A hybrid multi-objective approach for time series forecasting considering peak instants
Energy Reports(2024)
摘要
Smart grids are essential for planning and managing energy systems as a promising alternative for energy efficiency, generating economic and environmental benefits. In this field, forecasting systems have been the subject of several studies and prove to be a challenge, given the volatility of data and its dependence on external factors. One open issue in time series forecasting is the definition of the best set of hyperparameters of the used techniques. In most studies, this problem has been treated as a single objective, in which the general error of the forecast is used as the only objective function. However, the error in peak instants can also be considered, making the problem multi-objective. In the present paper, a method is proposed aiming to minimize both errors through a hybrid multi-objective nonlinear combination approach based on Support Vector Regression (SVR), Autoregressive Integrated Moving Average (ARIMA), and Non-dominated Sorting Genetic Algorithm II (NSGA-II), called MHSys (Multi-objective Hybrid System). This system increases the exploration of search space solutions through a Unified Pareto Front, which is a combination of the Pareto front of all NSGA-II non-dominated solutions. The MHSys returns a set of different Pareto optimal solutions, whose final solution can be chosen by the expert, weighting the priority objective. The proposed system reaches superior performance in comparison to previous literature approaches. The results demonstrate that choosing a solution among a set of optimal solutions, according to the priority of each objective, improves the system’s accuracy. Furthermore, according to the results, the Unified Pareto Front presents a greater coverage of possible solutions when compared to Individual Fronts.
更多查看译文
关键词
Time series forecasting,Hybrid system,Multi-objective optimization,SVR,ARIMA,NSGA-II
AI 理解论文
溯源树
样例
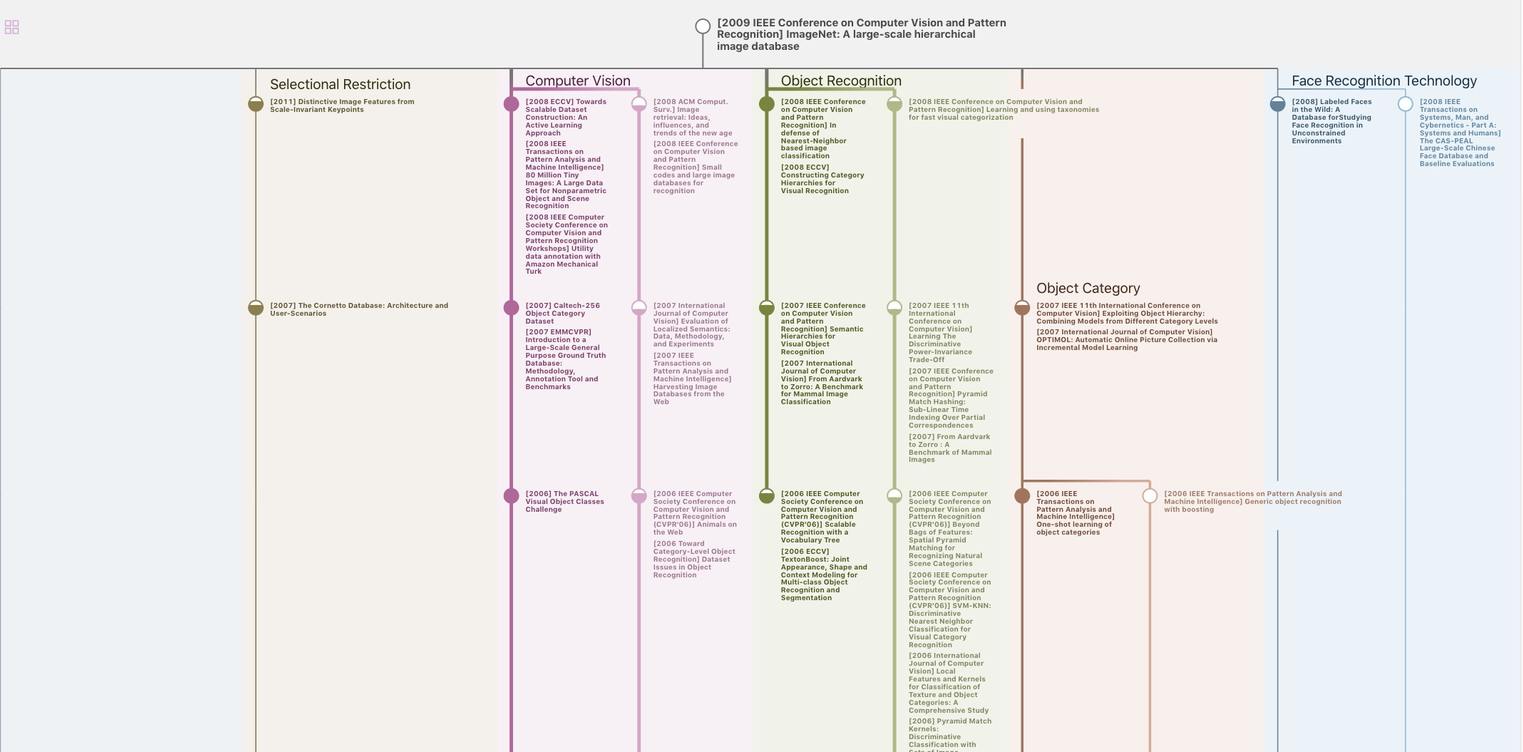
生成溯源树,研究论文发展脉络
Chat Paper
正在生成论文摘要