Enhancing Data Accessibility in COVID Research - A Synthetic Data Generator for the RADx Data Hub
2024 IEEE First International Conference on Artificial Intelligence for Medicine, Health and Care (AIMHC)(2024)
摘要
The NIH RADx Data Hub serves as an access platform for COVID-19 research studies funded by the U.S. National Institutes of Health (NIH). The RADx Data Hub contains a range of patient-related information, including diagnostic test data, demographic details, and clinical records. Controlled access to data safeguards patient privacy, but also presents challenges for users unfamiliar with the platform, developers seeking to test processes, and curators aiming to showcase its features. Meanwhile, using actual patient data raises confidentiality concerns and timing challenges, such as needing to get requisite approval. To address these limitations, synthetic datasets support generation of simulated patients, enabling effective learning and testing of the system with data closely resembling real study data. This paper describes a dynamic synthetic data generator for COVID-19 research data based on RADx codebooks and external sources. The generator empowers RADx Data Hub users to model diverse patient cohorts, generate hypotheses, and attain a better grasp of existing COVID-19 data that they may access through the RADx Data Hub.
更多查看译文
关键词
Synthetic data,COVID-19 research,data access platform,privacy protection,controlled access,data generator,data modeling,patient data,clinical records
AI 理解论文
溯源树
样例
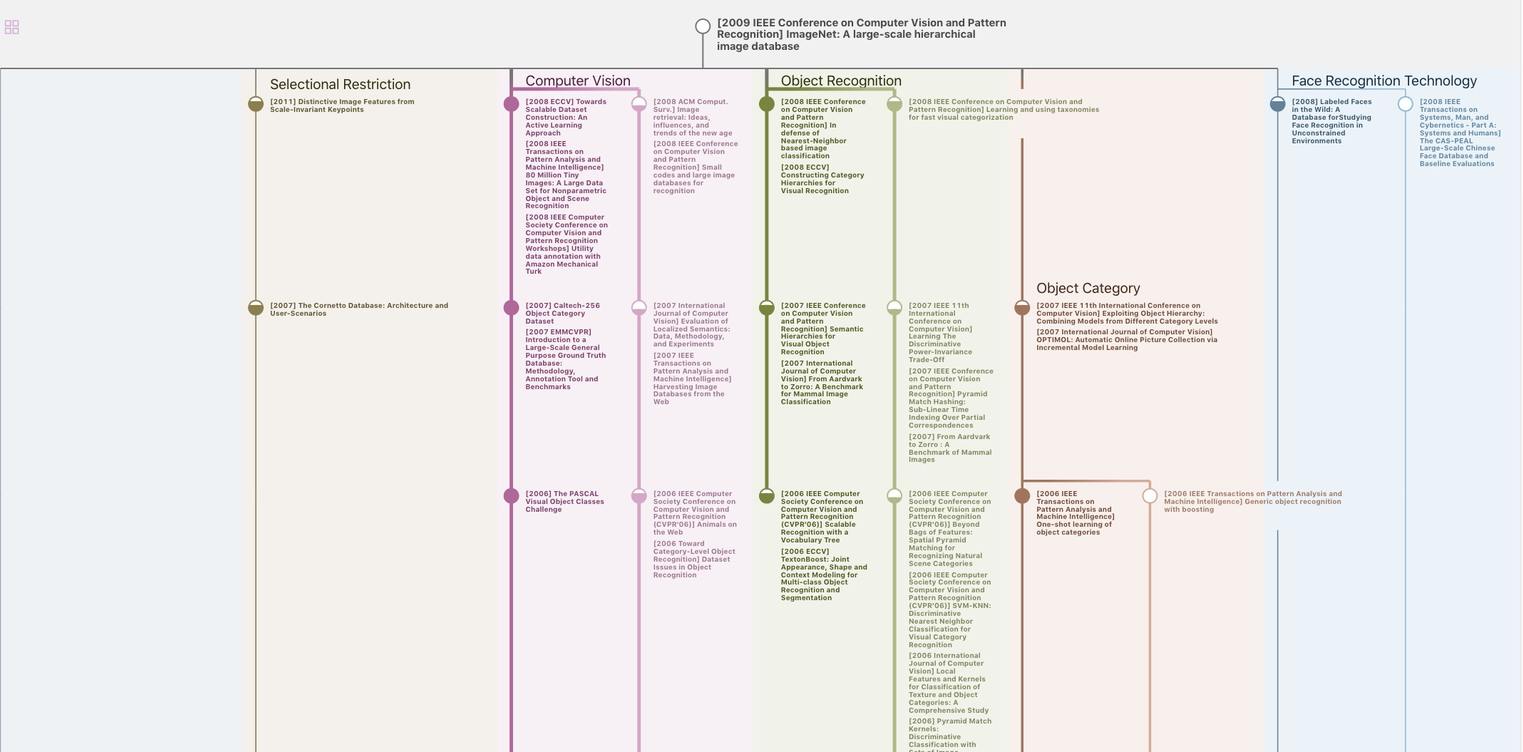
生成溯源树,研究论文发展脉络
Chat Paper
正在生成论文摘要