VCE-AnomalyNet: A New Dataset Fueling AI Precision in Anomaly Detection for Video Capsule Endoscopy ⋆
crossref(2024)
摘要
Video capsule endoscopy (VCE) is a minimally invasive diagnostic technique that helps in the detection of various anomalies like polyps, ulcers, aphthae, etc, within the intestinal lumen. Due to the high no. of frames in VCE and the low doctor-to-patient ratio across the globe, the inspection time of VCE is about 2-4 hours. Research has shown that Artificial Intelligence (AI) has the potential to decrease the inspection time in VCE reading and improve upon the false-positive rates. However, the lack of AI data is a big hindrance to it. To address this issue, we present the VCE-AnomalyNet Dataset, a new AI dataset fueling AI precision in anomaly detection for VCE. The dataset comprises 108,832 accurately labeled frames with bounding box annotations in YOLO (You Only Look Once) format. These frames have been compiled from multiple open-source datasets, aiming to support research in automatic anomaly detection in VCE. The dataset is available at VCE-AnomalyNet Dataset (zenodo.org) .
更多查看译文
AI 理解论文
溯源树
样例
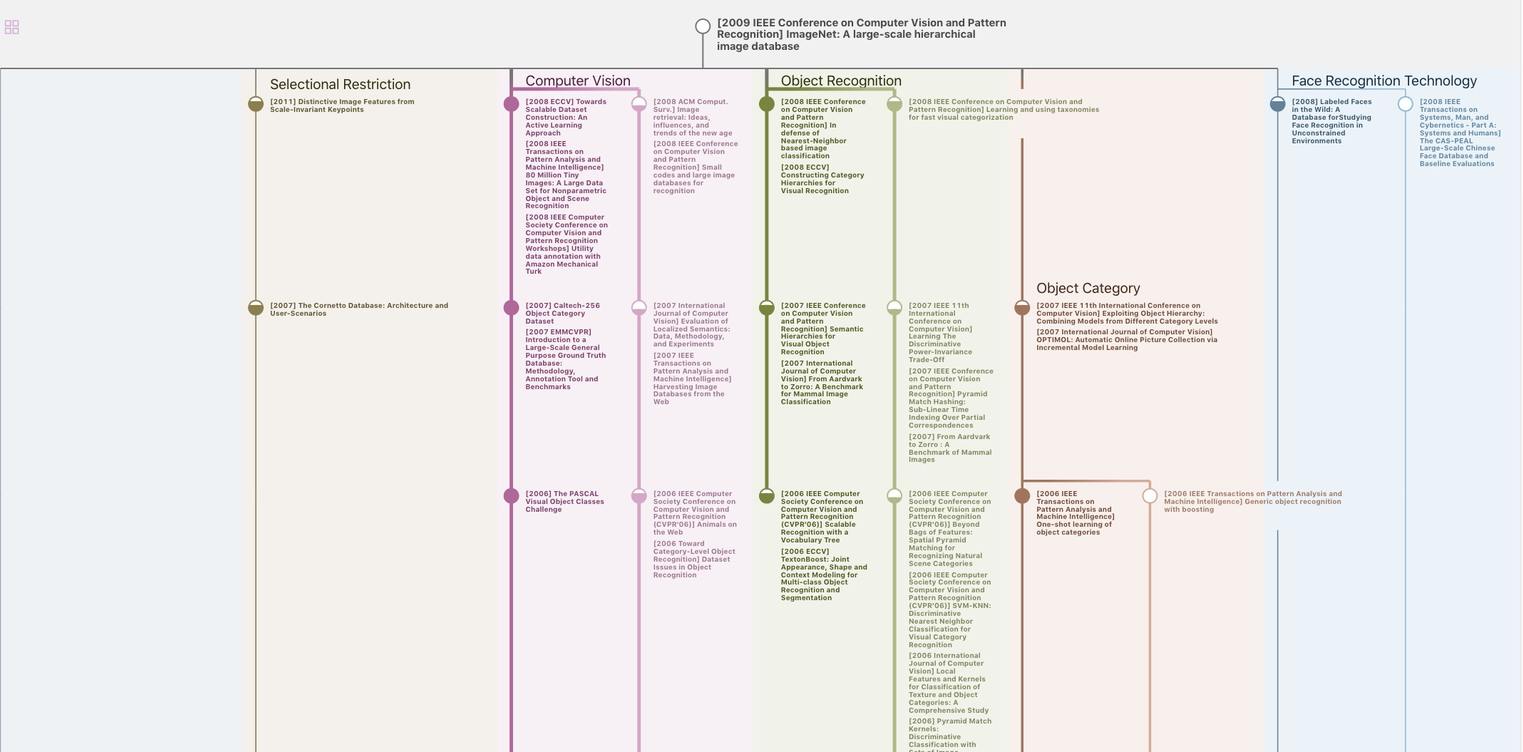
生成溯源树,研究论文发展脉络
Chat Paper
正在生成论文摘要