Extractor-attention-predictor network for quantitative photoacoustic tomography
Photoacoustics(2024)
Abstract
Quantitative photoacoustic tomography (qPAT) holds great potential in estimating chromophore concentrations, whereas the involved optical inverse problem, aiming to recover absorption coefficient distributions from photoacoustic images, remains challenging. To address this problem, we propose an extractor-attention-predictor network architecture (EAPNet), which employs a contracting-expanding structure to capture contextual information alongside a multilayer perceptron to enhance nonlinear modeling capability. A spatial attention module is introduced to facilitate the utilization of important information. We also use a balanced loss function to prevent network parameter updates from being biased towards specific regions. Our method obtains satisfactory quantitative metrics in simulated and real-world validations. Moreover, it demonstrates superior robustness to target properties and yields reliable results for targets with small size, deep location, or relatively low absorption intensity, indicating its broader applicability. The EAPNet, compared to the conventional UNet, exhibits improved efficiency, which significantly enhances performance while maintaining similar network size and computational complexity.
MoreTranslated text
Key words
Quantitative photoacoustic tomography,Optical inverse problem,Deep learning,Image reconstruction,Absorption coefficient estimation
AI Read Science
Must-Reading Tree
Example
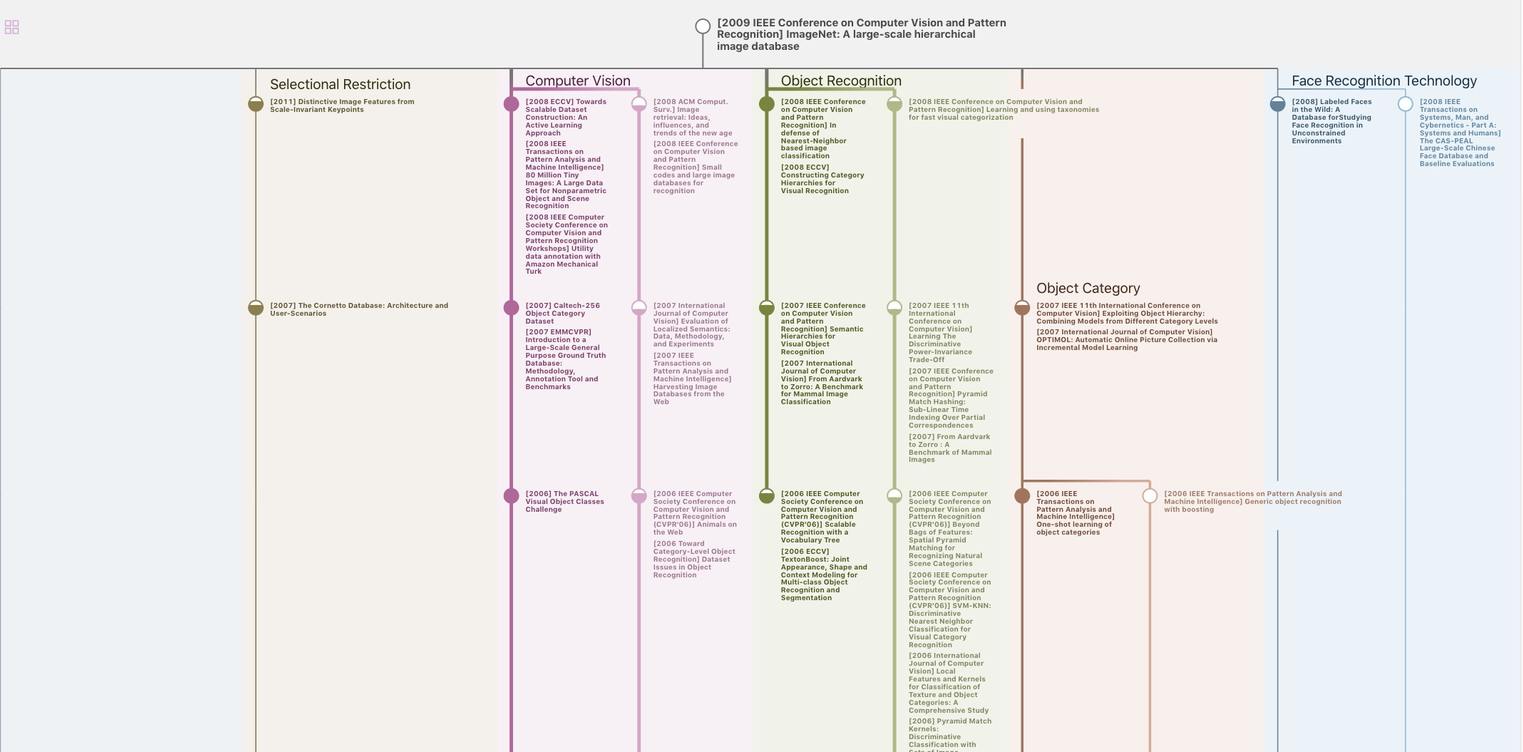
Generate MRT to find the research sequence of this paper
Chat Paper
Summary is being generated by the instructions you defined