Scope-Free Global Multi-Condition-Aware Industrial Missing Data Imputation Framework via Diffusion Transformer
IEEE Transactions on Knowledge and Data Engineering(2024)
摘要
Missing data is a common phenomenon in the industrial field. The recovery of missing data is crucial to enhance the reliability of subsequent data-driven monitoring and control of industrial processes. Most existing methods are limited by the confined scope of feature extraction, which makes it impossible to rely on global information to impute missing data. In addition, they usually assume that industrial data is a uniform distribution across all working conditions, ignoring the differences in data evolution patterns across different conditions. To address these issues, this paper proposes an innovative scope-free global multi-condition-aware imputation framework based on diffusion transformer (SGMCAI-DiT). First, it extends the diffusion model by introducing conditional probability to capture the condition distribution of the entire data. Then, a noise prediction model is designed based on a novel double-weighted attention mechanism (DW-SA) to broaden the horizons of feature extraction. By discerning the inter-conditional interactions and the intra-conditional local information, the missing data imputation performance can be improved. Finally, the effectiveness and suitability of the proposed SGMCAI-DiT are verified on four real datasets sourced from industrial processes and two public non-industrial datasets. Extensive experimental results demonstrate that the proposed method outperforms several state-of-the-art methods in different missing data scenarios.
更多查看译文
关键词
Missing data imputation,scope-free global multi-condition-aware imputation framework,diffusion model,transformer
AI 理解论文
溯源树
样例
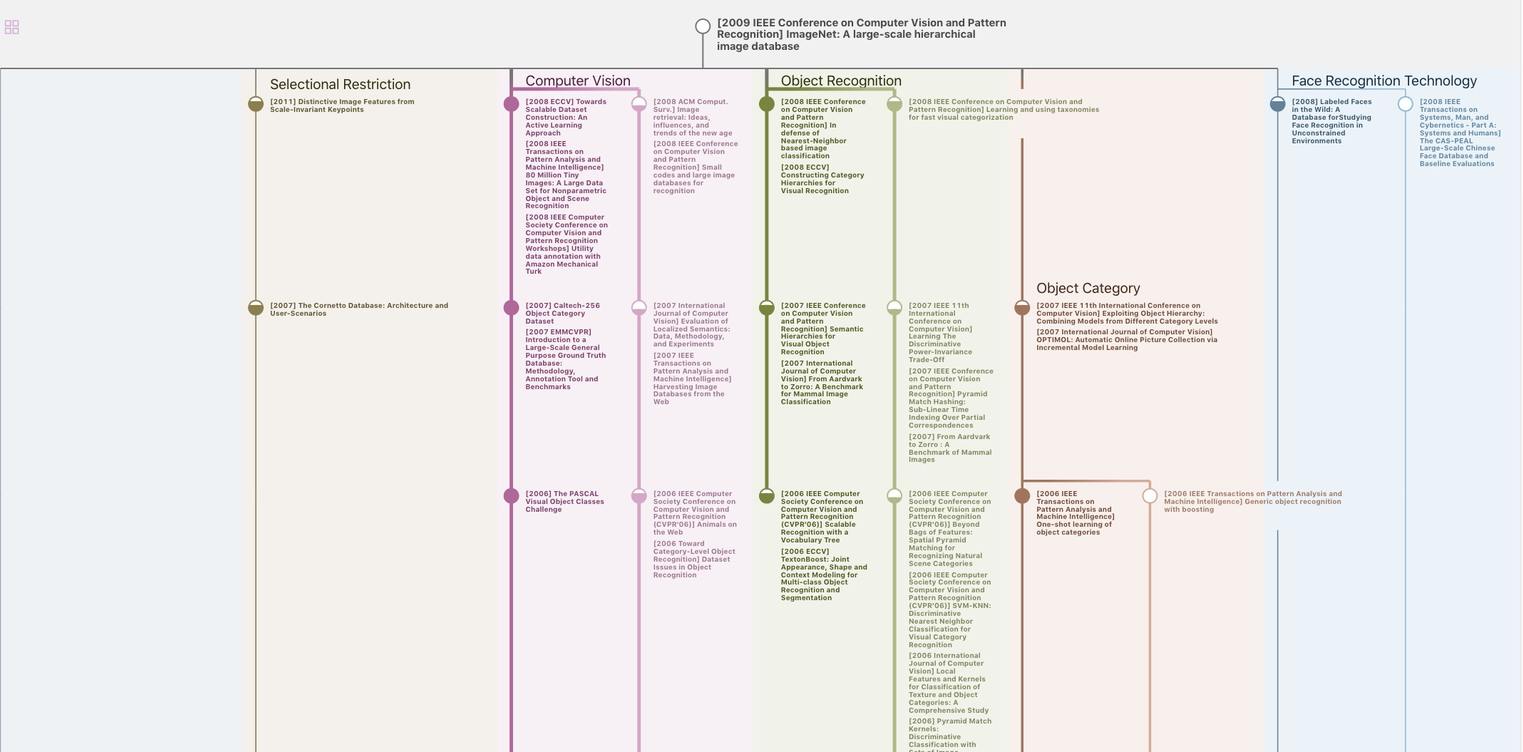
生成溯源树,研究论文发展脉络
Chat Paper
正在生成论文摘要