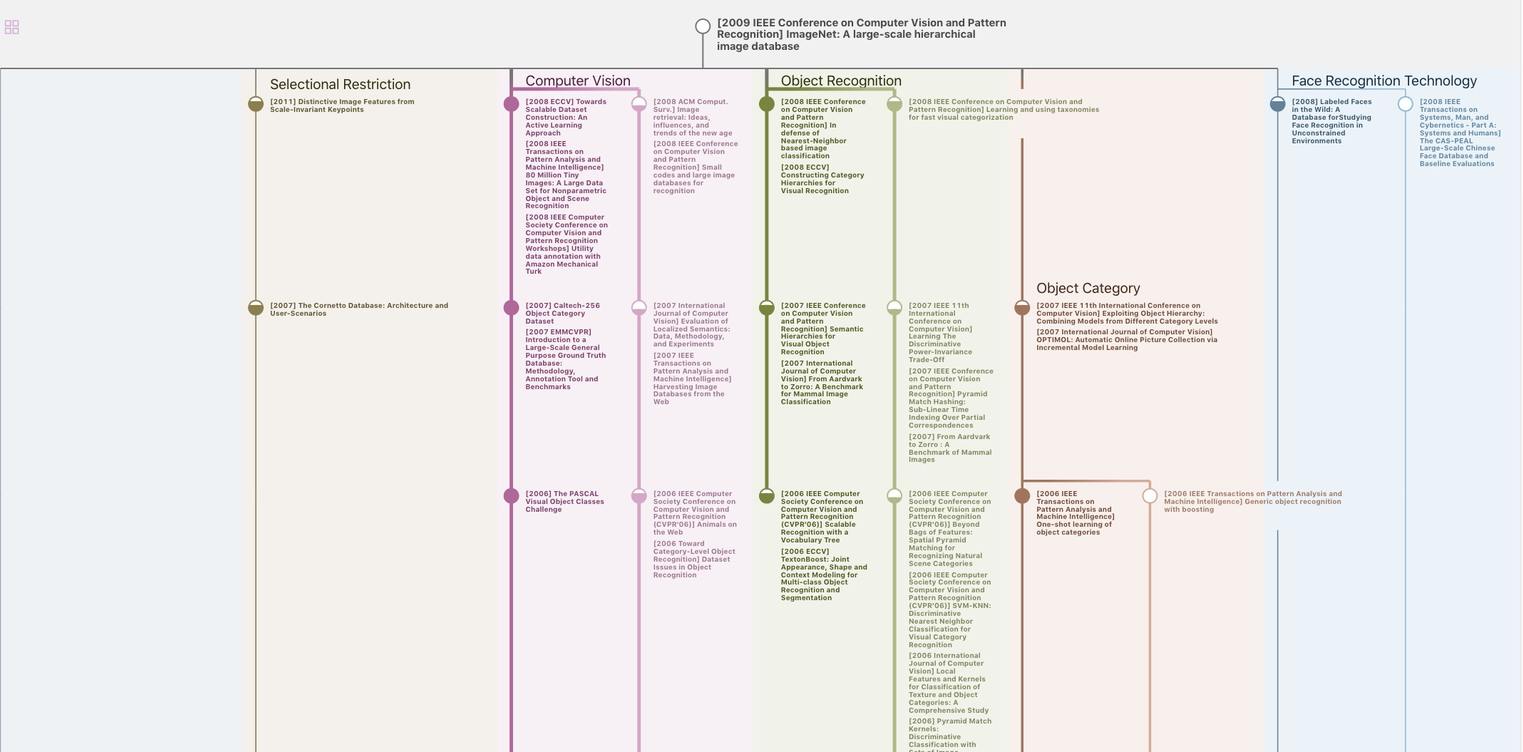
生成溯源树,研究论文发展脉络
Chat Paper
正在生成论文摘要