Superpixel Segmentation Based Evolutionary Multitasking Algorithm for Feature Selection of Hyperspectral Images
IEEE Transactions on Evolutionary Computation(2024)
摘要
Feature selection (FS) is a very important technique for hyperspectral image (HSI) classification, as successfully selecting informative features can significantly increase the learning performance while reducing the computational cost. However, most of the existing FS methods tend to treat the HSI as a whole for FS, which does not fully consider the unique characteristics of HSIs and disregards the fact that different feature classes possess varying preferences for features. Thus, this paper proposes a superpixel segmentation based evolutionary multitasking algorithm for FS of HSIs, called SS-EMT. First, the superpixel segmentation method is used to partition the original HSI into several superpixel blocks, which can preserve well the information of different classes of the original image. Second, in order to explore each superpixel block efficiently, an evolutionary multitasking algorithm using particle swarm optimization is designed, which treats each superpixel block as a subtask and then optimizes these subtasks collaboratively by transferring useful knowledge among related subtasks. In addition, a new individual evaluation mechanism is devised to obtain multiple high-quality feature subsets with different numbers of features simultaneously in a single run, thus reducing the computational cost. Finally, extensive experimental results on four common HSI datasets under three classifiers validate that our proposed method outperforms several state-of-the-art FS methods.
更多查看译文
关键词
Feature selection,hyperspectral images,evolutionary multitasking,superpixel segmentation
AI 理解论文
溯源树
样例
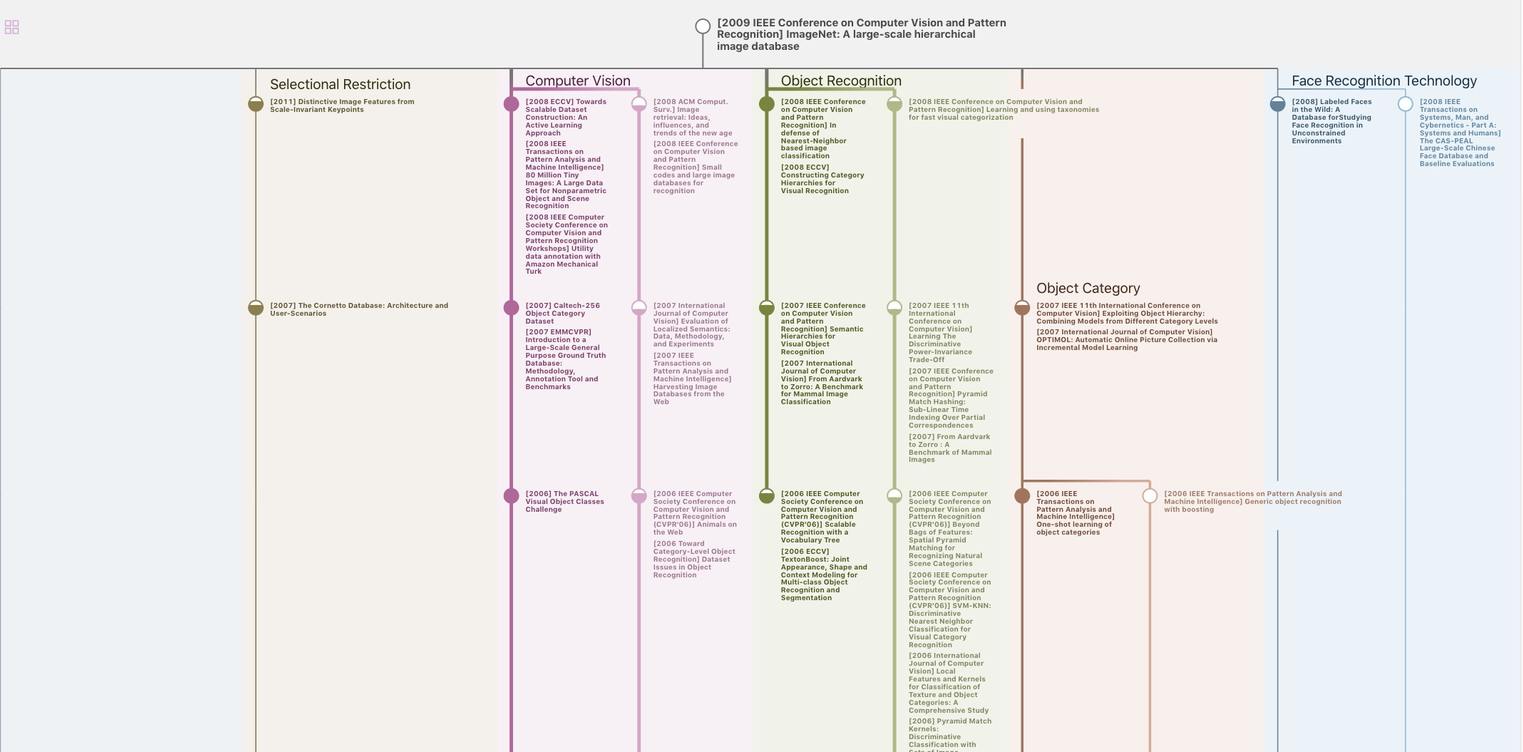
生成溯源树,研究论文发展脉络
Chat Paper
正在生成论文摘要