Complexity-aware Features Selection for Wrist-worn Human Activity Recognition.
Annual IEEE International Conference on Pervasive Computing and Communications(2024)
摘要
Wrist-worn wearable devices have become increasingly prevalent in monitoring human activities, with applications ranging from healthcare to fitness tracking. Accurate human activity recognition from wrist-worn sensor data is essential for enabling these applications. However, the high-dimensional nature of sensor data poses challenges in terms of computational complexity and model interpretability. Feature selection is a critical step in mitigating these challenges by identifying a subset of relevant features while preserving the discriminative information. In this study, we propose a complexity-aware feature selection method tailored for wearable systems. Our approach leverages the intrinsic characteristics of the data to prioritize and select the most informative features while simultaneously weighing their computational efficiency by means of an ad-hoc metric that quantifies the number of significant CPU instructions associated with each feature. The results on publicly available datasets demonstrate that the proposed method effectively balances computational complexity and recognition accuracy.
更多查看译文
关键词
Human Activity Recognition,Feature Selection,Constrained Devices
AI 理解论文
溯源树
样例
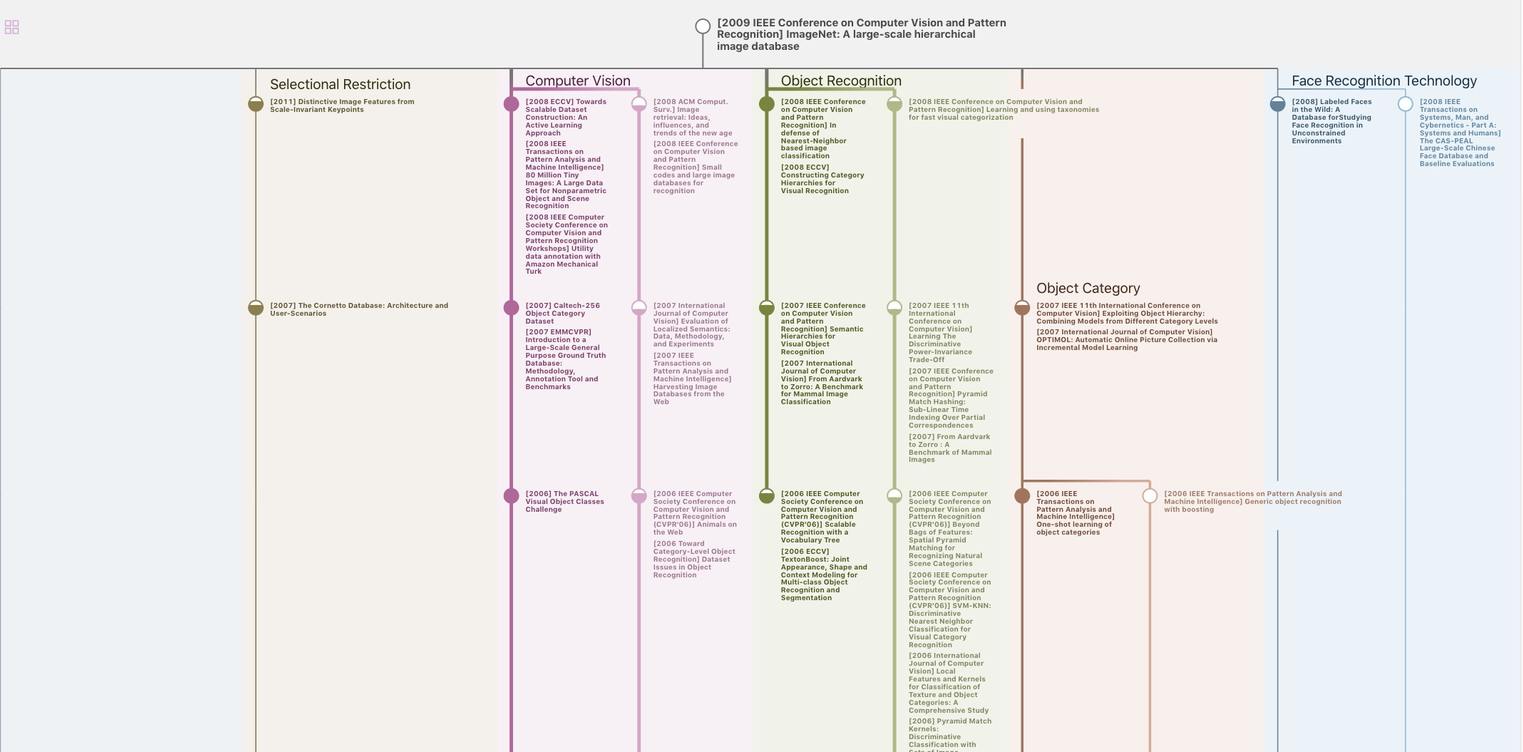
生成溯源树,研究论文发展脉络
Chat Paper
正在生成论文摘要