Sinbad Origins Of Contextually-Guided Feature Learning: Self-Supervision With Local Context For Target Detection
SoutheastCon 2024(2024)
Abstract
The domain of feature learning is replete with self-supervised methodologies that harness local context to decode complex patterns in data. This paper investigates the significance of the SINBAD model within the realm of self-supervised learning. SINBAD, a unique non-convolutional neural network approach, is particularly adept at extracting mutual information and identifying distinct local structures. The underlying theory of SINBAD, anchored in neuroscience, suggests that capturing predictable connections among subsets of features can significantly enhance feature extraction. This principle has been instrumental in the advancement of self-supervised learning as it has given rise to the development of its convolutional extensions that are self-guided by contextual understanding. In this paper, we explore the application of the SINBAD algorithm for automatic target recognition, specifically in detecting military vehicles within complex rural environments. Our methodology includes a preprocessing stage utilizing a linear Support Vector Machine (SVM) to learn and apply a filter. This filter is crucial for excluding image locations that are completely irrelevant, thereby isolating potentially relevant regions for contextual self-supervision. By focusing on these selected areas and avoiding the need to process arbitrary regions, we aim to minimize resource requirements and streamline the self-supervised learning process. In the subsequent phase of our approach, the focus shifts to identifying nonlinear features that provide insights into local contexts. This involves extracting correlated nonlinear functions from nearby, yet distinct and non-overlapping patches. By analyzing these functions, we can discern patterns and relationships within the local context, where each function correlates with its unique, adjacent patch. This sophisticated analysis allows for a deeper understanding of the local area, contributing significantly to the accuracy of the final classification step. Finally, the process culminates with the use of a linear classifier that utilize the SINBAD features to effectively identify and categorize image patches that contain the intended targets. The research was conducted using the TNO-TM Search_2 dataset, which comprises 44 high-resolution images. These images depict cluttered rural landscapes and feature 9 different types of military vehicles. The dataset's complexity and variety provide a comprehensive environment for testing and validating the effectiveness of the SINBAD model in accurately identifying and classifying these vehicles within such challenging and diverse scenes.
MoreTranslated text
AI Read Science
Must-Reading Tree
Example
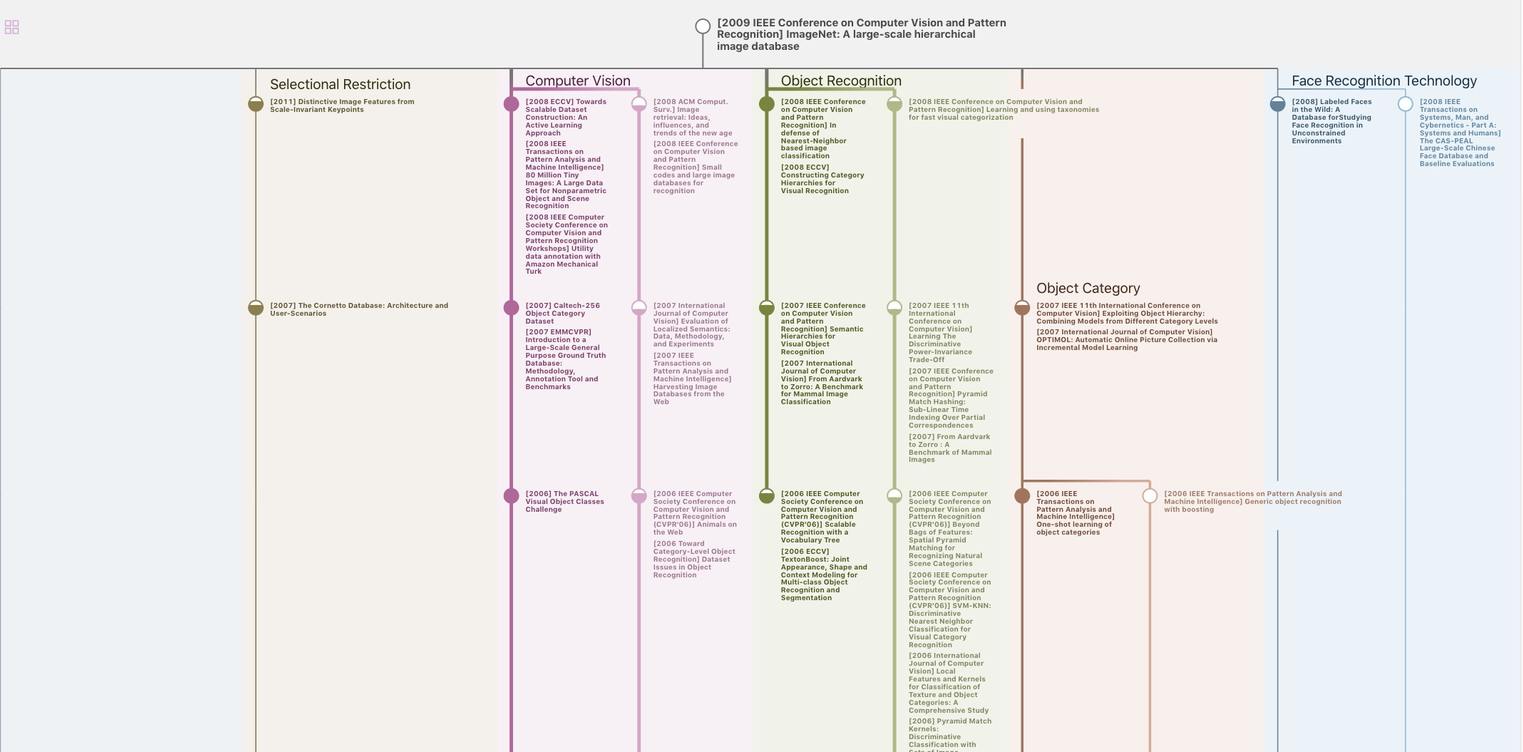
Generate MRT to find the research sequence of this paper
Chat Paper
Summary is being generated by the instructions you defined