An Integrated GoogleNet with Convolutional Neural Networks Model for Multiclass Bean Leaf Lesion Detection
2024 IEEE International Conference on Interdisciplinary Approaches in Technology and Management for Social Innovation (IATMSI)(2024)
摘要
The bean leaves have very complicated structures, therefore these diseases are able to infect not only upper surfaces, but also lower ones. In the initial stages it is rather difficult to precisely establish the alterations of leaf lesions as they are characterized with a complicated structure. These include many other pathogens like fungi and viruses which may instigate disease on bean leaves. A number of pathogens may manifest as a variety of symptoms that are not necessarily associated with each other, making it tougher to determine which bacterium causes them. It helps in controlling the health and yields of crops by monitoring on leaf diseases of the beans. A robust multiclass detection approach using an ensemble of GoogleNet and CNN models is presented by this study for bean bean leaf disease. It has greater precision and generalization because it leverages CNN’s hierarchical feature learning and Google Net’s creative inception modules. The system is trained on a variety of data sets including normal, angular leaf spot, and bean rust in different classes. The model's performance is further optimized through the incorporation of transfer learning. The effectiveness of the ensemble approach is demonstrated by experimental results in infection classification, which present a promising way forward for the timely control of diseases and precision agriculture in bean crops. The performance of the proposed ensemble model has been identified in terms of training loss, training accuracy, precision, recall, F1-score, testing loss, and testing accuracy, while the learning rates have been set to 0.05, and batch size of 32. The highest classification accuracy of 98.93% and lowest loss of 0.0043 have been identified at epoch 60, whereas, this accuracy was 84.11% and loss of 0.8707 at epoch 10. This model has been further compared with the existing models resulting it in the outperforming results by achieving higher accuracy.
更多查看译文
关键词
Bean leaf disease,GoogleNet,convolutional neural networks,angular leaf spot,bean rust
AI 理解论文
溯源树
样例
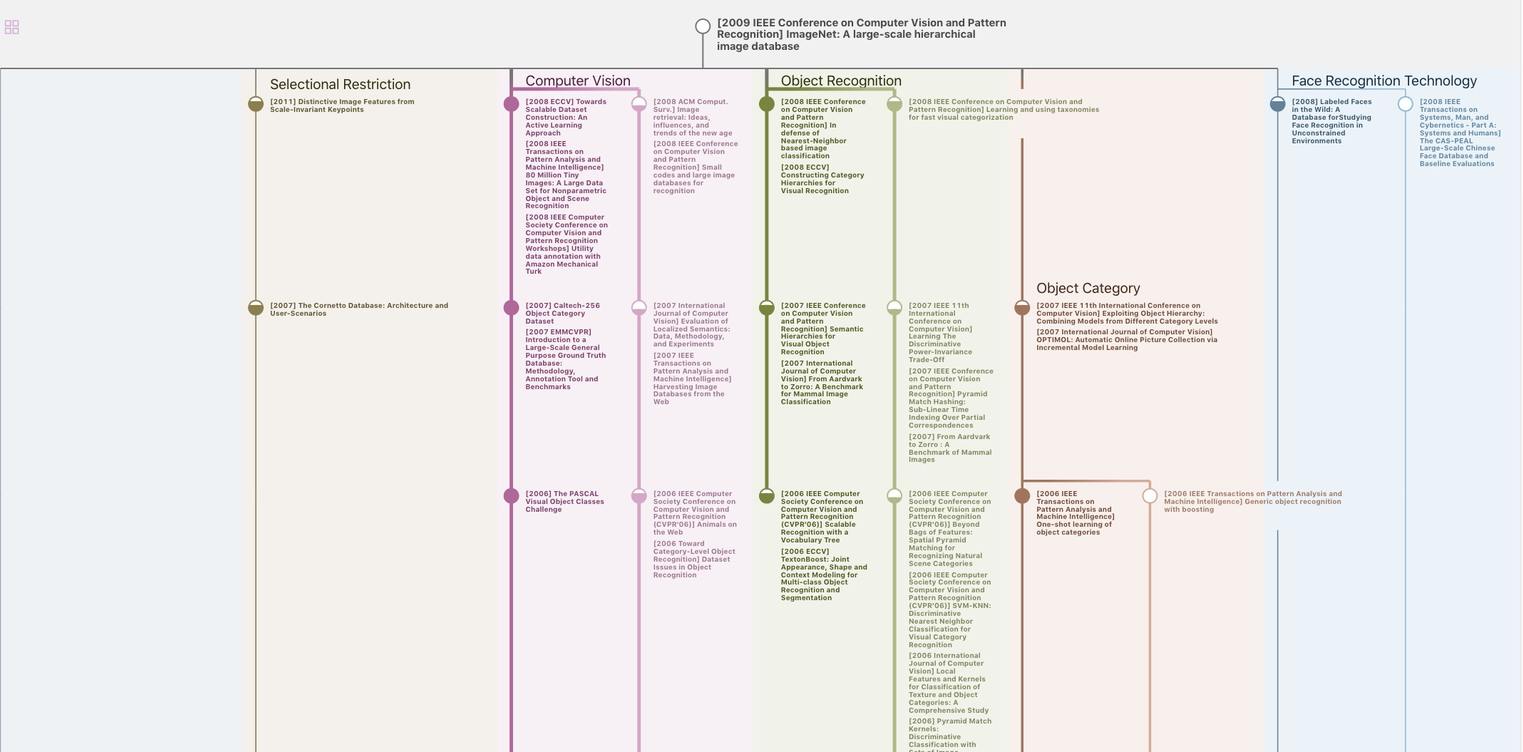
生成溯源树,研究论文发展脉络
Chat Paper
正在生成论文摘要