Tea Leaf Disease Detection: Federated Learning CNN Used for Accurate Severity Analysis
2024 IEEE International Conference on Interdisciplinary Approaches in Technology and Management for Social Innovation (IATMSI)(2024)
摘要
The purpose of this research paper is to investigate an innovative application of federated learning with convolutional neural networks (CNN) for the classification of tea leaf diseases. Based on aggregating data from four clients, we depict a variety of disease appearances based on four distinct degrees of severity. We use federated learning to transform local data insights into a comprehensive global model that protects user privacy while also performing stably across different datasets. We collected local client data insights using a federated learning framework and a federated averaging algorithm in the implementation of our scheme. The choice of methodology was imperative to achieving high accuracy in disease classification without compromising client information. In the result analysis, notable performance metrics were found across clients. Measures as specific as precision, recall, F1-score support, and accuracy were taken into account when calculating the model's effective disease identification. In order to gain a deeper understanding of the performance characteristics of the model across different classes or instances, we analyzed macro, micro, and weighted averages on local data. There was an equal distribution of macro averages among all classes. Macro averages ranged from 90.19% in DF_1 to 93.24% in DF_5. Based on the class support weighted average, df_5 came in first at 93.24 per cent. All classes contributed to the microaverage as well. Df_5 scored the highest average with 93.23 per cent. The averages indicate that the model can handle different class distributions and instance counts. To summarize, our research provides a strong argument for the use of federated learning with CNN in agriculture, specifically to classify diseases of tea leaves.
更多查看译文
关键词
Tea leaf diseases,Convolutional neural networks (CNNs),Federated learning,Disease classification,Severity levels
AI 理解论文
溯源树
样例
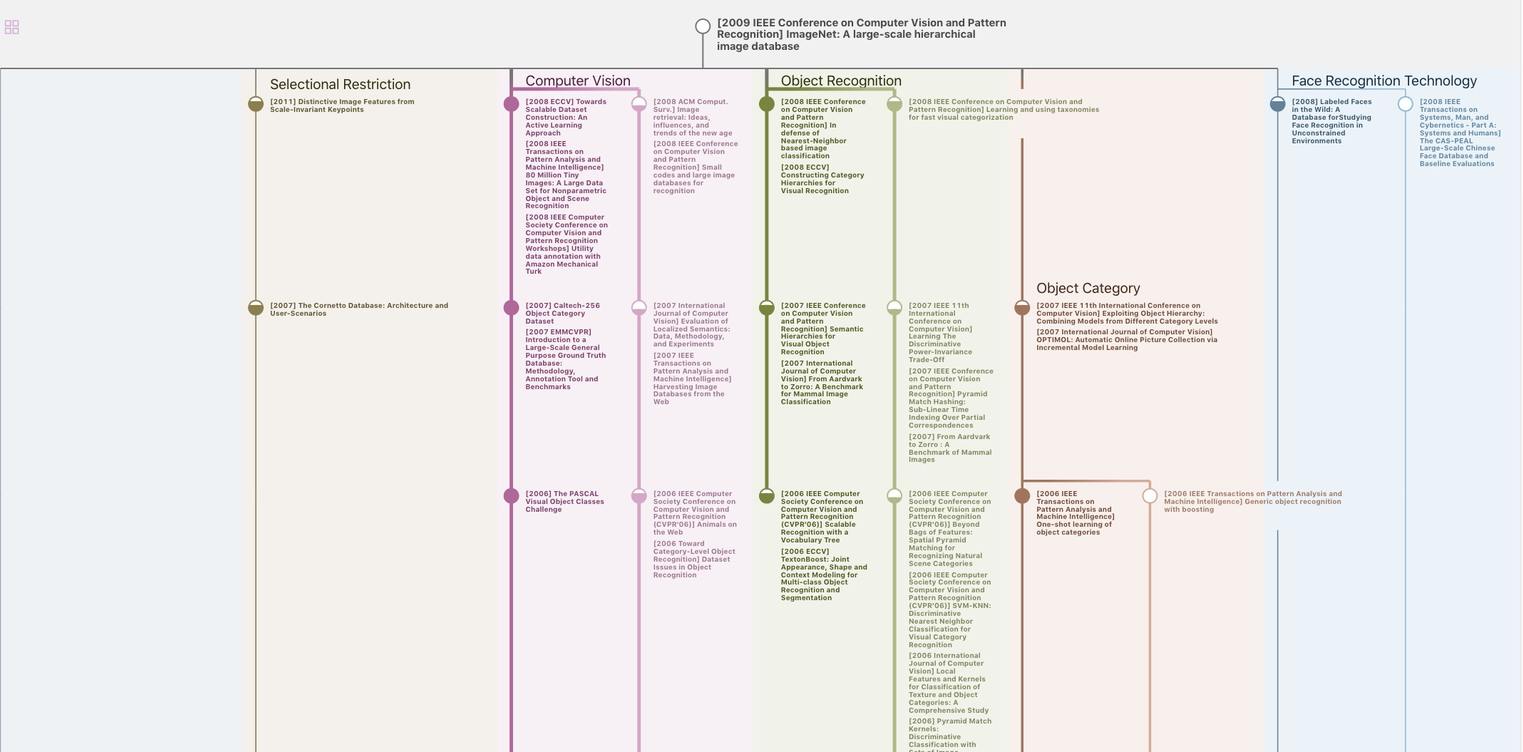
生成溯源树,研究论文发展脉络
Chat Paper
正在生成论文摘要