An Efficient and Interpretable Stacked Model for Wind Speed Estimation Based on Ensemble Learning Algorithms
ENERGY TECHNOLOGY(2024)
摘要
Wind energy has gained tremendous attention recently due to its abundance potential and to obviate the adverse impacts of fossil fuel consumption. However, the efficient development and operation of wind energy systems require precise and accurate wind speed prediction. To fulfill this aim, machine learning algorithms have shown its prowess in accurately predicting the wind speed and this study proposes an interpretable stacked ensemble with ridge regressor utilizing ML algorithms such as catboost (CB), gradient boost (GB), multilayer perceptron (MLP), and random forest (RF) as a base model. The base models' estimations are integrated by applying a ridge regressor to enhance the accuracy of wind speed estimation. The dataset consists of 87 600 samples from which 80% samples are used for training. The proposed approach provides interpretability of the prediction results through Shapley additive explanations and feature importance analysis. The result of the proposed method is calculated by comparing the estimation outcomes with each model such as CB, GB, MLP, and RF in terms of error metrics such as root mean squared error (RMSE) and mean absolute error (MAE). The proposed model outperforms the others due to a reduction of error in RMSE by 19.89% and MAE by 24% respectively. For wind speed prediction, this study presents a stacking ensemble method that outperforms other machine learning (ML) techniques with an R2 value of 0.970. The interpretable stacked ensemble with ridge regressor model pinpoints important variables such as surface pressure, direction, and hourly wind speed ranges and emphasises the significance of explainable ML algorithms for future research to address complicated variables in renewable energy applications.image (c) 2024 WILEY-VCH GmbH
更多查看译文
关键词
ensemble learning,estimations,machine learning,stacking,wind speeds
AI 理解论文
溯源树
样例
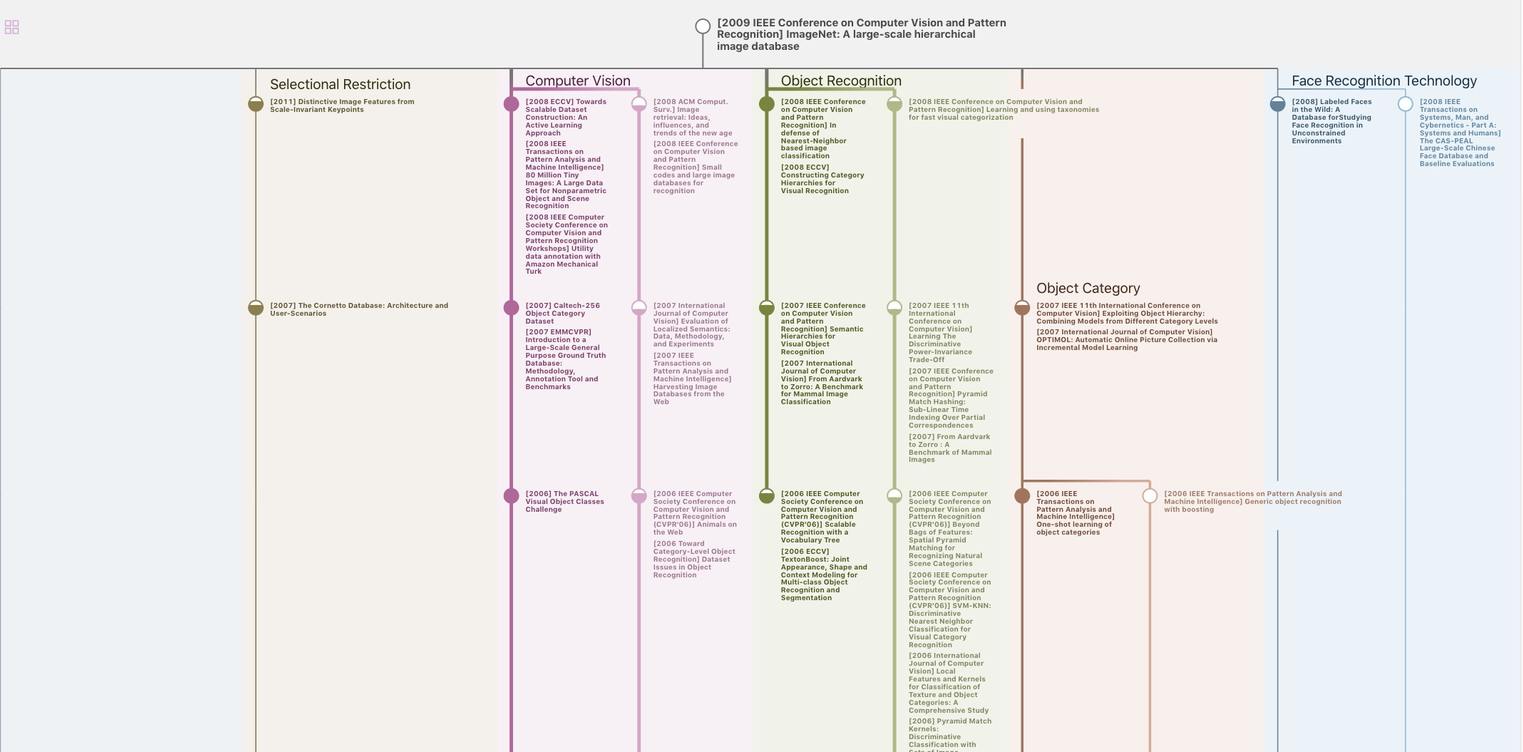
生成溯源树,研究论文发展脉络
Chat Paper
正在生成论文摘要