Novel Transfer Learning Approach for Driver Drowsiness Detection Using Eye Movement Behavior
IEEE ACCESS(2024)
摘要
Driver drowsiness detection is a critical field of research within automotive safety, aimed at identifying signs of fatigue in drivers to prevent accidents. Drowsiness impairs a driver's reaction time, decision-making ability, and overall alertness, significantly increasing the risk of collisions. Nowadays, the challenge is to detect drowsiness using physiological signals, which often require direct contact with the driver's body. This can be uncomfortable and distracting. This study aimed at detecting driver drowsiness through eye movement behavior imagery of the driver. We utilized a standard image dataset based on the eye movement behavior of drivers to conduct this research experiment. We proposed a novel transfer learning-based features generation which combined the strengths of the Visual Geometry Group (VGG-16) and Light Gradient-Boosting Machine (LGBM) methods. The proposed VGLG (VGG16-LGBM) approach first extracts spatial features from input eye image data and then generates salient transfer features using LGBM. Experimental evaluations reveal that the k-neighbors classifier outperformed the state-of-the-art approach with a high-performance accuracy of 99%. The computational complexity analysis shows that the proposed approach detects driver drowsiness in 0.00829 seconds. We have enhanced the performance through hyperparameter tuning and validations using k-fold validation. This research has the potential to revolutionize driver drowsiness detection, aiming to prevent road accidents and save precious lives.
更多查看译文
关键词
Vehicles,Feature extraction,Transfer learning,Brain modeling,Accidents,Predictive models,Neural networks,Safety,Deep learning,Vehicle driving,Driver drowsiness,eye images,eye behavior,machine learning,deep learning,transfer leaning
AI 理解论文
溯源树
样例
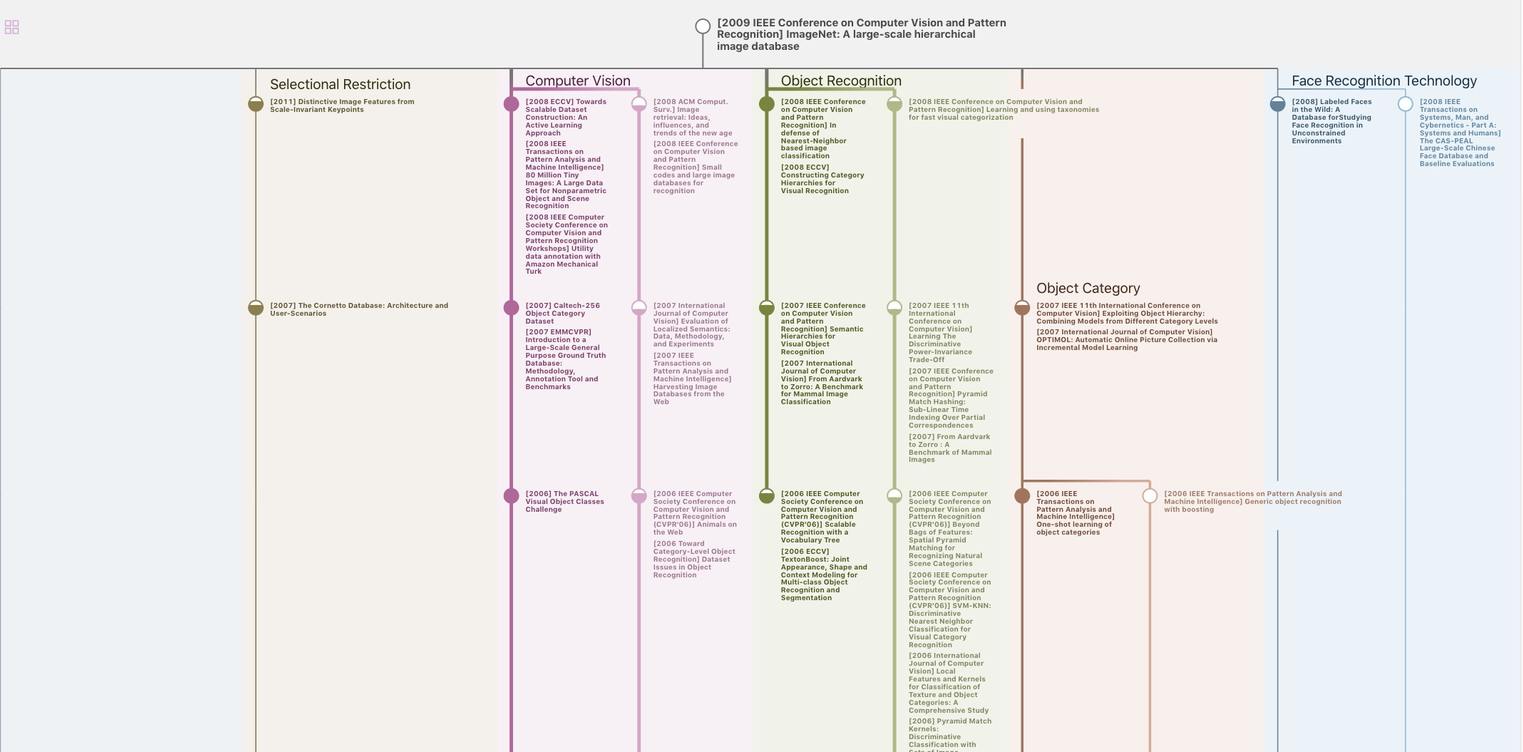
生成溯源树,研究论文发展脉络
Chat Paper
正在生成论文摘要