Deep reinforcement learning aided secure UAV communications in the presence of moving eavesdroppers
Journal of King Saud University - Computer and Information Sciences(2024)
摘要
Integrating unmanned aerial vehicles(UAVs) with wireless systems is widely anticipated to bring enormous benefits to network users, and is considered as a key enabling technology for next generation wireless networks. However deployment of UAVs raises security concerns, as they are prone to eavesdropping attacks. In this paper we investigate secure data transmissions in a UAV-aided communication system from the physical layer security(PLS) perspective. The UAV is employed as a flying base station and transmit confidential information to a ground user. Specifically, an eavesdropper is moving in the vicinity of the ground user, attempting to intercept legitimate data transmission. We aim to optimize UAV trajectory to maximize secrecy throughput and due to the fast changing environment with unpredictable movements of the eave, this nonconvex problem is difficult to solve. Instead, we propose a deep reinforcement learning framework by reformulating this problem as a Markov Decision Process(MDP). By carefully designing state spaces,state-dependent action sets and time-dependant reward signals, we aim to help the agent learn an optimized trajectory and complete the flight task within time limit. We adapt the deep Q network(DQN) algorithm and extend it with prioritized experience replay(PER) and importance sampling method to ensure a stable and effective learning outcome. Simulation results validate the effectiveness of the learning algorithm and confirm that the agent is able to learn an effective policy with desirable and robust learning outcomes. Compared with benchmark schemes, our proposed scheme is shown to achieve considerable performance gain in secrecy throughput.
更多查看译文
关键词
Unmanned aerial vehicle,Physical layer security,Deep reinforcement learning,Prioritized experience replay
AI 理解论文
溯源树
样例
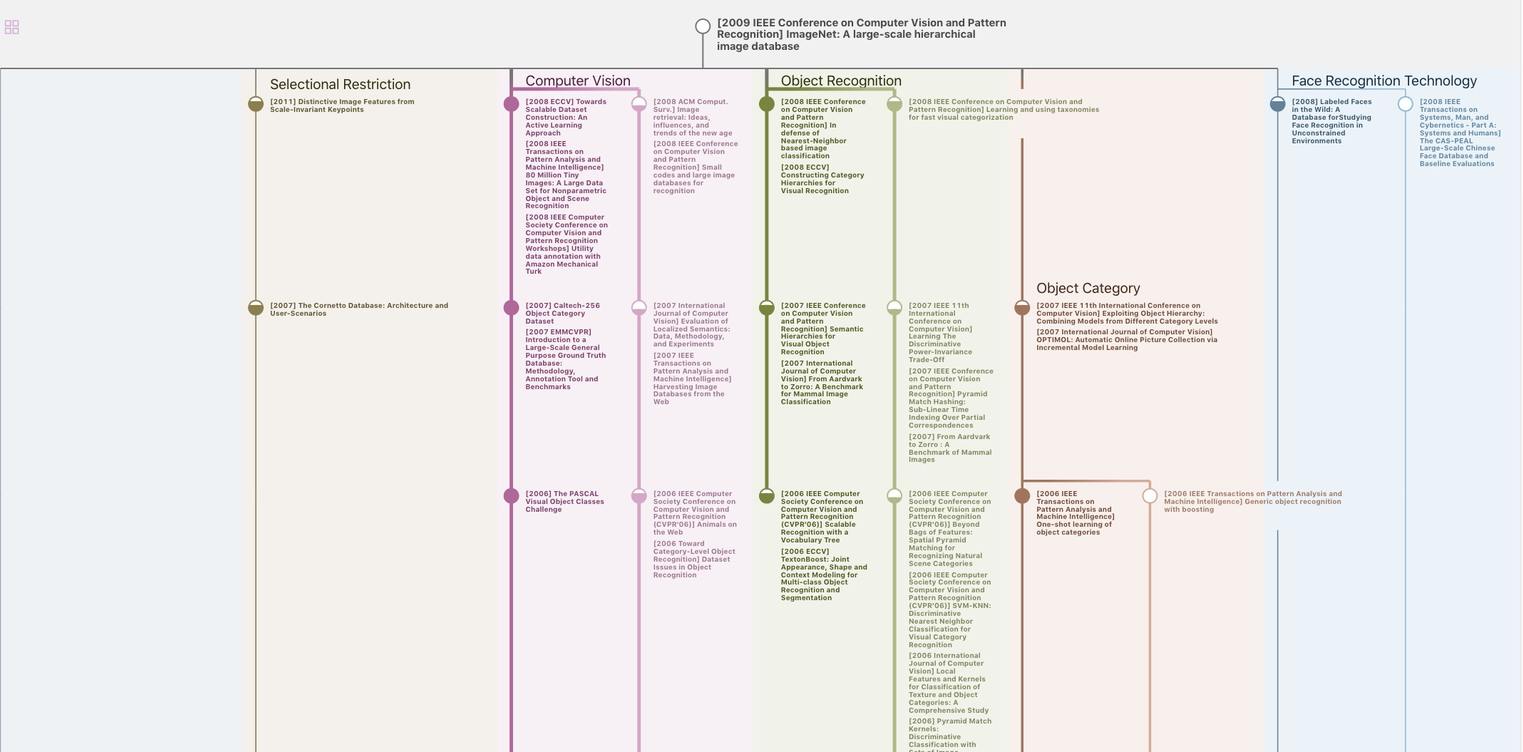
生成溯源树,研究论文发展脉络
Chat Paper
正在生成论文摘要