MDFL: Model-Distance Federated Learning on Non-IID data.
International Conference on Communication and Information Processing(2023)
Abstract
Federated learning (FL) is an emerging approach in the field of Artificial Intelligence of Things (AIoT) that enables collaborative model training across multiple edge devices or IoT nodes while protecting data privacy. However, FL faces significant challenges, particularly regarding the non-Independent and Identically Distributed (non-IID) data across IoT devices. Despite the considerable efforts dedicated to address this issue, existing approaches seriously impact on the speed of convergence in FL. In this paper, we propose MDFL, a novel FL framework that incorporates a distance-based participant selection strategy to enhance the overall performance and efficiency. MDFL eliminates the consistency among clients regarding the epochs of local training and the weighted coefficient of server aggregation. Specifically, our method allows strong participants to achieve more training epochs and gain a larger weighting proportion during the aggregation. We evaluate the effectiveness of our approach on the MNIST and FashionMNIST datasets. Experimental results demonstrate that MDFL achieves superior performance, improving the test accuracy from 0.9% to 1.8% on MNIST and from 0.6% to 1.0% on FashionMNIST, respectively.
MoreTranslated text
AI Read Science
Must-Reading Tree
Example
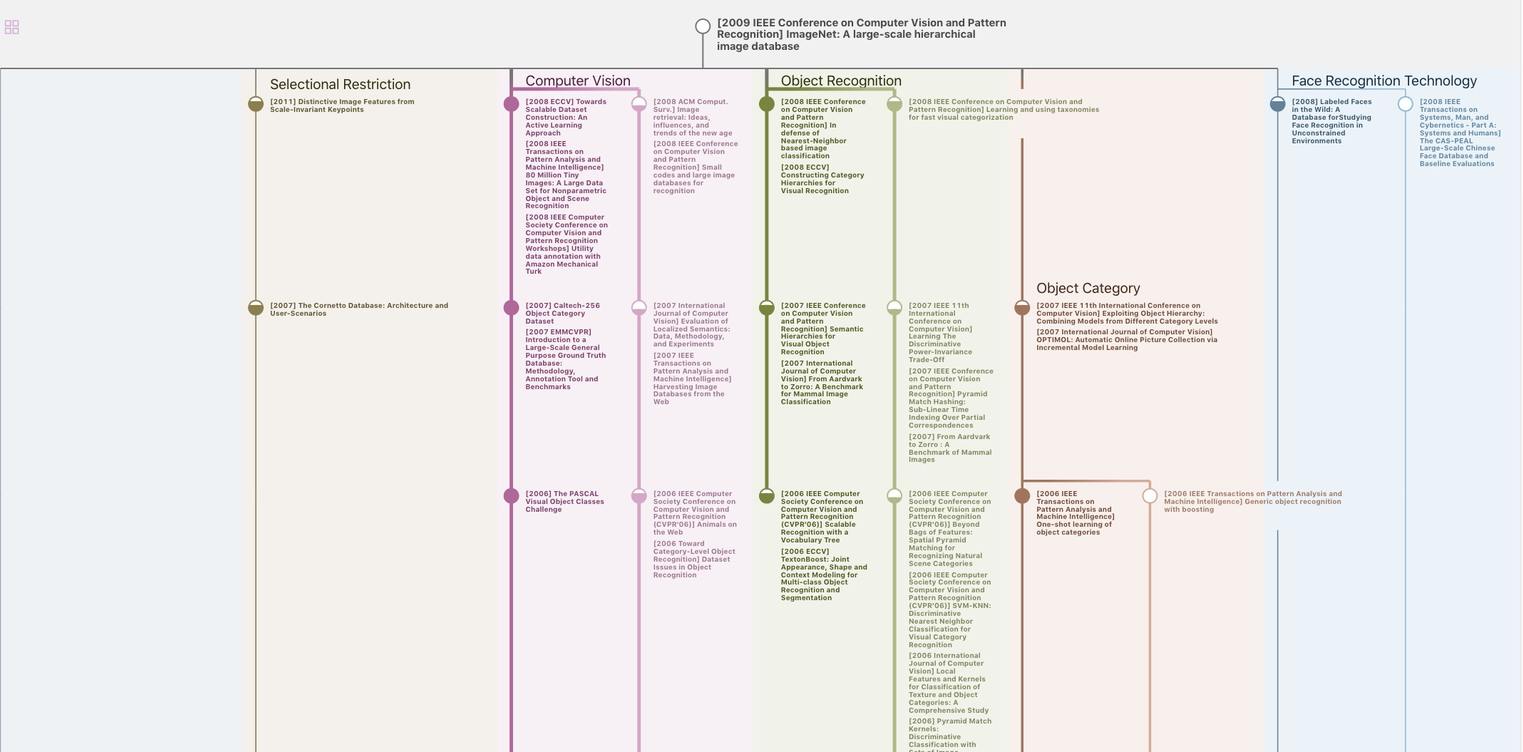
Generate MRT to find the research sequence of this paper
Chat Paper
Summary is being generated by the instructions you defined