GaitParse: Gait Parsing Algorithm with Self-Supervised Fine-Tuning for Gait Recognition.
International Conference on Communication and Information Processing(2023)
Abstract
Gait recognition, which uses individuals’ walking patterns for identity recognition, is an emerging biometric technology. However, in real-world applications, occlusion factors can easily affect the performance of gait recognition. In recent literatures, it has been demonstrated that the employment of human body part features has been shown to be beneficial for gait recognition. In this paper, we propose a novel method GaitParse to mitigate the effect of occlusion factors on gait recognition performance, which uses our designed gait parsing algorithm to support gait recognition by parsing human gait images into distinct body parts. Our algorithm consists of two main stages: The first stage is to apply the self-correcting human parsing model SC-ACE2P as a module, pre-trained on the Pascal dataset, to obtain a gait parser capable of parsing human body parts in RGB images. The second stage is self-supervised fine-tuning of the parser to improve accuracy and robustness for binary images. To conclude, our method focuses more on local features and provides richer feature representations, which enhances gait recognition performance and reduces recognition errors caused by occlusion factors. Experiments on the widely used public dataset, CASIA-B, richly demonstrate the superiority of our method over other traditional methods.
MoreTranslated text
AI Read Science
Must-Reading Tree
Example
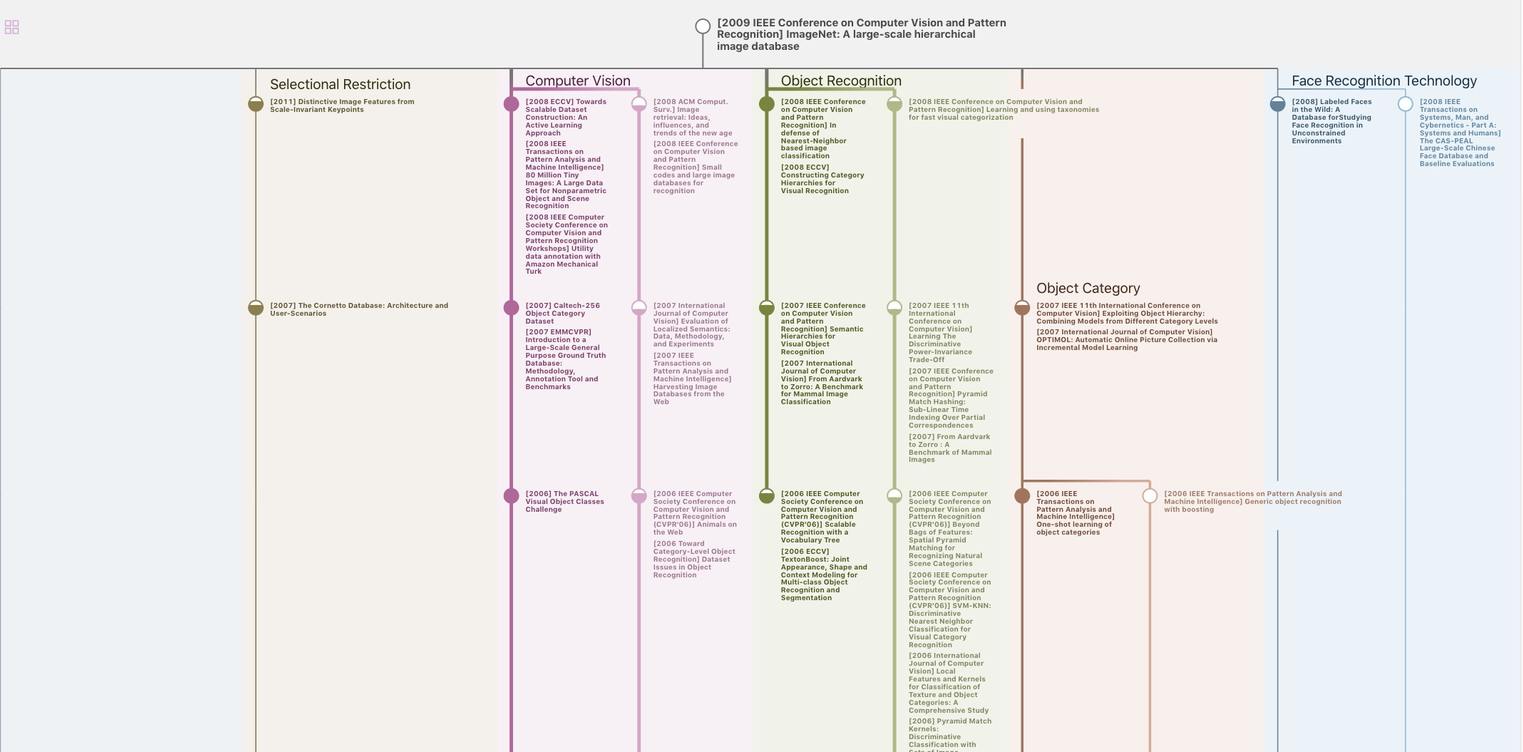
Generate MRT to find the research sequence of this paper
Chat Paper
Summary is being generated by the instructions you defined