Performance Bound for Iterative Learning Control of Iteration-Varying Battery SoC Profiles Under Iteration-Invariant Learning Gains
2024 SICE International Symposium on Control Systems (SICE ISCS)(2024)
Abstract
This research studies the problem of learning and control for electric vehicle (EV) battery state-of-charge (SoC) profiles subject to their slight daily variations. These variations are due to many factors including traffic and weather conditions, driver behaviors, etc., hence pose a challenge on how to learn from such small changes on the SoC patterns to derive better control algorithms for the EV batteries. To cope with such challenge, iterative learning control (ILC) framework is employed in the current study, and iteration-invariant learning gains are first used to verify the performance limit induced by such gains. Accordingly, an explicit and analytical upper bound is derived for the ILC tracking error, which shows the effects of the ILC learning gain, the SoC profile variations, and the initial ILC setup.
MoreTranslated text
AI Read Science
Must-Reading Tree
Example
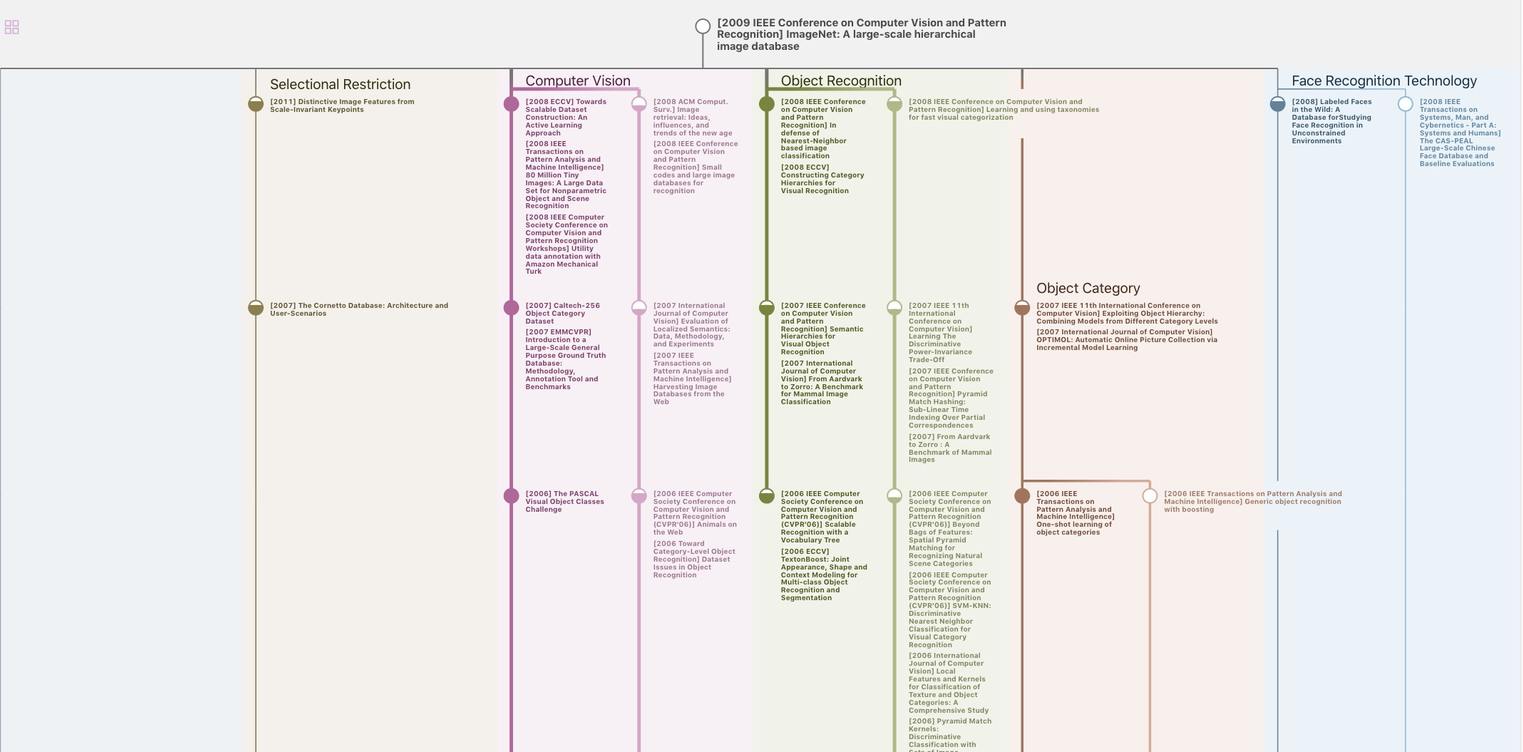
Generate MRT to find the research sequence of this paper
Chat Paper
Summary is being generated by the instructions you defined