Predictive Modelling for Heating and Cooling Load Systems of Residential Building
2024 IEEE International Conference on Interdisciplinary Approaches in Technology and Management for Social Innovation (IATMSI)(2024)
Abstract
This paper review various machine learning models to predict the energy efficiency of residential buildings. For this, a dataset comprising eight independent factors namely, surface area, relative compactness, wall area, overall height, roof area, glazing area, orientation, and glazing area distribution, along with two dependent variables as Heating Load (HL) and Cooling Load (CL) are provided. The dataset has 768 samples, and is divided as 70% training and 30% testing of models. Using mean absolute error (MAE), coefficient of determination (R
2
), and root mean square error (RMSE) the accuracies of the models are evaluated. Based on results found using the above evaluation, an overall efficient model, The Extra Tree Regressor model shows better accuracy as compared to other energy prediction model for HL and CL. Further various other aspects are also plotted such as the overall performance of the model, effect and correlation of input variables and output etc.
MoreTranslated text
Key words
Heating load (HL),cooling load (CL),forecasting,machine learning,regression
AI Read Science
Must-Reading Tree
Example
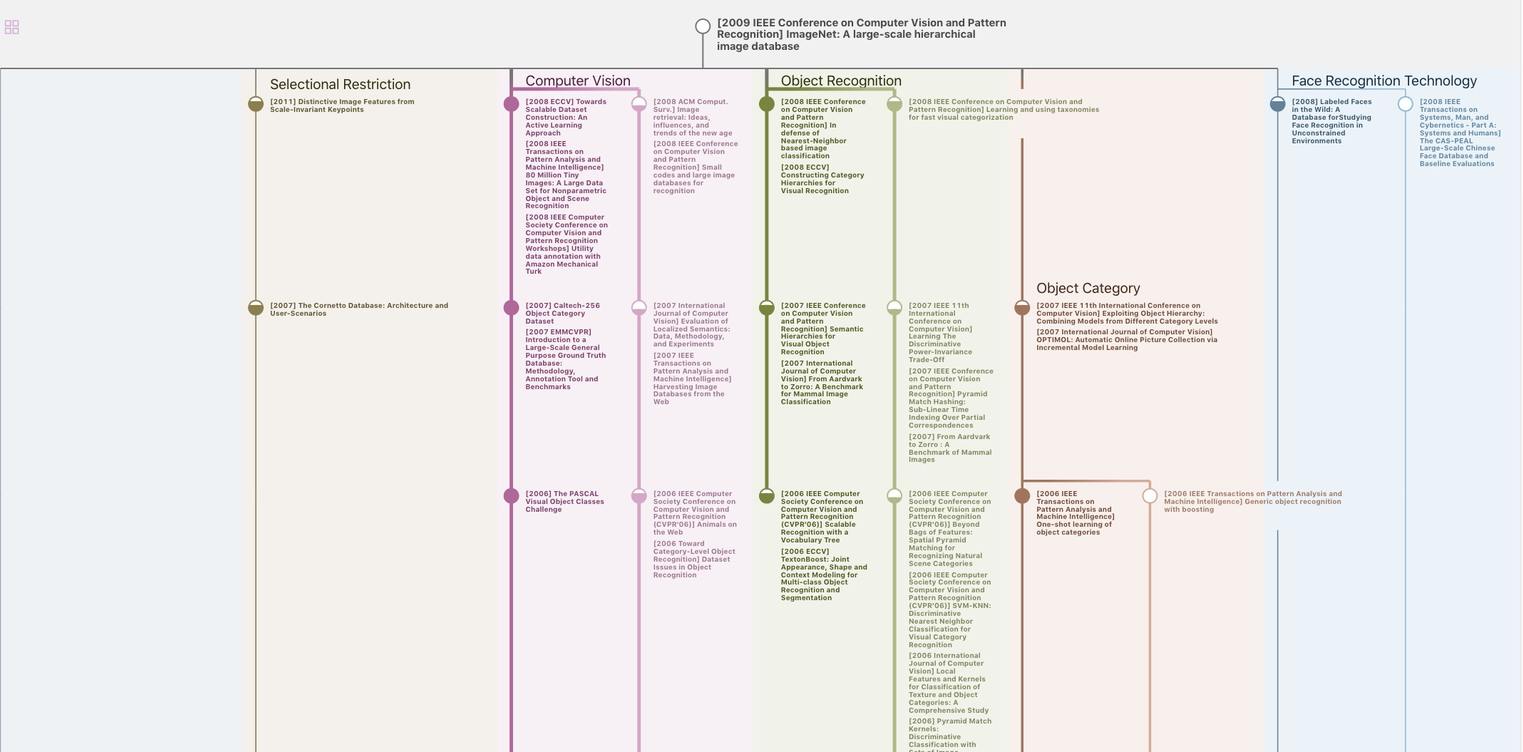
Generate MRT to find the research sequence of this paper
Chat Paper
Summary is being generated by the instructions you defined