Comparison of BERT Implementations for Enhanced Cancer Symptoms Extraction from Electronic Health Records
2024 IEEE First International Conference on Artificial Intelligence for Medicine, Health and Care (AIMHC)(2024)
Abstract
Effective management of cancer symptoms is pivotal for optimal clinical outcomes. This research aims to harness the potential of Electronic Health Records (EHRs), particularly unstructured clinical notes, as a rich data source for cancer symptom information. Given the complexity of extracting information from EHRs, we investigate the performance of various Large Language Models (LLMs), such as (Bidirectional Encoder Representations from Transformers) BERT and its variants, for cancer symptom identification. Using a carefully curated dataset of 1112 clinical notes annotated by experts for 13 prevalent cancer symptoms, we present a comparative analysis of the performance of models including BERT-based, Span BERT, Bio BERT, Clinical BERT, and PubMed BERT. Our findings unequivocally show that Clinical BERT outperforms other models, especially in metrics like precision, recall, and F1-score. This dominance of Clinical BERT underscores its potential to revolutionize cancer symptom management through EHRs, hinting at a brighter future for oncological research and improved treatment decision-making.
MoreTranslated text
AI Read Science
Must-Reading Tree
Example
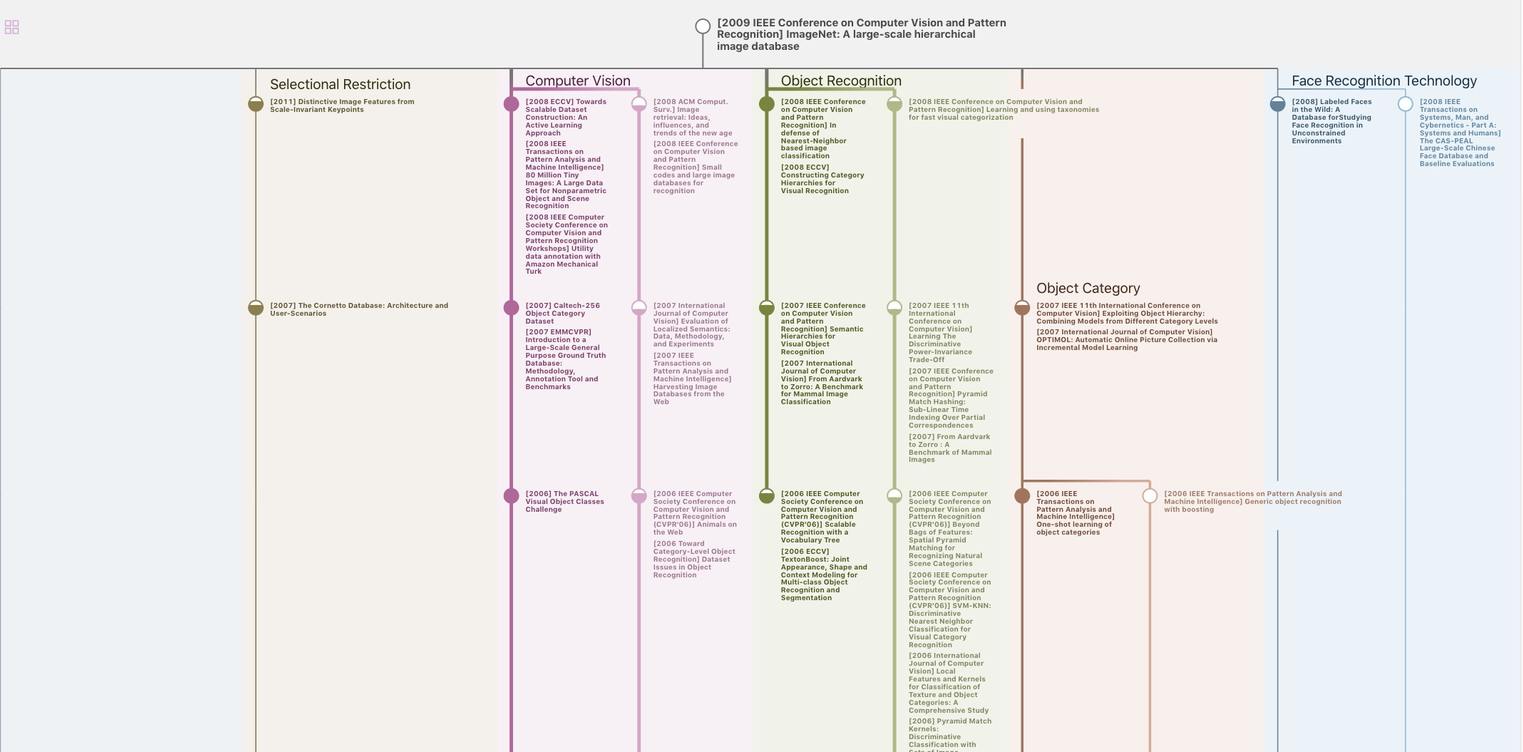
Generate MRT to find the research sequence of this paper
Chat Paper
Summary is being generated by the instructions you defined